Меню
Публикации
2024
2023
2022
2021
2020
2019
2018
2017
2016
2015
2014
2013
2012
2011
2010
2009
2008
2007
2006
2005
2004
2003
2002
2001
Главный редактор
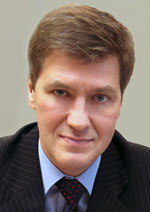
НИКИФОРОВ
Владимир Олегович
д.т.н., профессор
Партнеры
doi: 10.17586/2226-1494-2023-23-3-585-594
УДК 004.932.75
Совместное распознавание текста и оформления в исторических документах на русском языке
Читать статью полностью

Язык статьи - английский
Ссылка для цитирования:
Аннотация
Ссылка для цитирования:
Мохаммед С., Тесля Н. Совместное распознавание текста и оформления в исторических документах на русском языке // Научно-технический вестник информационных технологий, механики и оптики. 2023. Т. 23, № 3. С. 585–594 (на англ. яз.). doi: 10.17586/2226-1494-2023-23-3-585-594
Аннотация
Рассмотрена сквозная, свободная от сегментации архитектура Document Attention Network (DAN), на примере распознавания исторических документов на русском языке. Архитектура DAN способна распознать текст или макет документа любого размера и вывести распознанный текст, а также логические области макета оформления. Выполнено сравнение полученных результатов экспериментов с набором данных Digital Peter, по которому обучены модели распознавания рукописного текста, имеющие высокую точность распознавания на уровне строк. Набор данных состоит из документов рукописей Петра Великого. Эталонные данные для архитектуры DAN представлены в соответствии со сложной схемой формата XML, которая обеспечила точное определение макета оформления и текстовых областей. Получены следующие результаты распознавания текста на уровне страницы: 18,71 % для коэффициента ошибок символов (Character Error Rate, CER), 39,7 % — коэффициента ошибок в словах (Word Error Rate, WER), 14,11 % при упорядочении макета слов (Layout Ordering Error Rate, LOER) и 66,67 % для средней точности (mean Average Precision, mAP).
Ключевые слова: понимание документов, распознавание рукописного текста, анализ макета оформления, полносвязные сети, преобразователи
Благодарности. Исследование выполнено за счет средств государственного финансирования, тема FFZF-2022-0005.
Список литературы
Благодарности. Исследование выполнено за счет средств государственного финансирования, тема FFZF-2022-0005.
Список литературы
1. Sánchez J., Romero V., Toselli A.H., Vidal E. ICFHR2016 competition on handwritten text recognition on the READ dataset // Proc. of the 15th International Conference on Frontiers in Handwriting Recognition (ICFHR). 2016. P. 630–635. https://doi.org/10.1109/icfhr.2016.0120
2. Coquenet D., Chatelain C., Paquet T. DAN: a segmentation-free document attention network for handwritten document recognition // IEEE Transactions on Pattern Analysis and Machine Intelligence. 2023. in press. https://doi.org/10.1109/tpami.2023.3235826
3. Vaswani A., Shazeer N., Parmar N., Uszkoreit J., Jones L., Gomez A.N., Kaiser Ł., Polosukhin I. Attention is all you need // Advances in Neural Information Processing Systems 30 (NIPS 2017). 2017. P. 5998–6008.
