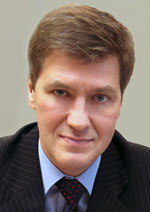
Nikiforov
Vladimir O.
D.Sc., Prof.
EXPERIMENTAL STUDY OF FIRMWARE FOR INPUT AND EXTRACTION OF USER’S VOICE SIGNAL IN VOICE AUTHENTICATION SYSTEMS
Read the full article

Abstract
T Scientific task for improving the signal-to-noise ratio for user’s voice signal in computer systems and networks during the process of user’s voice authentication is considered. The object of study is the process of input and extraction of the voice signal of authentication system user in computer systems and networks. Methods and means for input and extraction of the voice signal on the background of external interference signals are investigated. Ways for quality improving of the user’s voice signal in systems of voice authentication are investigated experimentally. Firmware means for experimental unit of input and extraction of the user’s voice signal against external interference influence are considered. As modern computer means, including mobile, have two-channel audio card, two microphones are used in the voice signal input. The distance between sonic-wave sensors is 20 mm and it provides forming one direction pattern lobe of microphone array in a desired area of voice signal registration (from 100 Hz to 8 kHz). According to the results of experimental studies, the usage of directional properties of the proposed microphone array and space-time processing of the recorded signals with implementation of constant and adaptive weighting factors has made it possible to reduce considerably the influence of interference signals. The results of firmware experimental studies for input and extraction of the user’s voice signal against external interference influence are shown. The proposed solutions will give the possibility to improve the value of the signal/noise ratio of the useful signals recorded up to 20 dB under the influence of external interference signals in the frequency range from 4 to 8 kHz. The results may be useful to specialists working in the field of voice recognition and speaker discrimination.
Acknowledgements. Работа выполнена при поддержке Министерства образования и науки Российской Федерации и Совета по грантам Президента Российской Федерации (грант МД-1072.2013.9) и частично при государственной финансовой поддержке ведущих университетов Российской Федерации (субсидия 074-U01).
References