Menu
Publications
2024
2023
2022
2021
2020
2019
2018
2017
2016
2015
2014
2013
2012
2011
2010
2009
2008
2007
2006
2005
2004
2003
2002
2001
Editor-in-Chief
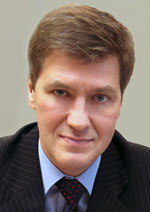
Nikiforov
Vladimir O.
D.Sc., Prof.
Partners
doi: 10.17586/2226-1494-2017-17-4-749-752
METHOD OF AUTOMATIC PAUSE PLACEMENT FOR KAZAKH LANGUAGE
Read the full article

Article in Russian
For citation: Kaliyev A. Method of automatic pause placement for Kazakh language. Scientific and Technical Journal of Information Technologies, Mechanics and Optics, 2017, vol. 17, no. 4, pp. 749–752 (in Russian). doi: 10.17586/2226-1494-2017-17-4-749-752
Abstract
For citation: Kaliyev A. Method of automatic pause placement for Kazakh language. Scientific and Technical Journal of Information Technologies, Mechanics and Optics, 2017, vol. 17, no. 4, pp. 749–752 (in Russian). doi: 10.17586/2226-1494-2017-17-4-749-752
Abstract
The paper considers a new pausing method for intonational speech synthesis systems based on the analysis of distributional semantics in large text corpora. The support vector machine and two speech corpora in Kazakh were used for pause prediction. The prediction of pause places was carried out at the level of bigrams, where the input parameters of the bigram were the vector representations of both of its words and their bit string representation in the Brown cluster model. The carried out studies have shown that the proposed pausing method for the automatic speech synthesis systems for the Kazakh language in the narrative style provides high accuracy of pause placement. The importance of homogeneous data usage was confirmed experimentally for solving such problems. Such approach can facilitate the creation of automatic speech synthesis for many languages.
Keywords: speech synthesis, pauses, clustering, text corpora, prosody
Acknowledgements. This work was financially supported by the Government of the Russian Federation, Grant No. 616029.
References
Acknowledgements. This work was financially supported by the Government of the Russian Federation, Grant No. 616029.
References
1. Brown P.F., Desouza P.V., Mercer R.L. et. al. Class-based n-gram models of natural language. Computational Linguistics, 1992, vol. 18, pp. 467–479.
2. Stratos K., Kim D., Collins M., Hsu D. A spectral algorithm for learning classbased n-gram models of natural language. Proc. 30th Conf. on Uncertainty in Artificial Intelligence. Quebec, Canada, 2014, pp. 762–771.
3. Miller S., Guinness J., Zamanian A. Name tagging with word clusters and discriminative training. Proc. Human Language Technologies and North American Association for Computational Linguistics, 2004, vol. 4, pp. 337–342.
4. Koo T., Carreras X., Collins M. Simple semi-supervised dependency parsing. Proc. 46th Annual Meeting of the Association for Computational Linguistics, ACL-08: HLT. Columbus,USA,2008, pp. 595–603.
5. Lancia F. Word Co-occurrence and Theory of Meaning. 2005. Available at:
www.soc.ucsb.edu/faculty/mohr/classes/soc4/summer_08/pages/Resources/Readings/TheoryofMeaning.pdf
(accessed: 25.04.2017).
6. Cortes C., Vapnik V. Support vector networks. Machine Learning, 1995, vol. 20, no. 3, pp. 273–297. doi: 10.1023/A:1022627411411
7. Rijsbergen C.J.V. Information Retrieval. 2nd ed. London, Butterworths, 1979, 152 p.
8. Chistikov P.G., Khomitsevich O.G. Improving prosodic break detection in a Russian TTS system. Lecture Notes in Computer Science, 2013, vol. 8113, pp. 181–188. doi: 10.1007/978-3-319-01931-4_24