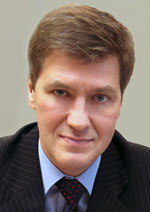
Nikiforov
Vladimir O.
D.Sc., Prof.
doi: 10.17586/2226-1494-2016-16-1-150-160
ROBUST MODIFICATION OF THE LASSO METHOD FOR GENOME-WIDE ASSOCIATION STUDY IN VIEW OF TARGET PHENOTYPE VALUES
Read the full article

For citation: Utkin L.V., Zhuk Yu.A., Coolen F. Robust modification of the Lasso method for genome-wide association study in view of target phenotype values. Scientific and Technical Journal of Information Technologies, Mechanics and Optics, 2016, vol. 16, no. 1, pp. 150–160.
Abstract
A modification of the Lasso method used for genome-wide association study by examples of double haploid lines of barley is proposed for taking into account the additional information about target values of the phenotype which is defined by some feature of plants. From a statistical point of view, a linear regression problem is studied. It is proposed to formalize the additional information about features of plants as intersection of two sets of weights assigned to the training set elements. The first set of weights is produced by means of the interval contamination model. The second set is formed by the pair-wise comparisons of phenotype values. The obtained intersection is convex and is totally defined by its extreme points. This feature allows reducing the Lasso method with sets of weights to a finite set of standard Lasso methods. Results of numerical experiments have showed that the modification provides the better accuracy measures in comparison with the standard Lasso when the training set is small.
Acknowledgements. The study was partially supported by RFBR, research project No. 15-01-01414-a and the Ministry of Education and Science of the Russian Federation, project No. 2014/181-2220.
References
1. Goddard M.E., Wray N.R., Verbyla K., Visscher P.M. Estimating effects and making predictions from genome-wide marker data. Statistical Science, 2009, vol. 24(4), pp. 517–529. doi: 10.1214/09-STS306
2. Altidor W., Khoshgoftaar T.M., Van Hulse J., Napolitano A. Ensemble feature ranking methods for data intensive computing applications. In Handbook of Data Intensive Computing. NY, Springer, 2011, pp. 349–376. doi: 10.1007/978-1-4614-1415-5_13
3. Lee I.-H., Lushington G.H., Visvanathan M. A filter-based feature selection approach for identifying potential biomarkers for lung cancer. Journal of Clinical Bioinformatics, 2011, vol. 1, no. 11 , art. 11. doi: 10.1186/2043-9113-1-11
4. Kohavi R., John G.H. Wrappers for feature subset selection. Artificial Intelligence, 1997, vol. 97, no. 1–2, pp. 273–324.
5. Guyon I., Weston J., Barnhill S., Vapnik V. Gene selection for cancer classification using support vector machines. Machine Learning, 2002, vol. 46, no. 1–3, pp. 389–422. doi: 10.1023/A:1012487302797
6. Lander E.S., Botstein D. Mapping mendelian factors underlying quantitative traits using RFLP linkage maps. Genetics, 1989, vol. 121, no. 1, pp. 185–199.
7. Lal T.N., Chapelle O., Weston J., Elisseeff A. Embedded methods. In Feature Extraction. Springer, 2006. V. 207. P. 137–165. doi: 10.1007/978-3-540-35488-8_6
8. Tibshirani R. Regression shrinkage and selection via the Lasso. Journal of the Royal Statistical Society. Series B (Methodological), 1996, vol. 58, no. 1, pp. 267–288.
9. Zou H., Hastie T. Regularization and variable selection via the elastic net. Journal of the Royal Statistical Society: Series B (Statistical Methodology), 2005, vol. 67, no. 2, pp. 301–320. doi: 10.1111/j.1467-9868.2005.00503.x
10. Gu X., Yin G., Lee J.J. Bayesian two-step Lasso strategy for biomarker selection in personalized medicine development for time-to-event endpoints. Contemporary Clinical Trials, 2013, vol. 36, no. 2, pp. 642–650. doi: 10.1016/j.cct.2013.09.009
11. Hayes B. Overview of statistical methods for genome-wide association studies (GWAS). Methods in Molecular Biology, 2013, vol. 1019, pp. 149–169. doi: 10.1007/978-1-62703-447-0-6
12. Walley P. Statistical Reasoning with Imprecise Probabilities. London: Chapman and Hall, 1991, 362 p.
13. Draper N., Smith H. Applied Regression Analysis. 2nd ed. NY, John Wiley and Sons, 1981, 709 p.
14. Huber P.J. Robust Statistics. NY, Wiley, 1981, 320 p.
15. Chutimanitsakun Y., Nipper R.W., Cuesta-Marcos A., Cistue L., Corey A., Filichkina T., Johnson E.A., Hayes P.M. Construction and application for qtl analysis of a restriction site associated DNA (rad) linkage map in barley. BMC Genomics, 2011, vol. 12, art. 4. doi: 10.1186/1471-2164-12-4
16. Cistue L., Cuesta-Marcos A., Chao S., Echavarri B., Chutimanitsakun Y., Corey A., Filichkina T., Garcia-Marino N., Romagosa I., Hayes P.M. Comparative mapping of the Oregon Wolfe barley using doubled haploid lines derived from female and male gametes. Theoretical and Applied Genetics, 2011, vol. 122, no. 7, pp. 1399–1410. doi: 10.1007/s00122-011-1540-9
17. Hayes P., Chen F.Q., Corey A., Pan A., Chen T.H.H., Baird E., Powell W., Thomas W., Waugh R., Bedo Z., Karsai I., Blake T., Oberthur L. The Dicktoo x Morex population. Plant Cold Hardiness, 1997, pp. 77–87. doi: 10.1007/978-1-4899-0277-1_8