4. Pletschacher S., Antonacopoulos A. The PAGE (Page Analysis and Ground-truth Elements) format framework // Proc. of the 20th International Conference on Pattern Recognition. 2010. P. 257–260. https://doi.org/10.1109/icpr.2010.72
5. Clausner C., Pletschacher S., Antonacopoulos A. Aletheia - An advanced document layout and text ground-truthing system for production environments // Proc. of the International Conference on Document Analysis and Recognition. 2011. P. 48–52. https://doi.org/10.1109/ICDAR.2011.19
6. Potanin M., Dimitrov D., Shonenkov A., Bataev V., Karachev D., Novopoltsev M., Chertok A. Digital Peter: New dataset, competition and handwriting recognition methods // Proc. of the HIP'21: The 6th International Workshop on Historical Document Imaging and Processing. 2021. P. 43–48. https://doi.org/10.1145/3476887.3476892
7. Shonenkov A., Karachev D., Novopoltsev M., Potanin M., Dimitrov D. StackMix and blot augmentations for handwritten text recognition // arXiv. 2021. arXiv:2108.11667. https://doi.org/10.48550/arXiv.2108.11667
8. Teslya N., Mohammed S. Deep learning for handwriting text recognition: Existing approaches and challenges // Proc. of the 31st Conference of Open Innovations Association (FRUCT). 2022. P. 339–346. https://doi.org/10.23919/FRUCT54823.2022.9770912
9. Bluche T., Messina R. Gated convolutional recurrent neural networks for multilingual handwriting recognition // Proc. of the 14th IAPR International Conference on Document Analysis and Recognition (ICDAR). V. 1. 2017. P. 646–651. https://doi.org/10.1109/ICDAR.2017.111
10. De Sousa Neto A.F., Bezerra B.L.D., Toselli A.H., Lima E.B. HTR-Flor: A deep learning system for offline handwritten text recognition // Proc. of the 33rd SIBGRAPI Conference on Graphics, Patterns and Images (SIBGRAPI). 2020. P. 54–61. https://doi.org/10.1109/SIBGRAPI51738.2020.00016
11. Shi B., Bai X., Yao C. An end-to-end trainable neural network for image-based sequence recognition and its application to scene text recognition // IEEE Transactions on Pattern Analysis and Machine Intelligence. 2017. V. 39. N 11. P. 2298–2304. https://doi.org/10.1109/TPAMI.2016.2646371
12. Bluche T., Louradour J., Messina R. Scan, attend and read: End-to-end handwritten paragraph recognition with MDLSTM attention // Proc. of the 14th IAPR International Conference on Document Analysis and Recognition (ICDAR). V. 1. 2017. P. 1050–1055. https://doi.org/10.1109/ICDAR.2017.174
13. Puigcerver J. Are multidimensional recurrent layers really necessary for handwritten text recognition? // Proc. of the 14th IAPR International Conference on Document Analysis and Recognition (ICDAR). V. 1. 2017. P. 67–72. https://doi.org/10.1109/icdar.2017.20
14. Graves A., Fernández S., Gomez F., Schmidhuber J. Connectionist temporal classification: labelling unsegmented sequence data with recurrent neural networks // ICML '06: Proc. of the 23rd International Conference on Machine Learning. 2006. P. 369–376. https://doi.org/10.1145/1143844.1143891
15. Li M., Lv T., Chen J., Cui L., Lu Y., Florencio D., Zhang C., Li Z., Wei F. TrOCR: Transformer-based optical character recognition with pre-trained models // arXiv. 2021. arXiv:2109.10282. https://doi.org/10.48550/arXiv.2109.10282
16. Dosovitskiy A., Beyer L., Kolesnikov A., Weissenborn D., Zhai X., Unterthiner T., Dehghani M., Minderer M., Heigold G., Gelly S., Uszkoreit J., Houlsby N. An image is worth than 16X16 words: transformers for image recognition // ICLR 2021 [Электронный ресурс]. URL: https://openreview.net/pdf?id=YicbFdNTTy (дата обращения: 23.12.2022).
17. Touvron H., Cord M., Douze M., Massa F., Sablayrolles A., Jégou H. Training data-efficient image transformers & distillation through attention // arXiv. 2020. arXiv:2012.12877. https://doi.org/10.48550/arXiv.2012.12877
18. Bao H., Dong L., Wei F. BEiT: BERT Pre-training of image transformers // arXiv. 2021. arXiv:2106.08254. https://doi.org/10.48550/arXiv.2106.08254
19. Devlin J., Chang M.W., Lee K., Toutanova K. BERT: Pre-training of deep bidirectional transformers for language understanding // Proc. of the 2019 Conference of the North American Chapter of the Association for Computational Linguistics: Human Language Technologies (NAACL). V. 1. 2019. P. 4171–4186. https://doi.org/https://aclanthology.org/N19-1423
20. Liu Y., Ott M., Goyal N., Du J., Joshi M., Chen D., Levy O., Lewis M., Zettlemoyer L., Stoyanov V. RoBERTa: A robustly optimized bert pretraining approach // arXiv. 2019. arXiv:1907.11692. https://doi.org/10.48550/arXiv.1907.11692
21. Dong L., Yang N., Wang W., Wei F., Liu X., Wang Y., Gao J., Zhou M., Hon H.-W. Unified language model pre-training for natural language understanding and generation // Advances in Neural Information Processing Systems 32 (NeurIPS). 2019.
