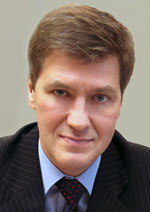
Nikiforov
Vladimir O.
D.Sc., Prof.
doi: 10.17586/2226-1494-2016-16-4-703-709
DEVELOPMENT OF AUTOMATED SPEECH RECOGNITION SYSTEM FOR EGYPTIAN ARABIC PHONE CONVERSATIONS
Read the full article

For citation: Romanenko A.N. Development of automated speech recognition system for Egyptian Arabic phone conversations. Scientific and Technical Journal of Information Technologies, Mechanics and Optics, 2016, vol. 16, no. 4, pp. 703–709. doi: 10.17586/2226-1494-2016-16-4-703-709
Abstract
The paper deals with description of several speech recognition systems for the Egyptian Colloquial Arabic. The research is based on the CALLHOME Egyptian corpus. The description of both systems, classic: based on Hidden Markov and Gaussian Mixture Models, and state-of-the-art: deep neural network acoustic models is given. We have demonstrated the contribution from the usage of speaker-dependent bottleneck features; for their extraction three extractors based on neural networks were trained. For their training three datasets in several languageswere used:Russian, English and differentArabic dialects.We have studied the possibility of application of a small Modern Standard Arabic (MSA) corpus to derive phonetic transcriptions. The experiments have shown that application of the extractor obtained on the basis of the Russian dataset enables to increase significantly the quality of the Arabic speech recognition. We have also stated that the usage of phonetic transcriptions based on modern standard Arabic decreases recognition quality. Nevertheless, system operation results remain applicable in practice. In addition, we have carried out the study of obtained models application for the keywords searching problem solution. The systems obtained demonstrate good results as compared to those published before. Some ways to improve speech recognition are offered.
References
1. Kirchhoff K., Bilmes J., Das S., Duta N., Egan M., Ji G., He F., Henderson J., Liu D., Noamany M., Schone P., Schwartz R., Vergyri D. Novel approaches to Arabic speech recognition: report from the 2002 Johns-Hopkins Summer Workshop. Proc. IEEE Int. Conf. on Acoustics, Speech and Signal Processing, ICASSP. Hong Kong, 2003, vol. 1, pp. 344–347.
2. Human Development Report 2006. Beyond Scarcity: Power, Poverty and Global Water Crisis. Palgrave Macmillan, UK, 2006, pp. 297–300.
3. Habash N., Eskander R., Hawwari A. A morphological analyzer for egyptian Arabic. NAACL-HLT 2012 Workshop on Computational Morphology and Phonology, SIGMOR-PHON2012. 2012, pp. 1–9.
4. Elmahdy M., Hasegawa-Johnson M., Mustafawi E., Duwairi R., Minker W. Challenges and techniques for dialectal arabic speech recognition and machine translation. Proc. Qatar Foundation Annual Research Forum. Doha, 2011.
5. Elmahdy M., Hasegawa-Johnson M., Mustafawi E. Hybrid phonemic and graphemic modeling for arabic speech recognition. International Journal of Computational Linguistics, 2012, vol. 3, no. 1, pp. 88–96.
6. Ali A., Mubarak H., Vogel S. Advances in dialectal arabic speech recognition: a study using twitter to improve Egyptian ASR. Proc. Int. Workshop on Spoken Language Translation, IWSLT 2014. South Lake Tahoe, USA, 2014, pp. 156–162.
7. El-Desoky Mousa A., Kuo H.-K.J., Mangu L., Soltau H. Morpheme-based feature-rich language models using Deep Neural Networks for LVCSR of Egyptian Arabic. Proc. 38th IEEE Int. Conf. on Acoustics Speech and Signal Processing, ICASSP. Vancouver, Canada, 2013, pp. 8435–8439. doi: 10.1109/ICASSP.2013.6639311
8. Ali A., Zhang Y., Cardinal P., Dahak N., Vogel S., Glass J. A complete KALDI recipe for building Arabic speech recognition systems. Proc. IEEE Workshop on Spoken Language Technology. South Lake Tahoe, USA, 2014, pp. 525–529. doi: 10.1109/SLT.2014.7078629
9. Thomas S.W., Saon G., Kuo H.-K., Mangu L. The IBM BOLT speech transcription system. Proc. 6th Annual Conference of the International Speech Communication Association. Dresden, Germany, 2015, pp. 3150–3153.
10. Trmal J., Chen G., Povey D., Khudanpur S. et. al. A keyword search system using open source software. Proc. IEEE Workshop on Spoken Language Technology. South Lake Tahoe, USA, 2014, pp. 530–535.
11. Povey D., Ghoshal A. et al. The Kaldi speech recognition toolkit. Proc. IEEE Workshop on Automatic Speech Recognition and Understanding, ASRU. Waikoloa, Hawaii, USA, 2011.
12. Liu F., Stern R., Huang X., Acero A. Efficient cepstral normalization for robust speech recognition. Proc. ARPA Workshop on Human Language Technology. Princeton, 1993, pp. 69–74. doi: 10.3115/1075671.1075688
13. Senoussaoui M., Kenny P., Dehak N., Dumouchel P. An i-vector extractor suitable for speaker recognition with both microphone and telephone speech. Odyssey 2010. The Speaker and Language Recognition Workshop. Brno, Czech Republic, 2010, pp. 28–33.
14. Gehring J., Miao Y., Metze F., Waibel A. Extracting deep bottleneck features using stacked auto-encoders. Proc. 38th IEEE Int. Conf. on Acoustics Speech and Signal Processing, ICASSP. Vancouver, Canada, 2013, pp. 3377–3381. doi: 10.1109/ICASSP.2013.6638284
15. Xin L., Hamaker J., He X. Robust feature space adaptation for telephony speech recognition. Proc. 9th Int. Conf. on Spoken Language Processing. Pittsburgh, USA, 2006, pp. 773–776.
16. Vesely K., Ghoshal A., Burget L., Povey D. Sequence-discriminative training of deep neural networks. Proc. 14th Annual Conf. of the International Speech Communication. Lyon, France, 2013, pp. 2345–2349.