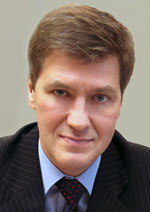
Nikiforov
Vladimir O.
D.Sc., Prof.
doi: 10.17586/2226- 1494-2016-16-5-956-959
ALGORITHM FOR CUMULATIVE CALCULATION OF GENE SET ENRICHMENT STATISTIC
Read the full article

For citation: Sergushichev A.A. Algorithm for cumulative calculation of gene set enrichment statistic. Scientific and Technical Journal of Information Technologies, Mechanics and Optics, 2016, vol. 16, no. 5, pp. 956–959. doi: 10.17586/2226-1494-2016-16-5-956-959
Abstract
Methods for gene set enrichment analysis, widely-used for analysis of gene expression data, were studied. A problem of cumulative calculation of enrichment statistic was considered. For this problem an algorithm based on square root decomposition heuristic was developed. An asymptotic run-time complexity of the algorithm was found. Practical implementation showed an order of magnitude increase in performance compared to a naïve algorithm when run on typical input sizes. The developed algorithm can be used to improve significantly the performance of gene set enrichment analysis.
Acknowledgements. This work was supported by the Russian Federation Government Grant No. 074-U01.
References
1. Mootha V.K., Lindgren C.M., Eriksson K.-F. et al. PGC-1 α-responsive genes involved in oxidative phosphorylation are coordinately downregulated in human diabetes. Nature Genetics, 2003, vol. 34, no. 3, pp. 267–273. doi: 10.1038/ng1180
2. Subramanian A., Tamayo P., Mootha V.K. et al. Gene set enrichment analysis: a knowledge-based approach for interpreting genome-wide expression profiles. Proceedings of the National Academy of Sciences of the United States of America, 2005, vol. 102, no. 43, pp. 15545–15550. doi: 10.1073/pnas.0506580102
3. Maciejewski H. Gene set analysis methods: statistical models and methodological differences. Briefings in Bioinformatics, 2014, vol. 15, no. 4, pp. 504–518. doi: 10.1093/bib/bbt002
4. Tarca A.L., Bhatti G., Romero R. A comparison of gene set analysis methods in terms of sensitivity, prioritization and specificity. PloS ONE, 2013, vol. 8, no. 11, pp. e79217. doi: 10.1371/journal.pone.0079217
5. Yu G., Wang L.-G., Yan G.-R., He Q.-Y. DOSE: an R/Bioconductor package for disease ontology semantic and enrichment analysis. Bioinformatics, 2015, vol. 31, no. 4, pp. 608–609. doi: 10.1093/bioinformatics/btu684
6. Väremo L., Nielsen J., Nookaew I. Enriching the gene set analysis of genome-wide data by incorporating directionality of gene expression and combining statistical hypotheses and methods. Nucleic Acids Research, 2013, vol. 41, no. 8, pp. 4378¬–4391. doi: 10.1093/nar/gkt111
7. Fang Z. GSEAPY: Gene Set Enrichment Analysis in Python. Available at: https://github.com/BioNinja/gseapy (accessed 07.07.2016).
8. Ivabnov M. Sqrt-dekompozitsiya [Sqrt-decomposition]. Available at: http://e-maxx.ru/algo/sqrt_decomposition (accessed 07.07.2016).
9. Cormen T.H., Leiserson C.E., Rivest R.L., Stein C. Introduction to Algorithms. 2nd ed. Cambridge, MIT Press, 2006, 1312 p.