Menu
Publications
2025
2024
2023
2022
2021
2020
2019
2018
2017
2016
2015
2014
2013
2012
2011
2010
2009
2008
2007
2006
2005
2004
2003
2002
2001
Editor-in-Chief
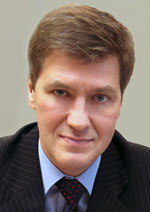
Nikiforov
Vladimir O.
D.Sc., Prof.
Partners
doi: 10.17586/2226-1494-2023-23-3-595-607
Intelligent clinical decision support for small patient datasets
Read the full article

Article in Russian
For citation:
Abstract
For citation:
Vatian A.S., Golubev A.A., Gusarova N.F., Dobrenko N.V., Zubanenko A.A., Kustova E.S., Tatarinova A.A., Tomilov I.V., Shovkoplyas G.F. Intelligent clinical decision support for small patient datasets. Scientific and Technical Journal of Information Technologies, Mechanics and Optics, 2023, vol. 23, no. 3, pp. 595–607 (in Russian). doi: 10.17586/2226-1494-2023-23-3-595-607
Abstract
The ways of substantiating the clinical decision of doctors in the absence of clinical treatment protocols are considered. A comparative evaluation of various statistical methods for ranking clinical symptoms in terms of significance for predicting the outcome of the disease in a small sample of patients with COVID-19 and a history of cardiovascular diseases was performed. The data set (141 patients, 81 factors) was formed based on the materials of electronic medical records of patients of the Federal State Budgetary Institution “National Medical Research Center named after V.A. Almazov”. A subset of controllable risk factors (51 factors) was identified. Descriptive statistics methods (one-way ANOVA, Mann-Whitney and χ² tests) and dimensionality reduction methods (univariate linear regression combined with multiple logistic regression, generalized discriminant analysis, and various decision tree algorithms) were used to rank the factors. To compare the ranking results and evaluate the statistical stability, Kendall’s correlation was used, visualized as a heat map and a positional graph. It has been established that the use of descriptive statistics methods is justified when ranking on a small sample size of patients. It is shown that the ensemble of ranking results may be statistically inconsistent. It is concluded that the positions of the same features obtained by ranking them as part of a complete set and a subset of features do not match; therefore, when choosing a statistical processing method for expert evaluation, one should take into account the meaningful formulation of the problem. It is shown that the statistical stability of ranking under conditions of small samples depends on the number of features taken into account, and this dependence is significantly different for different ranking methods. The proposed method of intellectual support and verification of clinical decisions in terms of choosing the most significant clinical signs can be used to select and justify the tactics of managing patients in the absence of clinical protocols.
Keywords: clinical decision support, clinical expertise, feature ranking, small cohorts, statistical methods
Acknowledgements. The work was supported by the grant of the President of the Russian Federation for state support of young Russian scientists — candidates of sciences MK-5723.2021.1.6
References
Acknowledgements. The work was supported by the grant of the President of the Russian Federation for state support of young Russian scientists — candidates of sciences MK-5723.2021.1.6
References
-
Adu-Amankwaah J., Mprah R., Adekunle A.O., Noah M.L.N., Adzika G.K., Machuki J.O., Sun H. The cardiovascular aspect of COVID-19. Annals of Medicine, 2021, vol. 53, no. 1, pp. 227–236. https://doi.org/10.1080/07853890.2020.1861644
-