22. Singh S.S., Karayev S. Full page handwriting recognition via image to sequence extraction // Lecture Notes in Computer Science. 2021. V. 12823. P. 55–69. https://doi.org/10.1007/978-3-030-86334-0_4
23. Rouhou A.C., Dhiaf M., Kessentini Y., Ben Salem S. Transformer-based approach for joint handwriting and named entity recognition in historical document // Pattern Recognition Letters. 2022. V. 155. P. 128–134. https://doi.org/10.1016/j.patrec.2021.11.010
24. Schreiber S., Agne S., Wolf I., Dengel A., Ahmed S. DeepDeSRT: Deep learning for detection and structure recognition of tables in document images // Proc. of the 14th IAPR International Conference on Document Analysis and Recognition (ICDAR). V. 1. 2017. P. 1162–1167. https://doi.org/10.1109/icdar.2017.192
25. Ares Oliveira S., Seguin B., Kaplan F. DhSegment: A generic deep-learning approach for document segmentation // Proc. of the 16th International Conference on Frontiers in Handwriting Recognition (ICFHR). P. 7–12. https://doi.org/10.1109/icfhr-2018.2018.00011
26. Yang X., Yumer E., Asente P., Kraley M., Kifer D., Giles C.L. Learning to extract semantic structure from documents using multimodal fully convolutional neural networks // Proc. of the 30th IEEE Conference on Computer Vision and Pattern Recognition (CVPR). 2017. P. 4342–4351. https://doi.org/10.1109/cvpr.2017.462
27. Xu Y., Li M., Cui L., Huang S., Wei F., Zhou M. LayoutLM: Pre-training of text and layout for document image understanding // Proc. of the 26th ACM SIGKDD International Conference on Knowledge Discovery & Data Mining. 2020. P. 1192–1200. https://doi.org/10.1145/3394486.3403172
28. Xu Y., Xu Y., Lv T., Cui L., Wei F., Wang G., Lu Y., Florencio D., Zhang C., Che W., Zhang M., Zhou L. LayoutLMv2: Multi-modal pre-training for visually-rich document understanding // Proc. of the 59th Annual Meeting of the Association for Computational Linguistics and the 11th International Joint Conference on Natural Language Processing. V. 1. 2021. P. 2579–2591. https://doi.org/10.18653/v1/2021.acl-long.201
29. Biswas S., Banerjee A., Lladós J., Pal U. DocSegTr: An Instance-level end-to-end document image segmentation transformer // arXiv. 2022. arXiv:2201.11438. https://doi.org/10.48550/arXiv.2201.11438
30. Li J., Xu Y., Lv T., Cui L., Zhang C., Wei F. DiT: Self-supervised pre-training for document image transformer // MM '22: Proc. of the 30th ACM International Conference on Multimedia. 2022. P. 3530–3539. https://doi.org/10.1145/3503161.3547911
31. Coquenet D., Chatelain C., Paquet T. End-to-end handwritten paragraph text recognition using a vertical attention network // IEEE Transactions on Pattern Analysis and Machine Intelligence. 2023. V. 45. N 1. P. 508–524. https://doi.org/10.1109/tpami.2022.3144899
32. Everingham M., Gool Van L., Williams C.K.I., Winn J. The PASCAL Visual Object Classes (VOC) Challenge // International Journal of Computer Vision. 2010. V. 8. N 2. P. 303–338. https://doi.org/10.1007/s11263-009-0275-4
33. Sánchez J.A., Romero V., Toselli A.H., Vidal E. ICFHR2016 competition on handwritten text recognition on the READ dataset // Proc. of the 15th International Conference on Frontiers in Handwriting Recognition (ICFHR). 2016. P. 630–635. https://doi.org/10.1109/icfhr.2016.0120
34. Grosicki E., Abed H.E. ICDAR 2011 - French handwriting recognition competition // Proc. of the International Conference on Document Analysis and Recognition (ICDAR). 2011. P. 1459–1463. https://doi.org/10.1109/icdar.2011.290
2. Coquenet D., Chatelain C., Paquet T. DAN: a segmentation-free document attention network for handwritten document recognition // IEEE Transactions on Pattern Analysis and Machine Intelligence. 2023. in press. https://doi.org/10.1109/tpami.2023.3235826
3. Vaswani A., Shazeer N., Parmar N., Uszkoreit J., Jones L., Gomez A.N., Kaiser Ł., Polosukhin I. Attention is all you need // Advances in Neural Information Processing Systems 30 (NIPS 2017). 2017. P. 5998–6008.
