Menu
Publications
2024
2023
2022
2021
2020
2019
2018
2017
2016
2015
2014
2013
2012
2011
2010
2009
2008
2007
2006
2005
2004
2003
2002
2001
Editor-in-Chief
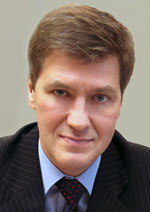
Nikiforov
Vladimir O.
D.Sc., Prof.
Partners
doi: 10.17586/2226-1494-2024-24-4-594-601
Predicting gene-disease associations using a heterogeneous graph neural network
Read the full article

Article in Russian
For citation:
Abstract
For citation:
Sidorenko D.A., Shalyto A.A. Predicting gene-disease associations using a heterogeneous graph neural network. Scientific and Technical Journal of Information Technologies, Mechanics and Optics, 2024, vol. 24, no. 4, pp. 594–601 (in Russian). doi: 10.17586/2226-1494-2024-24-4-594-601
Abstract
The research presents the development of a heterogeneous graph neural network model for predicting gene-disease using existing genomic and medical data. The novelty of the approach is in integrating the principles of graph neural networks and heterogeneous information networks for efficient processing of structured data and consideration of complex genepathology interactions. The solution proposed is a heterogeneous graph neural network which utilizes a heterogeneous graph structure for representing genes, diseases, and their relationships. The performance of the developed model was evaluated on the DisGeNET, LASTFM, YELP datasets. On these datasets, a comparison was made with current SOTA models. The comparison results demonstrated that the proposed model outperforms other models in terms of Average Precision (AP), F1-measure (F1@S), Hit@k, Area Under Receiver Operating Characteristic curve (AUROC) in predicting “gene-disease” associations. The model developed serves as a tool for bioinformatics analysis and can aid researchers and doctors in studying genetic diseases. This could expedite the discovery of new drug targets and the advancement of personalized medicine.
Keywords: machine learning, graph neural networks, heterogeneous information networks, bioinformatics, genetics, “gene-disease” prediction associations
References
References
- Henaff M., Bruna J., LeCun Y. Deep convolutional networks on graph-structured data. arXiv, 2015, arXiv:1506.05163. https://doi.org/10.48550/arXiv.1506.05163
- Wang X., Bo D., Shi C., Fan S., Ye Y., Yu P.S. A survey on heterogeneous graph embedding: methods, techniques, applications and sources. IEEE Transactions on Big Data, 2023, vol. 9, no. 2, pp. 415–436. https://doi.org/10.1109/TBDATA.2022.3177455
- Shao B., Li X., Bian G. A survey of research hotspots and frontier trends of recommendation systems from the perspective of knowledge graph. Expert Systems with Applications, 2021, vol. 165, pp. 113764. https://doi.org/10.1016/j.eswa.2020.113764
- László L. Random walks on graphs: a survey. Combinatorics. V. 2. 1993, pp. 1–46.
- Li L., Wang Y., An L., Kong X., Huang T. A network-based method using a random walk with restart algorithm and screening tests to identify novel genes associated with Menière’s disease. PLOS ONE, 2017, vol. 12, no. 8, pp. e0182592. https://doi.org/10.1371/journal.pone.0182592
- Muslu Ö., Hoyt C.T., Lacerda M., Hofmann-Apitius M., Frohlich H. GuiltyTargets: Prioritization of novel therapeutic targets with network representation learning. IEEE/ACM Transactions on Computational Biology and Bioinformatics, 2022, vol. 19, no. 1, pp. 491–500. https://doi.org/10.1109/TCBB.2020.3003830
- Li Y., Kuwahara H., Yang P., Song L., Gao X. PGCN: Disease gene prioritization by disease and gene embedding through graph convolutional neural networks. biorxiv.org, 2019. https://doi.org/10.1101/532226
- Dutta A., Alcaraz J., TehraniJamsaz A., Cesar E., Sikora A., Jannesari A. Performance optimization using multimodal modeling and heterogeneous GNN. arXiv, 2023, arXiv.2304.12568. https://doi.org/10.48550/arXiv.2304.12568