Madjid M., Safavi-Naeini P., Solomon S.D., Vardeny O. Potential effects of coronaviruses on the cardiovascular system: a review. JAMA Cardiology, 2020, vol. 5, no. 7, pp. 831–840. https://doi.org/10.1001/jamacardio.2020.1286
-
Rumyantsev P.O., Saenko U.V., Rumyantseva U.V. Statistical methods for the analyses in clinical practice. Part 1. Univariate statistical analysis. Problems of Endocrinology, 2009, vol. 55, no. 5, pp. 48–55. (in Russian). https://doi.org/10.14341/probl200955548-55
-
Remeseiro B., Bolon-Canedo V. A review of feature selection methods in medical applications. Computers in Biology and Medicine, 2019, vol. 112, pp. 103375. https://doi.org/10.1016/j.compbiomed.2019.103375
-
Soares I., Dias J., Rocha H., do Carmo Lopes M., Ferreira B. Feature selection in small databases: a medical-case study. IFMBE Proceedings, 2016, vol. 57, pp. 814–819. https://doi.org/10.1007/978-3-319-32703-7_158
-
Nezhad M.Z., Zhu D., Li X., Yang K., Levy Ph. SAFS: A deep feature selection approach for precision medicine. Proc. of the 2016 IEEE International Conference on Bioinformatics and Biomedicine (BIBM), 2016, pp. 501–506. https://doi.org/10.1109/bibm.2016.7822569
-
Alelyani S. Stable bagging feature selection on medical data. Journal of Big Data, 2021, vol. 8, no. 11, pp. 11. https://doi.org/10.1186/s40537-020-00385-8
-
Wu L., Hu Y., Liu X., Zhang X., Chen W., Yu A.S.L., Kellum J.A., Waitman L.R., Liu M. Feature ranking in predictive models for hospital-acquired acute kidney injury. Scientific Reports, 2018, vol. 8, pp. 17298. https://doi.org/10.1038/s41598-018-35487-0
-
Golugula A., Lee G., Madabhushi A. Evaluating feature selection strategies for high dimensional, small sample size datasets. Proc. of the 33rd Annual International Conference of the IEEE Engineering in Medicine and Biology Society, 2011, pp. 949–952. https://doi.org/10.1109/iembs.2011.6090214
-
Gao L., Wu W. Relevance assignation feature selection method based on mutual information for machine learning. Knowledge-Based Systems, 2020, vol. 209, pp. 106439 https://doi.org/10.1016/j.knosys.2020.106439
-
Wang B., Li R., Lu Z., Huang Y. Does comorbidity increase the risk of patients with covid-19: Evidence from meta-analysis. Aging, 2020, vol. 12, no. 7, pp. 6049–6057. https://doi.org/10.18632/aging.103000
-
Amin M.S., Chiam Y., Varathan K.D. Identification of significant features and data mining techniques in predicting heart disease. Telematics and Informatics, 2019, vol. 36, pp. 82–93. https://doi.org/10.1016/j.tele.2018.11.007
-
Joloudari J.H., Joloudari E.H., Saadatfar H., Ghasemigol M., Razavi S.M., Mosavi A., Nabipour N., Shamshirband S., Nadai L. Coronary artery disease diagnosis; ranking the significant features using a random trees model. International Journal of Environmental Research and Public Health, 2020, vol. 17, no. 3, pp. 731. https://doi.org/10.3390/ijerph17030731
-
Pasha S.J., Mohamed E.S. Novel feature reduction (NFR) model with machine learning and data mining algorithms for effective disease risk prediction. IEEE Access, 2020, vol. 8, pp. 184087–184108. https://doi.org/10.1109/ACCESS.2020.3028714
-
Alam Z., Rahman S., Rahman S. A Random Forest based predictor for medical data classification using feature ranking. Informatics in Medicine Unlocked, 2019, vol. 15, pp. 100180. https://doi.org/10.1016/j.imu.2019.100180
-
Saqlain S.M., Sher M., Shah F.A., Khan I., Ashraf M.U., Awais M., Ghani A. Fisher score and Matthews correlation coefficient-based feature subset selection for heart disease diagnosis using support vector machines. Knowledge and Information Systems, 2019, vol. 58, no. 1, pp. 139–167. https://doi.org/10.1007/s10115-018-1185-y
