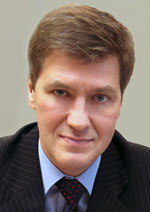
Nikiforov
Vladimir O.
D.Sc., Prof.
doi: 10.17586/2226-1494-2018-18-2-313-321
ANALYSIS OF USERS’ PROTECTION FROM SOCIO-ENGINEERING ATTACKS: SOCIAL GRAPH CREATION BASED ON INFORMATION FROM SOCIAL NETWORK WEBSITES
Read the full article

For citation: Abramov M.V., Tulupyev A.L., Suleymanov A.A. Analysis of users’ protection from socio-engineering attacks: social graph creation based on information from social network websites. Scientific and Technical Journal of Information Technologies, Mechanics and Optics, 2018, vol. 18, no. 2, pp. 313–321 (in Russian). doi: 10.17586/2226-1494-2018-18-2-313-321
Abstract
Subject of Research. The paper deals with accounts in social network websites as a source of information about the intensity of communication between employees in the team. On their basis we form success probability estimates for the spread of malefactorsocio-engineering attack on the user. Scope of Research. The research goal is to build a success assessment for malefactormulti-pass socio-engineering attack on the user based on information obtained from the accounts of company employees in social network websites which characterizes communication intensity between them. The research is aimed at development of models and algorithms for socio-engineering attack spreading on the collapsed social graph of the company and description of methods for calculation of security estimates for the information system users from multi-pass socio-engineering attacks, such attacks, where the target and the entry point do not match. Method. The methods are used of information searching, comparing and analyzing, which characterizes communication intensity between company employees, and data extracted from their accounts in social network websites. Success probability estimate of multi-pass socio-engineering attack reduces to probability estimate creation of a complex event. Main Results. A formula is presented for calculating of probability estimates of socio-engineering attack propagation between users. The estimates obtained in this way are compared to the arcs in the company's social graph, which is used in turn to assess the success probability of a multi-pass socio-engineering attack, the attack, passing through a chain of users. In the earlier studies, estimates of probabilities were defined expertly. The advantages of calculation automating of probability estimates based on data received from social network websites are described. Research Novelty.The paper considers approaches to probabilistic estimates of multi-pass socio-engineering attack success where attacks are intermediate, non-direct, and non-reducible to a single malefactoract. These estimates take into account user’s links in his or her social graph; the parameters of those links are based on the data obtained from social media/networks. Practical Relevance.The approach proposed in this paper provides the basis for further analysis of possible propagation trajectories of multi-pass social engineering attacks, as well as calculation of the probability of each such trajectory that in turn helps to expand the number of factors affecting the security evaluation of the information system users, and gives the possibility to set the backtracking task for attacks in one of the successful forms for finding solutions.
Acknowledgements. The research was carried out in the framework of the project on SPIIRAS state assignment No. 0073-2014-0002, with the financial support of the RFBR (project No. 16-31-00373 “Methods for parameters identifying of social processes from incomplete information based on probabilistic graphic models”; project No. 18-01-00626 “Methods of representation, synthesis of truth estimates and machine learning in algebraic Bayesian networks and related knowledge models with uncertainty: the logic-probability approach and graph systems”); project No. 18-37-00323 – Socio-engineering attacks in corporate information systems: approaches, methods and algorithms for identifying the most probable trajectories.
References
-
Liu J., Lyu Q., Wang Q., Yu X. A digital memories based user authentication scheme with privacy preservation. PloS ONE, 2017, vol. 12, no. 11, art. e0186925. doi: 10.1371/journal.pone.0186925
-
van Schaik P., Jeske D., Onibokun J., Coventry L., Jansen J., Kusev P. Risk perceptions of cyber-security and precautionary behavior. Computers in Human Behavior, 2017, vol. 75, pp. 547–559.
