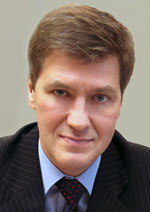
Nikiforov
Vladimir O.
D.Sc., Prof.
doi: 10.17586/2226-1494-2016-16-1-195-197
SPEAKER-DEPENDENT FEATURES FOR SPONTANEOUS SPEECH RECOGNITION
Read the full article

For citation: Medennikov I.P. Speaker-dependent features for spontaneous speech recognition. Scientific and Technical Journal of Information Technologies, Mechanics and Optics, 2016, vol. 16, no. 1, pp. 195–197.
Abstract
This paper presents the results of the study on improving robustness to the acoustic variability of the speech signal for spontaneous speech recognition system. The method is proposed to constructing high-level bottleneck features using deep neural network adapted to a speaker and to acoustic environment with i-vectors. The proposed method provides 11,9% relative reduction of word error rate in Russian spontaneous telephone speech recognition task.
References
1. Vesely K., Ghoshal A., Burget L., Povey D. Sequence-discriminative training of deep neural networks. Proc. of the Annual Conference of International Speech Communication Association, INTERSPEECH. Lyon, France, 2013, pp. 2345‒2349.
2. Saon G., Soltau H., Nahamoo D., Picheny M. Speaker adaptation of neural network acoustic models using i-vectors. Proc. IEEE workshop on Automatic Speech Recognition and Understanding, ASRU. Olomouc, Czech Republic, 2013, pp. 55‒59. doi: 10.1109/ASRU.2013.6707705
3. Soltau H., Saon G., Sainath T.N. Joint training of convolutional and non-convolutional neural networks. Proc. International Conference on Acoustics, Speech and Signal Processing, ICASSP. Florence, Italy, 2014, pp. 5572‒5576. doi: 10.1109/ICASSP.2014.6854669
4. Prudnikov A., Medennikov I., Mendelev V., Korenevsky M., Khokhlov Y. Improving acoustic models for Russian spontaneous speech recognition. Lecture Notes in Computer Science, 2015, vol. 9319, pp. 234‒242. doi: 10.1007/978-3-319-23132-7_29
5. Rouvier M., Favre B. Speaker adaptation of DNN-based ASR with i-vectors: does it actually adapt models to speakers? Proc. Annual Conference of the International Speech Communication Association, INTERSPEECH. Singapore, 2014, pp. 3007‒3011.
6. Kozlov A., Kudashev O., Matveev Y., Pekhovsky T., Simonchik K., Shulipa A. SVID speaker recognition system for NIST SRE 2012. Lecture Notes in Computer Science. Pilsen, Czech Republic, 2013, vol. 8113, pp. 278‒285. doi: 10.1007/978-3-319-01931-4_37
7. Povey D., Ghoshal A., Boulianne G., Burget L., Glembek O., Goel N., Hannemann M., Motlicek P., Qian Y., Schwarz P., Silovsky J., Stemmer G., Vesely K. The Kaldi speech recognition toolkit. Proc. IEEE Workshop on Automatic Speech Recognition and Understanding, ASRU. Waikoloa, USA, 2011, pp. 1‒4.
8. Senior A., Lopez-Moreno I. Improving DNN speaker independence with I-vector inputs. Proc. International Conference on Acoustics, Speech and Signal Processing, ICASSP. Florence, Italy, 2014, pp. 225‒229. doi: 10.1109/ICASSP.2014.6853591
9. Karafiat M., Grezl F., Hannemann M., Cernocky J. But neural network features for spontaneous Vietnamese in BABEL. Proc. Int. Conf. on Acoustics, Speech and Signal Processing, ICASSP. Florence, Italy, 2014, pp. 5622‒5626. doi: 10.1109/ICASSP.2014.6854679