Menu
Publications
2024
2023
2022
2021
2020
2019
2018
2017
2016
2015
2014
2013
2012
2011
2010
2009
2008
2007
2006
2005
2004
2003
2002
2001
Editor-in-Chief
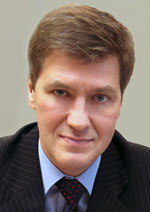
Nikiforov
Vladimir O.
D.Sc., Prof.
Partners
doi: 10.17586/2226-1494-2024-24-2-306-313
From the construction of wavelets based on derivatives of the Gaussian function to the synthesis of filters with a finite impulse response
Read the full article

Article in Russian
For citation:
Abstract
For citation:
Semenov V.I., Chumarov S.G. From the construction of wavelets based on derivatives of the Gaussian function to the synthesis of filters with a finite impulse response. Scientific and Technical Journal of Information Technologies, Mechanics and Optics, 2024, vol. 24, no. 2, pp. 306–313 (in Russian). doi: 10.17586/2226-1494-2024-24-2-306-313
Abstract
For continuous wavelet transformation, wavelets based on derivatives of the Gauss function are used, and for multiscale analysis, Daubechies wavelets are used. The development of algorithms for forward and inverse continuous wavelet transform in the frequency domain made it possible in this work to synthesize digital filters with a finite impulse response (FIR) different from existing methods. The quality of the synthesized filters was checked by decomposition and subsequent reconstruction of the signals. To do this, several filters were synthesized that completely cover the frequency range of the signal. Since wavelets are bandpass filters, the authors called the filters wavelets. The more precisely the reconstructed signal repeats the shape of the original signal, the better the wavelet constructed by one method or another. A comparison of the accuracy of signal reconstruction shows that the best conversion result is obtained by using synthesized wavelets. The impulse responses of the FIR filters are synthesized so that their frequency response are similar to the frequency responses of wavelets based on derivatives of the Gaussian function of a large order. The greater the filter order, the closer the frequency response is to a square-wave shape. Algorithms for forward and inverse wavelet transformation of a signal in the frequency domain using wavelets based on derivatives of the Gauss function are proposed. Profiling of the program shows that the time of the wavelet transforms using the fast Fourier transform is 15,000 times less than with the direct numerical integration for sampling the signal of 32,768 samples. These algorithms can be used for wavelets with a square-wave frequency response. At the same time, the numerical calculation time is halved. The accuracy of the reconstruction was compared for wavelets based on second-order derivatives, Daubechies wavelets, and wavelets with a square-wave frequency response. The reconstruction accuracy is highest for the latest wavelets. The use of the wavelet construction method is preferable since this method is relatively simple and it is easy to synthesize multiband filters with any form of frequency response. If, when synthesizing using existing methods, a short transition band can be obtained only for long impulse responses, while the transition band using the method of constructing wavelets is absent even for filters of very small orders. The paper presents the impulse responses of two- band, three-band digital filters and their frequency response. FIR digital filters with a square-wave frequency response have a higher delay-band attenuation coefficient compared to existing filters, do not have transition band, and can be used to process one-dimensional and two-dimensional signals.
Keywords: wavelet transform, impulse response, reconstruction, multiscale analysis, Mallat algorithm, frequency response, synthesis, digital filter
References
References
- Palani S. Design of finite impulse response (FIR) digital filters. Discrete Time Systems and Signal Processing. Springer, Cham, 2023, pp. 591–732. https://doi.org/10.1007/978-3-031-32421-5_5
- Berber S. Theory of the design, and operation of digital filters. Discrete Communication Systems. Oxford University Press, 2021, pp. 797–823. https://doi.org/10.1093/oso/9780198860792.003.0016
- Kennedy H.L. Lecture notes on the design of low-pass digital filters with wireless-communication applications. arXiv, 2022, arXiv:2211.07123. https://doi.org/10.48550/arXiv.2211.07123
- Agrawal N., Kumar A., Bajaj V., Singh G.K. Design of digital IIR filter: A research survey. Applied Acoustics, 2021, vol. 172, pp. 107669. https://doi.org/10.1016/j.apacoust.2020.107669
- Penedo S.R.M., Netto M.L., Justo J.F. Designing digital filter banks using wavelets. EURASIP Journal on Advances in Signal Processing, 2019, pp. 33. https://doi.org/10.1186/s13634-019-0632-6
- Ďuriš V., Semenov V.I., Chumarov S.G. Application of Continuous Fast Wavelet Transform for Signal Processing. London, Sciemcee Publishing, 2021, 181 p.
- Solonina A.I., Klionskii D.M., Merkucheva T.V., Perov S.N. Digital Signal Processing and MATLAB. St. Petersburg, BHV-Petersburg, 2013, 512 p. (in Russian)
- Daubeches I. Ten Lectures on Wavelets. Society for Industrial and Applied Mathematics, 1992, 357 p.
- Mallat S.G. A Wavelet Tour of Signal Processing. San Diego, CA, USA, Academic Press, 1999, 620 p.
- Novikov L.V. Basics of Wavelet Signal Analysis. Moscow, The Institute for Analytical Instrumentation RAS, 1999, 152 p. (in Russian)
- Zheltov P.V., Semyonov V.I. Wavelet analysis of acoustic signals. Vestnik of KNRTU a.n. A.N.Tupolev, 2008, no. 4, pp. 68–71. (in Russian)
- Zheltov P., Semenov V., Trofimova A., Shurbin A. Algorithms for phoneme identification and forming the word in a speech recognition system based on wavelet-transform. Bulletin of Chuvash University, 2014, no. 2, pp. 98–102. (in Russian)
- Shumarova O.S., Ignatyev S.A. Optimum choice of a wavelet when processing signals of eddy current sensors. Vestnik SGTU, 2013, vol. 4, no. 1(73), pp. 128–132. (in Russian)
- Ďuriš V., Chumarov S.G., Mikheev G.M., Mikheev K.G., Semenov V.I. The orthogonal wavelets in the frequency domain used for the images filtering. IEEE Access, 2020, vol. 8, pp. 211125–211134. https://doi.org/10.1109/ACCESS.2020.3039373
- Ďuriš V., Semenov V.I., Chumarov S.G.. Wavelets and digital filters designed and synthesized in the time and frequency domains. Mathematical Biosciences and Engineering, 2022, vol. 19, no. 3, pp. 3056–3068. https://doi.org/10.3934/mbe.2022141
- Ďuriš V., Chumarov S.G., Semenov V.I. Increasing the speed of multiscale signal analysis in the frequency domain. Electronics, 2023, vol. 12, no. 3, pp. 745. https://doi.org/10.3390/electronics12030745
- Semenov V.I., Chumarov S.G. Reducing the time of inverse wavelet transform of an image using a symmetric orthogonal wavelet. Certificate of registration of a computer program RU 2023664790, 07.07.2023. (in Russian)