Menu
Publications
2024
2023
2022
2021
2020
2019
2018
2017
2016
2015
2014
2013
2012
2011
2010
2009
2008
2007
2006
2005
2004
2003
2002
2001
Editor-in-Chief
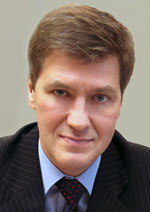
Nikiforov
Vladimir O.
D.Sc., Prof.
Partners
doi: 10.17586/2226-1494-2023-23-3-585-594
Joint recognition of text and layout in historical Russian documents
Read the full article

Article in English
For citation:
Abstract
For citation:
Mohammed S., Teslya N. Joint recognition of text and layout in historical Russian documents. Scientific and Technical Journal of Information Technologies, Mechanics and Optics, 2023, vol. 23, no. 3, pp. 585–594. doi: 10.17586/2226-1494-2023-23-3-585-594
Abstract
In this paper, we evaluated the Document Attention Network (DAN), the first end-to-end segmentation-free architecture on Historical Russian Documents. The DAN model jointly recognizes both text and layout from whole documents, it takes whole documents from any size as an input and output the text as well as logical layout tokens. For comparison purposes, we conduct our experiments on Digital Peter dataset as it has been recognized at line-level. Dataset consists of documents of Peter the Great manuscripts; ground truths are represented according to a sophisticated XML schema which enables an accurate detailed definition of layout and text regions. We achieved good results at page-level: 18.71 % for Character Error Rate (CER), 39.7 % for Word Error Rate (WER), 14.11 % For Layout Ordering Error Rate (LOER), and 66.67 % for mean Average Precision (mAP).
Keywords: document understanding, handwritten text recognition, layout analysis, fully connected networks, transformers
Acknowledgements. The study was carried out at the expense of state funding, topic project No. FFZF-2022-0005.
References
Acknowledgements. The study was carried out at the expense of state funding, topic project No. FFZF-2022-0005.
References
-
Sánchez J., Romero V., Toselli A.H., Vidal E. ICFHR2016 competition on handwritten text recognition on the READ dataset. Proc. of the 15th International Conference on Frontiers in Handwriting Recognition (ICFHR), 2016, pp. 630–635. https://doi.org/10.1109/icfhr.2016.0120
-
Coquenet D., Chatelain C., Paquet T. DAN: a segmentation-free document attention network for handwritten document recognition. IEEE Transactions on Pattern Analysis and Machine Intelligence, 2023, in press. https://doi.org/10.1109/tpami.2023.3235826
-
Vaswani A., Shazeer N., Parmar N., Uszkoreit J., Jones L., Gomez A.N., Kaiser Ł., Polosukhin I. Attention is all you need. Advances in Neural Information Processing Systems 30 (NIPS 2017), 2017, pp. 5998–6008.
-
Pletschacher S., Antonacopoulos A. The PAGE (Page Analysis and Ground-truth Elements) format framework. Proc. of the 20th International Conference on Pattern Recognition, 2010, pp. 257–260. https://doi.org/10.1109/icpr.2010.72
-
Clausner C., Pletschacher S., Antonacopoulos A. Aletheia - An advanced document layout and text ground-truthing system for production environments. Proc. of the International Conference on Document Analysis and Recognition, 2011, pp. 48–52. https://doi.org/10.1109/ICDAR.2011.19
-
Potanin M., Dimitrov D., Shonenkov A., Bataev V., Karachev D., Novopoltsev M., Chertok A. Digital Peter: New dataset, competition and handwriting recognition methods. Proc. of the HIP'21: The 6th International Workshop on Historical Document Imaging and Processing, 2021, pp. 43–48. https://doi.org/10.1145/3476887.3476892
-
Shonenkov A., Karachev D., Novopoltsev M., Potanin M., Dimitrov D. StackMix and blot augmentations for handwritten text recognition. arXiv, 2021, arXiv:2108.11667. https://doi.org/10.48550/arXiv.2108.11667