-
Shah S.S.M., Batool S.S., Khan I., Muhammad Ashraf U., Abbas S.H., Hussain S.A. Feature extraction through parallel Probabilistic Principal Component Analysis for heart disease diagnosis. Physica A: Statistical Mechanics and its Applications, 2017, vol. 482, pp. 796–807. https://doi.org/10.1016/j.physa.2017.04.113
-
Abdollahi J., Nouri-Moghaddam B. Feature selection for medical diagnosis: Evaluation for using a hybrid Stacked-Genetic approach in the diagnosis of heart disease. arXiv, 2021, arXiv:2103.08175. https://doi.org/10.48550/arXiv.2103.08175
-
Velusamy D., Ramasamy K. Ensemble of heterogeneous classifiers for diagnosis and prediction of coronary artery disease with reduced feature subset. Computer Methods and Programs in Biomedicine, 2021, vol. 198, pp. 105770. https://doi.org/10.1016/j.cmpb.2020.105770
-
Ghosh P., Azam S., Jonkman M., Karim A., Shamrat F.M.J., Ignatious E., Shultana S., Beeravolu A.R., De Boer F. Efficient prediction of cardiovascular disease using machine learning algorithms with relief and LASSO feature selection techniques. IEEE Access, 2021, vol. 9, pp. 19304–19326. https://doi.org/10.1109/ACCESS.2021.3053759
-
Zhou F., Yu T., Du R., Fan G., Liu Y., Liu Z., Xiang J., Wang Y., Song B., Gu X., Guan L., Wei Y., Li H., Wu X., Xu J., Tu S., Zhang Y., Chen H., Cao B. Clinical course and risk factors for mortality of adult inpatients with COVID-19 in Wuhan, China: a retrospective cohort study. Lancet, 2020, vol. 395, pp. 1054–1062. https://doi.org/10.1016/S0140-6736(20)30566-3
-
Ruan Q., Yang K., Wang W., Jiang L., Song J. Clinical predictors of mortality due to COVID-19 based on an analysis of data of 150 patients from Wuhan, China. Intensive Care Medicine, 2020, vol. 46, no. 5, pp. 846–848. https://doi.org/10.1007/s00134-020-05991-x
-
Li X., Xu S., Yu M., Wang K., Tao Y., Zhou Y., Shi J., Zhou M., Wu B., Yang Z., Zhang C., Yue J., Zhang Z., Renz H., Liu X., Xie J., Xie M., Zhao J. Risk factors for severity and mortality in adult COVID-19 inpatients in Wuhan. Journal of Allergy and Clinical Immunology, 2020, vol. 146, no. 1, pp. 110–118. https://doi.org/10.1016/j.jaci.2020.04.006
-
Liu X., Xue S., Xu J., Ge H., Mao Q., Xu X., Jiang H. Clinical characteristics and related risk factors of disease severity in 101 COVID-19 patients hospitalized in Wuhan, China. Acta Pharmacologica Sinica, 2022, vol. 43, no. 1, pp. 64–75. https://doi.org/10.1038/s41401-021-00627-2
-
Alshaikh M.K., Alotair H., Alnajjar F., Sharaf H., Alhafi B., Alashgar L., Aljuaid M. Cardiovascular risk factors among patients infected with COVID-19 in Saudi Arabia. Vascular Health and Risk Management, 2021, vol. 17, pp. 161–168. https://doi.org/10.2147/vhrm.s300635
-
Phelps M., Christensen D.M., Gerds T., Fosbøl E., Torp-Pedersen Ch., Schou M., Køber L., Kragholm K., Andersson Ch., Biering-Sørensen T., Christensen H.C., Andersen M.P., Gislason G. Cardiovascular comorbidities as predictors for severe COVID-19 infection or death. European Heart Journal - Quality of Care and Clinical Outcomes, 2021, vol. 7, no. 2, pp. 172–180. https://doi.org/10.1093/ehjqcco/qcaa081
-
Kovvuri V.R.R., Liu S., Seisenberger M., Fan X., Muller B., Fu H. On understanding the influence of controllable factors with a feature attribution algorithm: a medical case study. Proc. of the 2022 International Conference on Innovations in Intelligent SysTems and Applications (INISTA), 2022, pp. 1–8. https://doi.org/10.1109/inista55318.2022.9894147
-
Lundberg S.M., Lee S.I. A unified approach to interpreting model predictions. NIPS'17: Proc. of the 31st International Conference on Neural Information Processing Systems, 2017, pp. 4768–4777.
