Меню
Публикации
2024
2023
2022
2021
2020
2019
2018
2017
2016
2015
2014
2013
2012
2011
2010
2009
2008
2007
2006
2005
2004
2003
2002
2001
Главный редактор
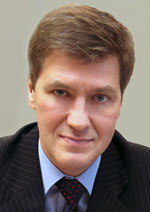
НИКИФОРОВ
Владимир Олегович
д.т.н., профессор
Партнеры
doi: 10.17586/2226-1494-2023-23-5-967-979
УДК 004.65
Кластеризация в аналитике больших данных: системный обзор и сравнительный анализ (обзорная статья)
Читать статью полностью

Язык статьи - английский
Ссылка для цитирования:
Аннотация
Ссылка для цитирования:
Хечми Шили. Кластеризация в аналитике больших данных: системный обзор и сравнительный анализ (обзорная статья) // Научно-технический вестник информационных технологий, механики и оптики. 2023. Т. 23, No 5. С. 967–979 (на англ. яз.). doi: 10.17586/2226-1494-2023-23-5-967-979
Аннотация
В современном мире широкое использование информационных и коммуникационных технологий привело к накоплению огромных и разнообразных объемов данных, широко известных как большие данные. Это обуславливает потребность в новых концепциях и аналитических методах, которые помогают извлекать важные идеи из быстро растущих объемов цифровых данных. Кластеризация — фундаментальный подход, используемый в интеллектуальном анализе данных для извлечения ценной информации. Несмотря на то, что в различных областях описано и реализовано множество методов кластеризации, данное разнообразие усложняет задачу отслеживания последних достижений в области больших данных. Работа направлена на всестороннюю оценку алгоритмов кластеризации, разработанных для больших данных, с выделением их различных функций. Выполнены эмпирические оценки шести больших наборов данных с использованием нескольких показателей достоверности и времени вычислений для оценки производительности рассматриваемых методов кластеризации.
Ключевые слова: большие данные, кластеризация, интеллектуальный анализ данных, эмпирические оценки, показатели производительности
Список литературы
Список литературы
- Hinneburg A., Keim D.A. Optimal grid-clustering: Towards breaking the curse of dimensionality in high-dimensional clustering // Proc. of the 25th International Conference on Very Large Data Bases. 1999. P. 506–517.
- Hinneburg A., Keim D.A. An efficient approach to clustering in large multimedia databases with noise // Proc. of the 4th ACM SIGKDD International Conference on Knowledge Discovery and Data Mining. 1998. P. 58–65.
- Guha S., Rastogi R., Shim K. ROCK: a robust clustering algorithm for categorical attributes // Proc. of the 15th International Conference on Data Engineering. 1999. P. 512–521. https://doi.org/10.1109/icde.1999.754967
- Gennari J.H., Langley P., Fisher D. Models of incremental concept formation // Artificial Intelligence. 1989. V. 40. N 1-3. P. 11–61. https://doi.org/10.1016/0004-3702(89)90046-5
- Kaufman L., Rousseeuw P.J. Finding Groups in Data: an Introduction to Cluster Analysis. John Wiley & Sons, 1990. 342 p.
- Arbelaitz O., Gurrutxaga I., Muguerza J., Pérez J.M., Perona I. An extensive comparative study of cluster validity indices // Pattern Recognition. 2013. V. 46. N 1. P. 243–256. https://doi.org/10.1016/j.patcog.2012.07.021
- Xu D., Tian Y. A comprehensive survey of clustering algorithms // Annals of Data Science. 2015. V. 2. N 2. P. 165–193. https://doi.org/10.1007/s40745-015-0040-1
- Sinaga K.P., Yang M. Unsupervised k-means clustering algorithm // IEEE Access. 2020. V. 8. P. 80716–80727. https://doi.org/10.1109/ACCESS.2020.2988796
- Shili H., Romdhane L.B. IF-CLARANS: intuitionistic fuzzy algorithm for big data clustering // Communications in Computer and Information Science. 2018. V. 854. P. 39–50. https://doi.org/10.1007/978-3-319-91476-3_4
- Karypis G., Han E.H., Kumar V. Chameleon: hierarchical clustering using dynamic modeling // Computer. 1999. V. 32. N 8. P. 68–75. https://doi.org/10.1109/2.781637
- Davies D.L., Bouldin D.W. A cluster separation measure // IEEE Transactions on Pattern Analysis and Machine Intelligence. 1979. V. PAMI-1. N 2. P. 224–227. https://doi.org/10.1109/TPAMI.1979.4766909
- Ankerst M., Breunig M., Kriegel H., Sander J. OPTICS: Ordering points to identify the clustering structure // ACM SIGMOD Record. 1999. V. 28. N 2. P. 49–60. https://doi.org/10.1145/304181.304187
- Cai Z., Wang J., He K. Adaptive density-based spatial clustering for massive data analysis // IEEE Access. 2020. V. 8. P. 23346–23358. https://doi.org/10.1109/ACCESS.2020.2969440
- Wang W., Yang J., Muntz R. STING: a statistical information grid approach to spatial data mining // Proc. of the 23th International Conference on Very Large Data Bases. 1997. P. 186–195.
