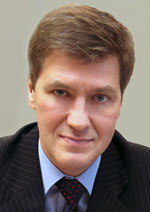
Nikiforov
Vladimir O.
D.Sc., Prof.
doi: 10.17586/2226-1494-2016-16-2-295-302
FORENSIC LINGUISTICS: AUTOMATIC WEB AUTHOR IDENTIFICATION
Read the full article

For citation: Vorobeva A.A. Forensic linguistics: automatic web author identification. Scientific and Technical Journal of Information Technologies, Mechanics and Optics, 2016, vol. 16, no. 2, pp. 295–302, doi:10.17586/2226-1494-2016-16-2-295-302
Abstract
Internet is anonymous, this allows posting under a false name, on behalf of others or simply anonymous. Thus, individuals, criminal or terrorist organizations can use Internet for criminal purposes; they hide their identity to avoid the prosecuting. Existing approaches and algorithms for author identification of web-posts on Russian language are not effective. The development of proven methods, technics and tools for author identification is extremely important and challenging task. In this work the algorithm and software for authorship identification of web-posts was developed. During the study the effectiveness of several classification and feature selection algorithms were tested. The algorithm includes some important steps: 1) Feature extraction; 2) Features discretization; 3) Feature selection with the most effective Relief-f algorithm (to find the best feature set with the most discriminating power for each set of candidate authors and maximize accuracy of author identification); 4) Author identification on model based on Random Forest algorithm. Random Forest and Relief-f algorithms are used to identify the author of a short text on Russian language for the first time. The important step of author attribution is data preprocessing - discretization of continuous features; earlier it was not applied to improve the efficiency of author identification. The software outputs top q authors with maximum probabilities of authorship. This approach is helpful for manual analysis in forensic linguistics, when developed tool is used to narrow the set of candidate authors. For experiments on 10 candidate authors, real author appeared in to top 3 in 90.02% cases, on first place real author appeared in 70.5% of cases.
Acknowledgements. Materials were presented at the conference ISPIT-2015 on Information security and protection of information technology
References
1. Gvozdev A.V., Lebedev I.S. Model' analiza informatsionnykh vozmozhnostei v otkrytykh komp'yuternykh sistemakh. Proc. VII Int. Conf. on Modern Problems of Applied Informatics. St. Petersburg, 2011, pp. 45–47. (In Russian)
2. Vorobeva A.A. Analiz vozmozhnosti primeneniya razlichnykh lingvisticheskikh kharakteristik dlya identifikatsii avtora anonimnykh korotkikh soobshchenii v global'noi seti Internet. Informatia i Kosmos, 2014, no. 1, pp. 42–46. (In Russian)
3. Stamatatos E. A survey of modern authorship attribution methods. Journal of the American Society for Information Science and Technology, 2009, vol. 60, no. 3, pp. 538–556. doi: 10.1002/asi.21001
4. Holmes D.I. The evolution of stylometry in humanities scholarship. Literary and Linguistic Computing, 1998, vol. 13, no. 3, pp. 111–117. doi: 10.1093/llc/13.3.111
5. Abbasi A., Chen H. Applying authorship analysis to extremist-group web forum messages. IEEE Intelligent Systems, 2005, vol. 20, no. 5, pp. 67–75. doi: 10.1109/MIS.2005.81
6. Houvardas J., Stamatatos E. N-gram feature selection for authorship identification. Lecture Notes in Computer Science, 2006, vol. 4183, pp. 77–86.
7. Maitra P., Ghosh S., Das D. Authorship verification: an approach based on random forest. Proc. 6th Conference and Labs of the Evaluation Forum, CLEF 2015. Toulouse, France, 2015.
8. Pacheco M.L., Fernandes K., Porco A. Random forest with increased generalization: a universal background approach for authorship verification. Proc. Conference and Labs of the Evaluation Forum, CLEF 2015. Toulouse, France, 2015.
9. Afroz S. Deception in Authorship Attribution. PhD thesis. Drexel University, 2013, 104 p.
10. Haj Hassan F.I., Chaurasia M.A. N-gram based text author verification. Proc. International Conference on Innovation and Information Management, ICIIM 2012. Chengdu, China, 2012, vol. 36, pp. 67–71.
11. Zheng R., Li J., Chen H., Huang Z. A framework for authorship identification of online messages: writing-style features and classification techniques. Journal of the American Society for Information Science and Technology, 2006, vol. 57, no. 3, pp. 378–393. doi: 10.1002/asi.20316
12. Fomenko V.P., Fomenko T.G. Avtorskii invariant russkikh literaturnykh tekstov. In Fomenko A.T. Novaya Khronologiya Gretsii. Moscow, 1995, vol. 2. (In Russian)
13. Khmelev D.V., Tweedie F.J. Using Markov chains for identification of writers. Literary and Linguistic Computing, 2001, vol. 16, no. 3, pp. 299–307. doi: 10.1093/llc/16.3.299
14. Romanov A.S. Metodika identifikatsii avtora teksta na osnove apparata opornykh vektorov. Doklady TUSUR, 2009, vol. 1, no. 2, pp. 36–42. (In Russian)
15. Kira K., Rendell L.A. A practical approach to feature selection. Proc. 9th International Workshop on Machine Learning, 1992, pp. 249–256. doi: 10.1016/B978-1-55860-247-2.50037-1
16. Kononenko I. Estimating attributes: analysis and extensions of RELIEF. Lecture Notes in Computer Science, 1994, vol. 784, pp. 171–182. doi: 10.1007/3-540-57868-4_57
17. Breiman L. Random forests. Machine Learning, 2001, vol. 45, no. 1, pp. 5–32. doi: 10.1023/A:1010933404324
18. Fatih Amasyali M., Diri B. Automatic Turkish text categorization in terms of author, genre and gender. Lecture Notes in Computer Science, 2006, vol. 3999, pp. 221–226.