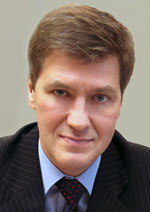
Nikiforov
Vladimir O.
D.Sc., Prof.
doi: 10.17586/2226-1494-2015-15-6-1130-1138
SMARTPHONE-BASED APPROACH TO ADVANCED DRIVER ASSISTANCE SYSTEM (ADAS) RESEARCH AND DEVELOPMENT
Read the full article

For citation: Lashkov I.B., Smirnov A.V., Kashevnik A.M. Smartphone-based approach to advanced driver assistance system (ADAS) research and development. Scientific and Technical Journal of Information Technologies, Mechanics and Optics, 2015, vol. 15, no. 6, pp. 1130–1138.
Abstract
Subject of Research.The paper deals with findings and presents asmartphone-based approach to advanced driver assistance system (ADAS) research and development.The approach is based on the data of smartphone cameras and sensors. The line of researchis associated with the developmentof mobile advanced driver assistance system (ADAS). Method.The proposedapproach isbased on the use of driver'sand vehicle behavior ontologies. Current ADAS systems can be divided into two main categories according to the method of implementation: mobile applications, manually installed by the driver from the application stores, and safetyhardware and softwaresystems,integrated into vehicles by manufacturesor in the automotive service centers.Mobile application installed on the smartphone uses the built-in rear and front-facing cameras and sensors to monitor both the road and vehicles ahead, and at the same time the driver in order to prevent traffic collisions. The service consists of components for objects recognition in the images obtained with cameras, and components for traffic situation analysis. Main Results. The driver safety mobile application has been developedfor the use on mobile phones.The mobile phone is mounted on the windshield of a car.In case of dangerous event occurrence, the application engine will make an audible or vibration signal to inform the driver to be concentratedand more vigilant. For example, road obstacles, rear-end and stationary vehicle accidents are the most common accident types.The mobile application detects whether a crash is imminent by computing the ‘Time To Contact’ (TTC) taking into account host vehicle speed, relative speed and relative acceleration.If the driver doesn’t maintain safe minimum distance with the car immediately ahead, the mobile application will alert the driver by displaying an attention icon with an audible alert. The dual-camera sensing application is designed to help the drivers increase the trip safety and assess and improve theirdriving skills. Practical Relevance.The proposed approach is designed to help the driver in the driving process, anticipate hazards and provide the driver with appropriate messages through his/her mobile phone.
Acknowledgements. The presented findings are part of the research carried out within the project funded by grants #13-07-00336, 13-07-12095, 13-01-00286, 14-07-00345 and 14-07-00363 of the Russian Foundation for Basic Research. This work is also carried out with the financial support of the Government of the Russian Federation, Grant 074-U01.
References
1. Janssen J., Krings D. Mit chip und smartphone – IPS und IPSI vernetzen handy-ticket-systeme in Deutschland. Der Nahverkehr, 2014, no. 1-2, pp. 7–9.
2. Smirnov A., Lashkov I. State-of-the-art analysis of available advanced driver assistance systems. Proc. 17th Conference of the Open Innovations Association FRUCT. Yaroslavl', Russia, 2015, pp. 345–349.
3. Hashimoto N., Tomita K., Boyli A., Matsumoto O., Smirnov A., Kashevnik A., Lashkov I. Operational evaluation of new transportation method for smart city: use of personal mobility vehicles under three different scenarios. Proc. 4th Int. Conf. on Smart Systems, Devices and Technologies. Brussels, Belgium, 2015, pp. 1–6.
4. Lashkov I.B., Smirnov A.V., Kashevnik A.M., Hashimoto N., Boyali A., Smirnov A.V. Smartphone-based on-the-fly two-wheeled self-balancing vehicles rider assistant. Proc. 17th Conference of Open Innovations Association FRUCT. Yaroslavl', Russia, 2015, pp. 201–209.
5. Whitlock A., Pethick J. Driver vigilance devices: systems review. Rail Safety and Standards Board's, T024 Quin 22 RPT Final Report, 2002, no. 1.
6. Dinges D.F., Grace R. PERCLOS: a valid psychophysiological measure of alertness as assessed by psychomotor vigilance. Technical Report FHWA-MCRT-98-006. Federal Highway Administration, Office of Motor Carrier Research and Standards, 1998.
7. Kiroi V., Shaposhnikov D., Anishchenko S. V osnovu sistem «tekhnicheskogo zreniya» dolzhny byt' polozheny zhivye neirotekhnologii [Systems of "machine vision" must be based on live nanotechnology]. Kommersant'-Nauka, 2015, no. 4, pp. 26–27.
8. Zakharchenko D.V. Izmenenie Parametrov Okulomotornykh i Dvigatel'nykh Reaktsii Operatora pod Deistviem Alkogolya: diss. …kand. biol. nauk [Changing the Oculomotor and Motor Reactions of the Operator under the Alcohol Influence. Dis. Biol. Sci.]. Moscow, 2015, 105 p.
9. Anon. Proximity array sensing system: head position monitor/metric. Technical Report NM 87504. Advanced Safety Concepts, Sante Fe, 1998.
10. Dinges D.F., Mallis M.M., Maislin G., Powell J.W. Evaluation of techniques for ocular measurement as an index of fatigue and the basis for alertness management. NHTSA Report DOT-HS-808762. Washington, 1998, 112 p.
11. Wierville W.W. Overview of research on driver drowsiness definition and driver drowsiness detection. Proc. 14th Int. Conf. on Enhanced Safety of Vehicles. Munich, 1994, pp. 462–468.
12. Portalova M.A. Influence of psychical, and also physiological features of personality of driver on reliability of management a transport vehicle. Society and Law, 2009, no. 5, pp. 311–313. (In Russian)
13. Gu Q., Yang J., Zhai Y., Kong L. Vision-based multi-scaled vehicle detection and distance relevant mix tracking for driver assistance system. Optical Review, 2015, vol. 22, no. 2, pp. 197–209. doi: 10.1007/s10043-015-0067-8
14. Gaikwad V., Lokhande S., Lane departure identification for advanced driver assistance. IEEE Transactions on Intelligent Transportation Systems, 2015, vol. 16, no. 2, pp. 910–918. doi: 10.1109/TITS.2014.2347400
15. Viola P., Jones M.J. Robust real-time face detection. International Journal of Computer Vision, 2004, vol. 57, no. 2, pp. 137–154. doi: 10.1023/B:VISI.0000013087.49260.fb
16. Freund Y., Schapire R. A short introduction to boosting. Journal of Japanese Society for Artificial Intelligence, 1999, vol. 14, no. 5, pp. 771–780.