-
Bhadra T., Mallik S., Hasan N., Zhao Z. Comparison of five supervised feature selection algorithms leading to top features and gene signatures from multi-omics data in cancer. BMC Bioinformatics, 2022, vol. 23, no. 3S, pp. 153. https://doi.org/10.1186/s12859-022-04678-y
-
Barraza N., Moro S., Ferreyra M., de la Peña A. Mutual information and sensitivity analysis for feature selection in customer targeting: A comparative study. Journal of Information Science, 2019, vol. 45, no. 1, pp. 53–67. https://doi.org/10.1177/0165551518770967
-
Bouchlaghem Y., Akhiat Y., Amjad S. Feature selection: A Review and comparative study. E3S Web of Conferences, 2022, vol. 351, pp. 01046. https://doi.org/10.1051/e3sconf/202235101046
-
Chen R.-C., Dewi Ch., Huang S.-W., Caraka R.E. Selecting critical features for data classification based on machine learning methods.Journal of Big Data, 2020, vol. 7, no. 1, pp. 52. https://doi.org/10.1186/s40537-020-00327-4
-
Sun P., Wang D., Mok V.C., Shi L. Comparison of feature selection methods and machine learning classifiers for radiomics analysis in glioma grading. IEEE Access, 2019, vol. 7, pp. 102010–102020. https://doi.org/10.1109/access.2019.2928975
-
Nguyen G., Kim D., Nguyen A. The effectiveness of feature attribution methods and its correlation with automatic evaluation scores. Proc. of the 35th Conference on Neural Information Processing Systems (NeurIPS 2021), 2021.
-
Amrhein V., Korner-Nievergelt F., Roth T. The earth is flat (p > 0.05): significance thresholds and the crisis of unreplicable research. PeerJ, 2017, vol. 5, pp. e3544. https://doi.org/10.7717/peerj.3544
-
Kolukisa B., Hacilar H., Goy G., Kus M., Bakir-Gungor B., Aral A., Gungor V.C. Evaluation of classification algorithms, linear discriminant analysis and a new hybrid feature selection methodology for the diagnosis of coronary artery disease. Proc. of the 2018 IEEE International Conference on Big Data (Big Data), 2018, pp. 2232–2238. https://doi.org/10.1109/BigData.2018.8622609
-
Ricciardi C., Valente A.S., Edmunds K., Cantoni V., Green R., Fiorillo A., Picone I., Santini S., Cesarelli M. Linear discriminant analysis and principal component analysis to predict coronary artery disease. Health Informatics Journal, 2020, vol. 26, no. 3, pp. 2181–2192. https://doi.org/10.1177/1460458219899210
-
Breiman L. Random Forests. Machine Learning, 2001, vol. 45, pp. 5–32. https://doi.org/10.1023/A:1010933404324
-
Ke G., Meng Q., Finley T., Wang T., Chen W., Ma W., Ye Q., Liu T.-Y. LightGBM: A highly efficient gradient boosting decision tree.Proc. of the 31st Conference on Neural Information Processing Systems (NIPS 2017), 2017, pp. 3149–3157.
-
Prokhorenkova L., Gusev G., Vorobev A., Dorogush A.V., Gulin A. CatBoost: unbiased boosting with categorical features. Proc. of the 32nd Conference on Neural Information Processing Systems (NeurIPS 2018), 2018.