-
Teslya N., Mohammed S. Deep learning for handwriting text recognition: Existing approaches and challenges. Proc. of the 31st Conference of Open Innovations Association (FRUCT), 2022, pp. 339–346. https://doi.org/10.23919/FRUCT54823.2022.9770912
-
Bluche T., Messina R. Gated convolutional recurrent neural networks for multilingual handwriting recognition. Proc. of the 14th IAPR International Conference on Document Analysis and Recognition (ICDAR). V. 1, 2017, pp. 646–651. https://doi.org/10.1109/ICDAR.2017.111
-
De Sousa Neto A.F., Bezerra B.L.D., Toselli A.H., Lima E.B. HTR-Flor: A deep learning system for offline handwritten text recognition. Proc. of the 33rd SIBGRAPI Conference on Graphics, Patterns and Images (SIBGRAPI), 2020, pp. 54–61. https://doi.org/10.1109/SIBGRAPI51738.2020.00016
-
Shi B., Bai X., Yao C. An end-to-end trainable neural network for image-based sequence recognition and its application to scene text recognition. IEEE Transactions on Pattern Analysis and Machine Intelligence, 2017, vol. 39, no. 11, pp. 2298–2304. https://doi.org/10.1109/TPAMI.2016.2646371
-
Bluche T., Louradour J., Messina R. Scan, attend and read: End-to-end handwritten paragraph recognition with MDLSTM attention. Proc. of the 14th IAPR International Conference on Document Analysis and Recognition (ICDAR). V. 1, 2017, pp. 1050–1055. https://doi.org/10.1109/ICDAR.2017.174
-
Puigcerver J. Are multidimensional recurrent layers really necessary for handwritten text recognition? Proc. of the 14th IAPR International Conference on Document Analysis and Recognition (ICDAR). V. 1, 2017, pp. 67–72. https://doi.org/10.1109/icdar.2017.20
-
Graves A., Fernández S., Gomez F., Schmidhuber J. Connectionist temporal classification: labelling unsegmented sequence data with recurrent neural networks. ICML '06: Proc. of the 23rd International Conference on Machine Learning, 2006, pp. 369–376. https://doi.org/10.1145/1143844.1143891
-
Li M., Lv T., Chen J., Cui L., Lu Y., Florencio D., Zhang C., Li Z., Wei F. TrOCR: Transformer-based optical character recognition with pre-trained models. arXiv, 2021, arXiv:2109.10282. https://doi.org/10.48550/arXiv.2109.10282
-
Dosovitskiy A., Beyer L., Kolesnikov A., Weissenborn D., Zhai X., Unterthiner T., Dehghani M., Minderer M., Heigold G., Gelly S., Uszkoreit J., Houlsby N. An image is worth than 16X16 words: transformers for image recognition. ICLR 2021. Available at: https://openreview.net/pdf?id=YicbFdNTTy (accessed: 23.12.2022).
-
Touvron H., Cord M., Douze M., Massa F., Sablayrolles A., Jégou H. Training data-efficient image transformers & distillation through attention. arXiv, 2020, arXiv:2012.12877. https://doi.org/10.48550/arXiv.2012.12877
-
Bao H., Dong L., Wei F. BEiT: BERT Pre-training of image transformers. arXiv, 2021, arXiv:2106.08254. https://doi.org/10.48550/arXiv.2106.08254
-
Devlin J., Chang M.W., Lee K., Toutanova K. BERT: Pre-training of deep bidirectional transformers for language understanding. Proc. of the 2019 Conference of the North American Chapter of the Association for Computational Linguistics: Human Language Technologies (NAACL). V. 1, 2019, pp. 4171–4186. https://doi.org/https://aclanthology.org/N19-1423
-
Liu Y., Ott M., Goyal N., Du J., Joshi M., Chen D., Levy O., Lewis M., Zettlemoyer L., Stoyanov V. RoBERTa: A robustly optimized bert pretraining approach. arXiv, 2019, arXiv:1907.11692. https://doi.org/10.48550/arXiv.1907.11692
-
Dong L., Yang N., Wang W., Wei F., Liu X., Wang Y., Gao J., Zhou M., Hon H.-W. Unified language model pre-training for natural language understanding and generation. Advances in Neural Information Processing Systems 32 (NeurIPS), 2019.