- Vanschoren J., van Rijn J.N., Bischl B., Torgo L. OpenML: Networked science in machine learning // ACM SIGKDD Explorations Newsletter. 2013. V. 15. N 2. P. 49–60. https://doi.org/10.1145/2641190.2641198
- Goil S., Nagesh H., Choudhary A. MAFIA: Efficient and scalable subspace clustering for very large data sets: Technical Report CPDC-TR-9906-010. 1999.
- Agrawal R., Gehrke J., Gunopulos D., Raghavan P. Automatic subspace clustering of high dimensional data for data mining applications // ACM SIGMOD Record. 1998. V. 27. N 2. P. 94–105. https://doi.org/10.1145/276305.276314
- Dempster P., Laird N.M., Rubin D.B. Maximum likelihood from incomplete data via the em algorithm // Journal of the Royal Statistical Society: Series B (Methodological). 1977. V. 39. N 1. P. 1–38. https://doi.org/10.1111/j.2517-6161.1977.tb01600.x
- Calinski T., Harabasz J. A dendrite method for cluster analysis // Communications in Statistics - Simulation and Computation. 1974. V. 3. N 1. P. 1–27. https://doi.org/10.1080/03610917408548446
- Dunn J. Well-separated clusters and optimal fuzzy partitions // Journal of Cybernetics. 1974. V. 4. N 1. P. 95–104. https://doi.org/10.1080/01969727408546059
- Canbay Y., Sağıroğlu S. Big data anonymization with spark // Proc. of the 2017 International Conference on Computer Science and Engineering (UBMK). 2017. P. 833–838. https://doi.org/10.1109/UBMK.2017.8093543
- Lorbeer B., Kosareva A., Deva B., Softić D., Ruppel P., Küpper A. Variations on the Clustering Algorithm BIRCH // Big Data Research. 2018. V. 11. P. 44–53. https://doi.org/10.1016/j.bdr.2017.09.002
- Tsai C., Huang S. An effective and efficient grid-based data clustering algorithm using intuitive neighbor relationship for data mining // Proc. of the 2015 International Conference on Machine Learning and Cybernetics (ICMLC). 2015. P. 478–483. https://doi.org/10.1109/ICMLC.2015.7340603
- Kailing K., Kriegel H., Kröger P. Density-connected subspace clustering for high-dimensional data // Proc. of the 2014 SIAM International Conference on Data Mining. 2004. P. 246–257. https://doi.org/10.1137/1.9781611972740.23
- Kohonen T. The self-organizing map // Proceedings of the IEEE. 1990. V. 78. N 9. P. 1464–1480. https://doi.org/10.1109/5.58325
- Bandyopadhyay S., Saha S. A point symmetry-based clustering technique for automatic evolution of clusters // IEEE Transactions on Knowledge and Data Engineering. 2008. V. 20. N 11. P. 1441–1457. https://doi.org/10.1109/tkde.2008.79
- Guha S., Rastogi R., Shim K. CURE: an efficient clustering algorithm for large databases // ACM SIGMOD Record. 1998. V. 27. N 2. P. 73–84. https://doi.org/10.1145/276305.276312
- Mahmud M.S., Huang J.Z., Salloum S., Emara T.Z., Sadatdiynov K. A survey of data partitioning and sampling methods to support big data analysis // Big Data Mining and Analytics. 2020. V. 3. N 2. P. 85–101. https://doi.org/10.26599/BDMA.2019.9020015
- Djouzi K., Beghdad-Bey K. A review of clustering algorithms for big data // Proc. of the International Conference on Networking and Advanced Systems (ICNAS). 2019. P. 1–6. https://doi.org/10.1109/ICNAS.2019.8807822
- Fahad A., Alshatri N., Tari Z., Alamri A., Khalil I., Zomaya A.Y., Foufou S., Bouras A. A survey of clustering algorithms for big data: Taxonomy and empirical analysis // IEEE Transactions on Emerging Topics in Computing. 2014. V. 2. N 3. P. 267–279. https://doi.org/10.1109/TETC.2014.2330519
- D’Urso P., De Giovanni L., Massari R. Smoothed self-organizing map for robust clustering // Information Sciences. 2020. V. 512. P. 381–401. https://doi.org/10.1016/j.ins.2019.06.038
- Asuncion A., Newman D.J. UCI Machine Learning Repository. University of California, School of Information and Computer Science, Irvine, CA, 2007.
- Zhang T., Ramakrishnan R., Livny M. BIRCH: a new data clustering algorithm and its applications // Data Mining and Knowledge Discovery. 1997. V. 1. N 2. P. 141–182. https://doi.org/10.1023/A:1009783824328
- MacQueen J. Some methods for classification and analysis of multivariate observations // Proceedings of the fifth Berkeley Symposium Mathematical Statist. Probability. V. 1. 1967. P. 281–297.
- Ng R.T., Han J. Efficient and effective clustering methods for spatial data mining // VLDB '94: Proc. of the 20th International Conference on Very Large Data Bases. 1994. P. 144–144.
- Fisher D.H. Knowledge acquisition via incremental conceptual clustering // Machine Learning. 1987. V. 2. N 2. P. 139–172. https://doi.org/10.1007/bf00114265
- Ester M., Kriegel H.P., Sander J., Xu X. A density-based algorithm for discovering clusters in large spatial data bases with noise // KDD'96: Proc. of the Second International Conference on Knowledge Discovery and Data Mining. 1996. P. 226–231.