4. Pletschacher S., Antonacopoulos A. The PAGE (Page Analysis and Ground-truth Elements) format framework // Proc. of the 20th International Conference on Pattern Recognition. 2010. P. 257–260. https://doi.org/10.1109/icpr.2010.72
5. Clausner C., Pletschacher S., Antonacopoulos A. Aletheia - An advanced document layout and text ground-truthing system for production environments // Proc. of the International Conference on Document Analysis and Recognition. 2011. P. 48–52. https://doi.org/10.1109/ICDAR.2011.19
6. Potanin M., Dimitrov D., Shonenkov A., Bataev V., Karachev D., Novopoltsev M., Chertok A. Digital Peter: New dataset, competition and handwriting recognition methods // Proc. of the HIP'21: The 6th International Workshop on Historical Document Imaging and Processing. 2021. P. 43–48. https://doi.org/10.1145/3476887.3476892
7. Shonenkov A., Karachev D., Novopoltsev M., Potanin M., Dimitrov D. StackMix and blot augmentations for handwritten text recognition // arXiv. 2021. arXiv:2108.11667. https://doi.org/10.48550/arXiv.2108.11667
8. Teslya N., Mohammed S. Deep learning for handwriting text recognition: Existing approaches and challenges // Proc. of the 31st Conference of Open Innovations Association (FRUCT). 2022. P. 339–346. https://doi.org/10.23919/FRUCT54823.2022.9770912
9. Bluche T., Messina R. Gated convolutional recurrent neural networks for multilingual handwriting recognition // Proc. of the 14th IAPR International Conference on Document Analysis and Recognition (ICDAR). V. 1. 2017. P. 646–651. https://doi.org/10.1109/ICDAR.2017.111
10. De Sousa Neto A.F., Bezerra B.L.D., Toselli A.H., Lima E.B. HTR-Flor: A deep learning system for offline handwritten text recognition // Proc. of the 33rd SIBGRAPI Conference on Graphics, Patterns and Images (SIBGRAPI). 2020. P. 54–61. https://doi.org/10.1109/SIBGRAPI51738.2020.00016
11. Shi B., Bai X., Yao C. An end-to-end trainable neural network for image-based sequence recognition and its application to scene text recognition // IEEE Transactions on Pattern Analysis and Machine Intelligence. 2017. V. 39. N 11. P. 2298–2304. https://doi.org/10.1109/TPAMI.2016.2646371
12. Bluche T., Louradour J., Messina R. Scan, attend and read: End-to-end handwritten paragraph recognition with MDLSTM attention // Proc. of the 14th IAPR International Conference on Document Analysis and Recognition (ICDAR). V. 1. 2017. P. 1050–1055. https://doi.org/10.1109/ICDAR.2017.174
13. Puigcerver J. Are multidimensional recurrent layers really necessary for handwritten text recognition? // Proc. of the 14th IAPR International Conference on Document Analysis and Recognition (ICDAR). V. 1. 2017. P. 67–72. https://doi.org/10.1109/icdar.2017.20
14. Graves A., Fernández S., Gomez F., Schmidhuber J. Connectionist temporal classification: labelling unsegmented sequence data with recurrent neural networks // ICML '06: Proc. of the 23rd International Conference on Machine Learning. 2006. P. 369–376. https://doi.org/10.1145/1143844.1143891
15. Li M., Lv T., Chen J., Cui L., Lu Y., Florencio D., Zhang C., Li Z., Wei F. TrOCR: Transformer-based optical character recognition with pre-trained models // arXiv. 2021. arXiv:2109.10282. https://doi.org/10.48550/arXiv.2109.10282
16. Dosovitskiy A., Beyer L., Kolesnikov A., Weissenborn D., Zhai X., Unterthiner T., Dehghani M., Minderer M., Heigold G., Gelly S., Uszkoreit J., Houlsby N. An image is worth than 16X16 words: transformers for image recognition // ICLR 2021 [Электронный ресурс]. URL: https://openreview.net/pdf?id=YicbFdNTTy (дата обращения: 23.12.2022).
17. Touvron H., Cord M., Douze M., Massa F., Sablayrolles A., Jégou H. Training data-efficient image transformers & distillation through attention // arXiv. 2020. arXiv:2012.12877. https://doi.org/10.48550/arXiv.2012.12877
18. Bao H., Dong L., Wei F. BEiT: BERT Pre-training of image transformers // arXiv. 2021. arXiv:2106.08254. https://doi.org/10.48550/arXiv.2106.08254
19. Devlin J., Chang M.W., Lee K., Toutanova K. BERT: Pre-training of deep bidirectional transformers for language understanding // Proc. of the 2019 Conference of the North American Chapter of the Association for Computational Linguistics: Human Language Technologies (NAACL). V. 1. 2019. P. 4171–4186. https://doi.org/https://aclanthology.org/N19-1423
20. Liu Y., Ott M., Goyal N., Du J., Joshi M., Chen D., Levy O., Lewis M., Zettlemoyer L., Stoyanov V. RoBERTa: A robustly optimized bert pretraining approach // arXiv. 2019. arXiv:1907.11692. https://doi.org/10.48550/arXiv.1907.11692
21. Dong L., Yang N., Wang W., Wei F., Liu X., Wang Y., Gao J., Zhou M., Hon H.-W. Unified language model pre-training for natural language understanding and generation // Advances in Neural Information Processing Systems 32 (NeurIPS). 2019.