-
The Human Factor in IT Security: How Employees are Making Businesses Vulnerable from Within. Kaspersky Lab. 2017. URL: https://www.kaspersky.com/blog/the-human-factor-in-it-security/ (accessed: 06.10.2017)
-
Anoshin I. Card weaknesses. How not to become a victim of high-tech scammers. RBK, Newspaper, 2017, no. 164. URL: http://www.rbc.ru/newspaper/2017/09/29/59ca447b9a79474aa6f65673 (accessed: 06.10.2017)
-
Antonyuk E.M., Varshavsky I.E., Antonyuk P.E. Adaptive systems of automatic control with prioritized channels. Proc. 20th IEEE Int. Conf. on Soft Computing and Measurements. St. Petersburg, 2017, pp. 539–540. doi: 10.1109/SCM.2017.7970643
-
Desnitsky V.A., Kotenko I.V. Modeling and analysis of security incidents for mobile communication mesh Zigbee-based network. Proc. 20th IEEE Int. Conf. on Soft Computing and Measurements. St. Petersburg, 2017, pp. 500–502. doi: 10.1109/SCM.2017.7970629
-
Du J., Jiang C., Chen K.C., Ren Y., Poor H.V. Community-structured evolutionary game for privacy protection in social networks. IEEE Transactions on Information Forensics and Security, 2018, vol. 13, no. 3, pp. 574–589. doi: 10.1109/TIFS.2017.2758756
-
Gupta B.B., Tewari A., Jain A.K., Agrawal D.P. Fighting against phishing attacks: state of the art and future challenges. Neural Computing and Applications, 2017, vol. 28, no. 12, pp. 3629–3654. doi: 10.1007/s00521-016-2275-y
-
Kotenko I., Chechulin A., Branitskiy A. Generation of source data for experiments with network attack detection software. Journal of Physics: Conference Series, 2017, vol. 820, no. 1, art. 012033. doi: 10.1088/1742-6596/820/1/012033
-
Azarov A.A., Tulup'eva T.V., Suvorova A.V., Tulup'ev A.L., Abramov M.V., Yusupov R.M. Socio-Engineering Attacks: Problems of Analysis. St. Petersburg, Nauka Publ., 2016, 352 p. (in Russian)
-
Mitnik K.D., Saimon V.L. Art of Deceit. Moscow, IT Company Publ., 2004, 416 p. (in Russian)
-
Ding D., Han Q.L., Xiang Y., Ge X., Zhang X.M. A survey on security control and attack detection for industrial cyber-physical systems. Neurocomputing, 2018, vol. 275, pp. 1674–1683. doi: 10.1016/j.neucom.2017.10.009
-
Corbellini A., Godoy D., Mateos C., Schiaffino S., Zunino A. DPM: A novel distributed large-scale social graph processing framework for link prediction algorithms. Future Generation Computer Systems, 2017, vol. 78, pp. 474–480.doi: 10.1016/j.future.2017.02.025
-
Su S., Li X., Cheng X., Sun C. Location‐aware targeted influence maximization in social networks. Journal of the Association for Information Science and Technology, 2018, vol. 69, no. 2, pp. 229–241.
-
Suvorova A.V., Tulup'eva T.V., Tulup'ev A.L., Sirotkin A.V., Pashchenko A.E. Probabilistic graphical models of individual socially significant behavior on the base of incomplete data. SPIIRAS Proceedings, 2012, vol. 3, no. 22, pp. 101–112. (in Russian)
-
Shindarev N., Bagretsov G., Abramov M., Tulupyeva T., Suvorova A. Approach to identifying of employees profiles in websites of social networks aimed to analyze social engineering vulnerabilities. Advances in Intelligent Systems and Computing, 2017, vol. 679, pp. 441–447. doi: 10.1007/978-3-319-68321-8_45
-
Social Networks in Russia. Mail.Ru Group 2014. URL: https://corp.imgsmail.ru/media/files/issledovanie-auditorij-sotcialnykh-setej.pdf (accessed: 20.01.2018).
-
Bagretsov G.I., Shindarev N.A., Abramov M.V., Tulupyeva T.V. Approaches to development of models for text analysis of information in social network profiles in order to evaluate user’s vulnerabilities profile. Proc. 20th IEEE Int. Conf. on Soft Computing and Measurements. St. Petersburg, 2017, pp. 93–95.
-
Mal'chevskaya E.A., Birillo A.I., Kharitonov N.A., Zolotin A.A. Elaboration of local priori inference matrix-vector approach in algebraic Bayesian networks. Proc. NSMV-2017. St. Petersburg, 2017, vol. 1, pp. 92–100. (in Russian)
-
Tulup'eva T.V. Tulup'ev A.L., Pashchenko A.E., Azarov A.A., Stepashkin M.V. Social psychological factors that influence the information system users vulnerability degree in regard of socio-engineering attacks. SPIIRAS Proceedings, 2010, vol. 1, no. 12, pp. 200–214. (in Russian)
-
Abramov M.V., Azarov A.A., Tulup'eva T.V., Tulup'ev A.L. Model of malefactor competencies profile for analyzing information system personnel security from social engineering attacks. Information and Control Systems, 2016, vol. 83, no. 4, pp. 77–84. (in Russian) doi: 10.15217/issn1684-8853.2016.4.77
-
Baccelli F., Chatterjee A., Vishwanath S. Pairwise stochastic bounded confidence opinion dynamics: heavy tails and stability. IEEE Transactions on Automatic Control, 2017, vol. 62, no. 11, pp. 5678–5693. doi: 10.1109/TAC.2017.2691312
-
Bell D.C., Trevino R.A. Modeling HIV risk. Journal of Acquired Immune Deficiency Syndromes and Human Retrovirology, 1999, vol. 22, no. 3, pp. 280–287.
-
Samsonovich A.V. On a roadmap for the BICA challenge. Biologically Inspired Cognitive Architectures, 2012, vol. 1, pp. 100–107. doi: 10.1016/j.bica.2012.05.002
-
Ginni Rometty on the End of Programming. Bloomberg, 2017. URL: https://www.bloomberg.com/news/features/2017-09-20/ginni-rometty-on-artificial-intelligence (accessed: 06.10.2017)