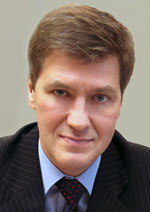
Nikiforov
Vladimir O.
D.Sc., Prof.
doi: 10.17586/2226-1494-2016-16-6-1096-1102
STATISTICAL METHOD OF TERM EXTRACTION FROM CHINESE TEXTS WITHOUT PRELIMINARY SEGMENTATION OF PHRASES
Read the full article

For citation: Bessmertny I.A., Yu Chuqiao, Ma Pengyu. Statistical method of term extraction from Chinese texts without preliminary segmentation of phrases. Scientific and Technical Journal of Information Technologies, Mechanics and Optics, 2016, vol. 16, no. 6, pp. 1096–1102. doi: 10.17586/2226-1494-2016-16-6-1096-1102
Abstract
Subject of Research. The paper considers the problem of automatic term extraction from natural language texts (text mining). One of the first-priority problems in this topic is creation of domain thesaurus. Some well approved methods of terms extraction exist for alphabetic languages, for instance, the latent semantic analysis. Applying of these methods for hieroglyphic texts is challenged because of missing blanks between words. The sentences segmentation task in hieroglyphic languages is usually solved by dictionaries or by statistical methods, particularly, by means of a mutual information approach. Methods of sentences segmentation, as methods of terms extraction, separately, do not reach 100 percent accuracy and fullness, and their consistent applying just increases a number of errors. The aim of this work is improving the fullness and accuracy of domain terms extraction from hieroglyphic texts. Method.The proposed method lies in detection of repeating two, three or four symbol sequences in each sentence and correlation of occurrence frequencies for these sequences in domain and contrast documents collection. According to research carried out it was stated that a trivial ranging of all possible symbol sequences enables to extract satisfactory only frequently using terms. Filtering of symbol sequences by their ratio of frequencies in the domain and contrast collection gave the possibility to extract reliably frequently used terms and find satisfactory rare domain terms. Some results of terms extraction for the “Network technologies” domain from a Chinese text are presented in this paper. A set of articles from the newspaper “Rénmín Rìbào” was used as a contrast collection and some satisfactory results were obtained.
References
1. Joachims T. Learning to Classify Text Using Support Vector Machines: Methods, Theory and Algorithms. Kluwer Academic Publishers, 2002, 205 p.
2. Wallach H.M. Topic modeling: beyond bag-of-words. Proc. 23rd Int. Conf. on Machine Learning. Pittsburgh, USA, 2006, pp. 977–984.
3. Nugumanova A., Bessmertnyi I. Applying the latent semantic analysis to the issue of automatic extraction of collocations from the domain texts. Communications in Computer and Information Science, 2013, vol. 394, pp. 92–101. doi: 10.1007/978-3-642-41360-5_8
4. Taiwanese Principles of Text Segmentation. Available at: http://ip194097.ntcu.edu.tw/TG/CompLing/hunsu/hunsu.htm (accessed 28.10.2016).
5. Xue N. Chinese word segmentation as character tagging. Computational Linguistics and Chinese Language Processing, 2003, vol. 8, no. 1, pp. 29–48.
6. Zeng D., Wei D., Chau M., Wang F. Domain-specific Chinese word segmentation using suffix tree and mutual information. Information Systems Frontiers, 2011, vol. 13, no. 1, pp. 115–125. doi: 0.1007/s10796-010-9278-5
7. Huang Lei, Wu Yan-Peng, Zhu Qun-Feng. Research and improvement of TFIDF feature weighting method. Computer Science, 2014, vol. 41, no. 6, pp. 204–208.
8. Li Xiaochao, Zhao Shang, Lao Yan, Chen Min, Liu Mengmeng. Statistics law of same frequency words in Chinese texts and its application to keywords extraction. Application Research of Computers, vol. 33, no. 4, pp. 1007–1012.
9. Conrado M.S., Pardo T.A.S., Rezende S.O. A machine learning approach to automatic term extraction using a rich feature set. Proc. NAACL HLT Student Research Workshop. Atlanta, USA, 2013, pp. 16–23.
10. Ahmad K., Gillam L., Tostevin L. University of surrey participation in TREC8: weirdness indexing for logical document extrapolation and retrieval (WILDER). Proc. 8th Text Retrieval Conference TREC. Gaithersburg, USA, 1999, pp. 717.
11. Penas A., Verdejo F., Gonzalo J. Corpus-based terminology extraction applied to information access. Proceedings of Corpus Linguistics, 2001, vol. 2001, pp. 458–465.
12. Kim S.N., Baldwin T., Kan M.-Y. An unsupervised approach to domain-specific term extraction. Proc. Australasian Language Technology Association Workshop, 2009, pp. 94–98.
13. Basili R. A contrastive approach to term extraction. Proc. 4th Terminological and Artificial Intelligence Conference, TIA2001. Nancy, France, 2001.
14. Wong W., Liu W., Bennamoun M. Determining termhood for learning domain ontologies using domain prevalence and tendency. Proc. 6th Australasian Conference on Data Mining and Analytics. Gold Coast, Australia, 2007, vol. 70, pp. 47–54.
15. Yang Y., Pedersen J.O. A comparative study on feature selection in text categorization. Proc. 14th Int. Conf. on Machine Learning ICML, 1997, vol. 97, pp. 412–420.
16. Astrakhantsev N.A. Automatic term acquisition from domain-specific text collection by using Wikipedia. Trudy ISP RAN, 2014, vol. 26, no. 4, pp. 7–20. (In Russian)
17. Nugumanova A.B., Bessmertnyi I.A., Petsina P., Baiburin E.M. Semantic relations in text classification based on Bag-of-words model. Programmnye Produkty i Sistemy, 2016, no. 2, pp. 89–99.