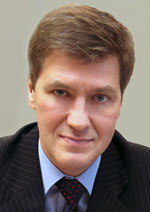
Nikiforov
Vladimir O.
D.Sc., Prof.
doi: 10.17586/2226-1494-2015-15-5-886-892
APPLICATION OF PARTIAL LEAST SQUARES REGRESSION FOR AUDIO-VISUAL SPEECH PROCESSING AND MODELING
Read the full article

For citation: Oleinik A.L. Application of Partial Least Squares regression for audio-visual speech processing and modeling. Scientific and Technical Journal of Information Technologies, Mechanics and Optics, 2015, vol. 15, no. 5, pp. 886–892
Abstract
Subject of Research. The paper deals with the problem of lip region image reconstruction from speech signal by means of Partial Least Squares regression. Such problems arise in connection with development of audio-visual speech processing methods. Audio-visual speech consists of acoustic and visual components (called modalities). Applications of audio-visual speech processing methods include joint modeling of voice and lips’ movement dynamics, synchronization of audio and video streams, emotion recognition, liveness detection. Method. Partial Least Squares regression was applied to solve the posed problem. This method extracts components of initial data with high covariance. These components are used to build regression model. Advantage of this approach lies in the possibility of achieving two goals: identification of latent interrelations between initial data components (e.g. speech signal and lip region image) and approximation of initial data component as a function of another one. Main Results. Experimental research on reconstruction of lip region images from speech signal was carried out on VidTIMIT audio-visual speech database. Results of the experiment showed that Partial Least Squares regression is capable of solving reconstruction problem. Practical Significance. Obtained findings give the possibility to assert that Partial Least Squares regression is successfully applicable for solution of vast variety of audio-visual speech processing problems: from synchronization of audio and video streams to liveness detection.
Acknowledgements. The work was done under government financial support for the leading universities of the Russian Federation (grant 074- U01). The author expresses his sincere appreciation to Professor Georgy Kukharev, his scientific adviser, and Yuri Matveev, Head of SIS Department, for their critical remarks and advice that significantly improved the paper.
References