- Thanapalasingam T., van Berkel L., Bloem P., Groth P. Relational graph convolutional networks: Closer Look. PeerJ Computer Science, 2022, vol. 8, pp. e1073. https://doi.org/10.7717/PEERJ-CS.1073
- Wang X., Ji H., Shi C., Wang B., Ye Y., Cui P., Yu P.S. Heterogeneous graph attention network. Proc. of the WWW '19: The World Wide Web Conference, 2019, pp. 2022–2032. https://doi.org/10.1145/3308558.3313562
- Ali A., Bagchi A. An overview of protein-protein interaction. Current Chemical Biology, 2015, vol. 9, no. 1, pp. 53–65. https://doi.org/10.2174/221279680901151109161126
- Malone J., Holloway E., Adamusiak T., Kapushesky M., Zheng J., Kolesnikov N., Zhukova A., Brazma A., Parkinson H. Modeling sample variables with an experimental factor ontology. Bioinformatics, 2010, vol. 26, no. 8, pp. 1112–1118. https://doi.org/10.1093/bioinformatics/btq099
- Lee J., Yoon W., Kim S., Kim D., Kim S., So C.H., Kang J. BioBERT: a pre-trained biomedical language representation model for biomedical text mining. Bioinformatics, 2020, vol. 36, no. 4, pp. 1234–1240. https://doi.org/10.1093/bioinformatics/btz682
- Zhang X., Zou Y., Shi W. Dilated convolution neural network with LeakyReLU for environmental sound classification. Proc. of the 22nd International Conference on Digital Signal Processing (DSP), 2017. https://doi.org/10.1109/ICDSP.2017.8096153
- Piñero J., Queralt-Rosinach N., Bravo A., Deu-Pons J., Bauer-Mehren A., Baron M., Sanz F., Furlong L.I. DisGeNET: A discovery platform for the dynamical exploration of human diseases and their genes. Database, 2015, vol. 2015. https://doi.org/10.1093/database/bav028
- Alam M., Cevallos B., Flores O., Lunetto R., Yayoshi K., Woo J. Yelp Dataset Analysis using Scalable Big Data. arXiv, 2021, arXiv.2104.08396v1. https://doi.org/10.48550/arXiv.2104.08396
- Li Y., Guo X., Lin W., Zhong M., Li Q., Liu Z., Zhong W., Zhu Z. Learning dynamic user interest sequence in knowledge graphs for click-through rate prediction. IEEE Transactions on Knowledge and Data Engineering, 2023, vol. 35, no. 1, pp. 647–657. https://doi.org/10.1109/TKDE.2021.3073717
- Kuo Y., Wang R., Liu G., Shu Z., Wang N., Zhang R., Yu J., Chen J., Li X., Zhou X. HerGePred: Heterogeneous network embedding representation for disease gene prediction. IEEE Journal of Biomedical and Health Informatics, 2019, vol. 23, no. 4, pp. 1805–1815. https://doi.org/10.1109/JBHI.2018.2870728
- Grover A., Leskovec J. node2vec: Scalable feature learning for networks. Proc. of the KDD’16 . International Conference on Knowledge Discovery & Data Mining, 2016, pp. 855–864. https://doi.org/10.1145/2939672.2939754
- Yuxiao D., Chawla N., Swami A. metapath2vec: Scalable representation learning for heterogeneous networks. Proc. of the KDD’17 . International Conference on Knowledge Discovery & Data Mining, 2017, pp 135–144. https://doi.org/10.1145/3097983.3098036
- Mikolov T., Chen K., Corrado G., Dean J. Efficient Estimation of Word Representations in Vector Space. Proc. of the Workshop ICLR, 2013.
- Perozzi B., Al-Rfou R., Skiena S. DeepWalk: Online learning of social representations. Proc. of the KDD’14. 20th ACM SIGKDD International Conference on Knowledge Discovery and Data Mining, 2014, pp. 701–710. https://doi.org/10.1145/2623330.2623732
- Hu Z., Dong Y., Wang K., Sun Y. Heterogeneous graph transformer. Proc. of the WWW ’20. The Web Conference, 2020, pp. 2704–2710. https://doi.org/10.1145/3366423.3380027
- He M., Huang C., Liu B., Wang Y., Li J. Factor graph-aggregated heterogeneous network embedding for disease-gene association prediction. BMC Bioinformatics, 2021, vol. 22, pp. 165. https://doi.org/10.1186/s12859-021-04099-3