22. Singh S.S., Karayev S. Full page handwriting recognition via image to sequence extraction // Lecture Notes in Computer Science. 2021. V. 12823. P. 55–69. https://doi.org/10.1007/978-3-030-86334-0_4
23. Rouhou A.C., Dhiaf M., Kessentini Y., Ben Salem S. Transformer-based approach for joint handwriting and named entity recognition in historical document // Pattern Recognition Letters. 2022. V. 155. P. 128–134. https://doi.org/10.1016/j.patrec.2021.11.010
24. Schreiber S., Agne S., Wolf I., Dengel A., Ahmed S. DeepDeSRT: Deep learning for detection and structure recognition of tables in document images // Proc. of the 14th IAPR International Conference on Document Analysis and Recognition (ICDAR). V. 1. 2017. P. 1162–1167. https://doi.org/10.1109/icdar.2017.192
25. Ares Oliveira S., Seguin B., Kaplan F. DhSegment: A generic deep-learning approach for document segmentation // Proc. of the 16th International Conference on Frontiers in Handwriting Recognition (ICFHR). P. 7–12. https://doi.org/10.1109/icfhr-2018.2018.00011
26. Yang X., Yumer E., Asente P., Kraley M., Kifer D., Giles C.L. Learning to extract semantic structure from documents using multimodal fully convolutional neural networks // Proc. of the 30th IEEE Conference on Computer Vision and Pattern Recognition (CVPR). 2017. P. 4342–4351. https://doi.org/10.1109/cvpr.2017.462
27. Xu Y., Li M., Cui L., Huang S., Wei F., Zhou M. LayoutLM: Pre-training of text and layout for document image understanding // Proc. of the 26th ACM SIGKDD International Conference on Knowledge Discovery & Data Mining. 2020. P. 1192–1200. https://doi.org/10.1145/3394486.3403172
28. Xu Y., Xu Y., Lv T., Cui L., Wei F., Wang G., Lu Y., Florencio D., Zhang C., Che W., Zhang M., Zhou L. LayoutLMv2: Multi-modal pre-training for visually-rich document understanding // Proc. of the 59th Annual Meeting of the Association for Computational Linguistics and the 11th International Joint Conference on Natural Language Processing. V. 1. 2021. P. 2579–2591. https://doi.org/10.18653/v1/2021.acl-long.201
29. Biswas S., Banerjee A., Lladós J., Pal U. DocSegTr: An Instance-level end-to-end document image segmentation transformer // arXiv. 2022. arXiv:2201.11438. https://doi.org/10.48550/arXiv.2201.11438
30. Li J., Xu Y., Lv T., Cui L., Zhang C., Wei F. DiT: Self-supervised pre-training for document image transformer // MM '22: Proc. of the 30th ACM International Conference on Multimedia. 2022. P. 3530–3539. https://doi.org/10.1145/3503161.3547911
31. Coquenet D., Chatelain C., Paquet T. End-to-end handwritten paragraph text recognition using a vertical attention network // IEEE Transactions on Pattern Analysis and Machine Intelligence. 2023. V. 45. N 1. P. 508–524. https://doi.org/10.1109/tpami.2022.3144899
32. Everingham M., Gool Van L., Williams C.K.I., Winn J. The PASCAL Visual Object Classes (VOC) Challenge // International Journal of Computer Vision. 2010. V. 8. N 2. P. 303–338. https://doi.org/10.1007/s11263-009-0275-4
33. Sánchez J.A., Romero V., Toselli A.H., Vidal E. ICFHR2016 competition on handwritten text recognition on the READ dataset // Proc. of the 15th International Conference on Frontiers in Handwriting Recognition (ICFHR). 2016. P. 630–635. https://doi.org/10.1109/icfhr.2016.0120
34. Grosicki E., Abed H.E. ICDAR 2011 - French handwriting recognition competition // Proc. of the International Conference on Document Analysis and Recognition (ICDAR). 2011. P. 1459–1463. https://doi.org/10.1109/icdar.2011.290