Menu
Publications
2024
2023
2022
2021
2020
2019
2018
2017
2016
2015
2014
2013
2012
2011
2010
2009
2008
2007
2006
2005
2004
2003
2002
2001
Editor-in-Chief
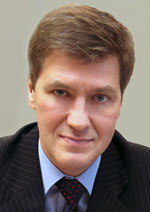
Nikiforov
Vladimir O.
D.Sc., Prof.
Partners
doi: 10.17586/2226-1494-2023-23-4-828-835
Approach to the generalized parameters formation of the complex technical systems technical condition using neural network structures
Read the full article

Article in Russian
For citation:
Abstract
For citation:
Maltsev G.N., Yakimov V.L. Approach to the generalized parameters formation of the complex technical systems technical condition using neural network structures. Scientific and Technical Journal of Information Technologies, Mechanics and Optics, 2023, vol. 23, no. 4, pp. 828–835 (in Russian). doi: 10.17586/2226-1494-2023-23-4-828-835
Abstract
A variant of the generalized parameters process forming of the complex technical systems technical condition is considered. This approach is relevant for modern robotic systems equipped with built-in telemetry tools. The proposed approach to the generalized parameters formation is based on heterogeneous telemetry parameters weighted summation using information about structural and functional relationships in a complex technical system, followed by digital low-frequency filtering of the weighted summation results. This solution makes it possible to increase the complex technical systems technical condition reliability assessment by the values of generalized parameters in the external control loop. The form of generalized parameter representation in the technical condition gradations, which corresponds to the normal functioning, emergency situations and complex technical system partially operational state, is chosen. A multilevel hierarchical model of the generalized parameters formation of the complex technical systems technical condition on the basis of telemeasurements based on a variety of neural network structures allowing to take into account the nonlinear nature of the parameters being telemetered and the mutual influence between them has been developed. The model uses a variety of digital low-frequency filters that reduce the level of disturbances in the generalized parameters time series. The occurrence of disturbances is associated with the uncertainty of changes in the values of the telemetered and generalized parameters near the tolerance limits set by experts with expanded gradations according to technical condition. Information about the limits of tolerances characterizes not only the situations of regular and non-standard functioning, but also the complex technical systems partially operational state. The results of the generalized parameters formation of the spacecraft onboard system technical condition using multilayer neural networks, Kolmogorov–Gabor polynomials and digital filtering methods are presented. The advantages of using multilayer neural networks and median filters in the developed model are shown. The use of generalized parameters will significantly reduce the information load on the transmission channels of telemetry information as well as the means of its processing and analysis in the external control loop. The proposed solutions based on the basic operations of weighted summation and nonlinear transformation can be effectively implemented on promising vector-matrix and tensor processors that support their execution at the hardware level.
Keywords: technical system, technical condition, telemetric parameters, generalized parameter, neural networks, digital filtering
References
References
- Pospelov D.A. Situational Management: Theory and Practice. Moscow, URSS Publ., 2021, 288 p. (in Russian)
- Song Y., Peng Y., Liu D. Model-based health diagnosis for lithium-ion battery pack in space applications. IEEE Transactions on Industrial Electronics, 2021, vol. 68, no. 12, pp. 12375–12384. https://doi.org/10.1109/TIE.2020.3045745
- Ritter N., Straub J. Implementation of hardware-based expert systems and comparison of their performance to software-based expert systems. Machines, 2021, vol. 9, no. 12, pp. 361. https://doi.org/10.3390/machines9120361
- Loskutov A.I., Klykov V.A.Identification and technical diagnostics of onboard equipment of autonomous spacecrafts based on bijective transformations of the set of diagnostic features. Testing. Diagnostics, 2016, no. 4, pp. 57–63. (in Russian). https://doi.org/10.14489/td.2016.04.pp.057-063
- Mozgalevskii A.V., Gaskarov D.V. Technical Diagnostics (Continuous Objects). Moscow, Vysshaja shkola Publ., 1975, 207 p. (in Russian)
- Yakimov V. L., Pankratov A. V. Algorithm of diagnostic signs formation for onboard dynamic systems based on Hurst index. Trudy MAI, 2015, no. 83, pp. 23. (in Russian)
- Yakimov V., Maltsev G. Hybrid network structures and their use in diagnosing complex technical systems. Informatics and Automation, 2022, vol. 21, no. 1, pp. 126–160. (in Russian). https://doi.org/10.15622/ia.2022.21.5
- Maltsev G.N., Nazarov A.V., Yakimov V.L. The study of the diagnosing of automatic spacecraft on-board equipment using a discrete-event simulation model. SPIIRAS Proceedings, 2018, no. 1(56), pp. 95–121. (in Russian). https://doi.org/10.15622/sp.56.5
- Duer S., Valicek J., Paś J., Stawowy M., Bernatowicz D., Duer R., Walczak M. Neural networks in the diagnostics process of low-power solar plant devices. Energies, 2021, vol. 14, no. 9, pp. 2719. https://doi.org/10.3390/en14092719
- Kuz'min A.B. Credibility of tolerance complex industrial system monitoring methods. Avtomatika i Telemekhanika, 1987, no. 10, pp. 59–65. (in Russian)
- Gorban A. Generalized approximation theorem and computational capabilities of neural networks. Sibirskij zhurnal vychislitel'noj matematiki, 1998, vol. 1, no. 1, pp. 12‒24. (in Russian)
- Marushko E.E., Doudkin A.A. Ensembles of neural networks for forecasting of time series of spacecraft telemetry. Optical Memory and Neural Networks, 2017, vol. 26, no. 1, pp. 47–54. https://doi.org/10.3103/S1060992X17010064
- Fayemi O., Di Q., Zhen Q., Wang Y. Adaptive processing for EM telemetry signal recovery: Field data from sichuan province. Energies, 2020, vol. 13, no. 22, pp. 5873. https://doi.org/10.3390/en13225873
- Liu W., Dou Z., Wang W., Liu Y., Zou H., Zhang B., Hou S. Short-term load forecasting based on elastic net improved GMDH and difference degree weighting optimization. Applied Sciences, 2018, vol. 8, no. 9, pp. 1603. https://doi.org/10.3390/app8091603
- Jung J., Sael L. Fast and accurate pseudoinverse with sparse matrix reordering and incremental approach. Machine Learning, 2020, vol. 109, no. 12, pp. 2333–2347. https://doi.org/10.1007/s10994-020-05920-5