-
Singh S.S., Karayev S. Full page handwriting recognition via image to sequence extraction. Lecture Notes in Computer Science, 2021, vol. 12823, pp. 55–69. https://doi.org/10.1007/978-3-030-86334-0_4
-
Rouhou A.C., Dhiaf M., Kessentini Y., Ben Salem S. Transformer-based approach for joint handwriting and named entity recognition in historical document. Pattern Recognition Letters, 2022, vol. 155, pp. 128–134. https://doi.org/10.1016/j.patrec.2021.11.010
-
Schreiber S., Agne S., Wolf I., Dengel A., Ahmed S. DeepDeSRT: Deep learning for detection and structure recognition of tables in document images. Proc. of the 14th IAPR International Conference on Document Analysis and Recognition (ICDAR). V. 1, 2017, pp. 1162–1167. https://doi.org/10.1109/icdar.2017.192
-
Ares Oliveira S., Seguin B., Kaplan F. DhSegment: A generic deep-learning approach for document segmentation. Proc. of the 16th International Conference on Frontiers in Handwriting Recognition (ICFHR), pp. 7–12. https://doi.org/10.1109/icfhr-2018.2018.00011
-
Yang X., Yumer E., Asente P., Kraley M., Kifer D., Giles C.L. Learning to extract semantic structure from documents using multimodal fully convolutional neural networks. Proc. of the 30th IEEE Conference on Computer Vision and Pattern Recognition (CVPR), 2017, pp. 4342–4351. https://doi.org/10.1109/cvpr.2017.462
-
Xu Y., Li M., Cui L., Huang S., Wei F., Zhou M. LayoutLM: Pre-training of text and layout for document image understanding. Proc. of the 26th ACM SIGKDD International Conference on Knowledge Discovery & Data Mining, 2020, pp. 1192–1200. https://doi.org/10.1145/3394486.3403172
-
Xu Y., Xu Y., Lv T., Cui L., Wei F., Wang G., Lu Y., Florencio D., Zhang C., Che W., Zhang M., Zhou L. LayoutLMv2: Multi-modal pre-training for visually-rich document understanding. Proc. of the 59th Annual Meeting of the Association for Computational Linguistics and the 11th International Joint Conference on Natural Language Processing. V. 1, 2021, pp. 2579–2591. https://doi.org/10.18653/v1/2021.acl-long.201
-
Biswas S., Banerjee A., Lladós J., Pal U. DocSegTr: An Instance-level end-to-end document image segmentation transformer. arXiv, 2022, arXiv:2201.11438. https://doi.org/10.48550/arXiv.2201.11438
-
Li J., Xu Y., Lv T., Cui L., Zhang C., Wei F. DiT: Self-supervised pre-training for document image transformer. MM '22: Proc. of the 30th ACM International Conference on Multimedia, 2022, pp. 3530–3539. https://doi.org/10.1145/3503161.3547911
-
Coquenet D., Chatelain C., Paquet T. End-to-end handwritten paragraph text recognition using a vertical attention network. IEEE Transactions on Pattern Analysis and Machine Intelligence, 2023, vol. 45, no. 1, pp. 508–524. https://doi.org/10.1109/tpami.2022.3144899
-
Everingham M., Gool Van L., Williams C.K.I., Winn J. The PASCAL Visual Object Classes (VOC) Challenge. International Journal of Computer Vision, 2010, vol. 8, no. 2, pp. 303–338. https://doi.org/10.1007/s11263-009-0275-4
-
Sánchez J.A., Romero V., Toselli A.H., Vidal E. ICFHR2016 competition on handwritten text recognition on the READ dataset. Proc. of the 15th International Conference on Frontiers in Handwriting Recognition (ICFHR), 2016, pp. 630–635. https://doi.org/10.1109/icfhr.2016.0120
-
Grosicki E., Abed H.E. ICDAR 2011 - French handwriting recognition competition. Proc. of the International Conference on Document Analysis and Recognition (ICDAR), 2011, pp. 1459–1463. https://doi.org/10.1109/icdar.2011.290