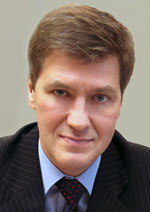
Nikiforov
Vladimir O.
D.Sc., Prof.
doi: 10.17586/2226-1494-2018-18-2-350-352
FEATURE COMBINATION FOR THE TASK OF NEURAL NETWORK ACOUSTIC MODEL LEARNING
Read the full article

For citation: Romanenko A.N. Feature combination for the task of neural network acoustic model learning. Scientific and Technical Journal of Information Technologies, Mechanics and Optics, 2018, vol. 18, no. 2, pp. 350–352 (in Russian). doi: 10.17586/2226-1494-2018-18-2-350-352
Abstract
A method of feature combination for the problem of neural network acoustic models training is proposed aimed at the quality improvement of speech recognition. Unlike the feeding of a concatenated vector of features of a different nature to the neural network input, the proposed method uses a delayed union at the level of hidden layers. It uses individual input streams for each type of features. Such streams are able to extract specific patterns for each type of features and then combine them on the hidden layer of the neural network acoustic model. The effect of the method on the system quality was studied in the task of Russian conversational telephone speech recognition. The proposed method achieves 0.41% absolute reduction of the word error rate relative to the concatenation of features and 1.35% in comparison with the best system using one type of features. The results of the work can be used to develop automatic speech recognition systems.
Acknowledgements. The research is supported by the Ministry of Education and Science of the Russian Federation, contract No. 8.9971.2017/DAAD
References
-
Siohan O., Rybach D. Multitask learning and system combination for automatic speech recognition. Proc. IEEE Workshop on Automatic Speech Recognition and Understanding. Scottsdale, USA, 2015, pp. 589–595. doi: 10.1109/ASRU.2015.7404849
-
Saon G., Kurata G., Sercu T. et al. English conversational telephone speech recognition by humans and machines. Proc. INTERSPEECH. Stockholm, Sweden, 2017, pp. 132–136. doi: 10.21437/Interspeech.2017-405
-
Narang S., Elsen E., Diamos G., Sengupta S. Exploring sparsity in recurrent neural networks. Proc. International Conference on Learning Representations, ICLR. Toulon, France, 2017. arXiv:1704.05119
-
Zolnay A., Schluter R., Ney H. Acoustic feature combination for robust speech recognition. Proc. IEEE Int. Conf. on Acoustics, Speech and Signal Processing. Philadelphia, USA, 2005, pp. I457–I460. doi: 10.1109/ICASSP.2005.1415149
-
Pulkki V., Karjalainen M. Communication Acoustics: An Introduction to Speech, Audio and Psychoacoustics. Wiley, 2015, 454 p.
-
Ghahremani P., BabaAli B., Povey D. at al. A pitch extraction algorithm tuned for automatic speech recognition. Proc. Int. Conf. on Acoustics, Speech and Signal Processing. Florence, Italy, 2014, pp. 2494–2498. doi: 10.1109/ICASSP.2014.6854049
-
Grezl F., Karafiat M., Kontar S. Probabilistic and bottle-neck features for LVCSR of meetings. Proc. IEEE Int. Conf. on Acoustics, Speech and Signal Processing, ICASSP. Honolulu, USA, 2007, vol. 4, pp. IV757–IV760. doi: 10.1109/ICASSP.2007.367023
-
Medennikov I.P. Speaker-dependent features for spontaneous speech recognition. Scientific and Technical Journal of Information Technologies, Mechanics and Optics, 2016, vol. 16, no. 1, pp. 195–197. (In Russian) doi: 10.17586/2226-1494-2016-16-1-195-197
-
Khokhlov Y., Medennikov I., Romanenko A. et al. The STC keyword search system for OpenKWS 2016 evaluation. Proc. INTERSPEECH. Stockholm, Sweden, 2017, pp. 3602–3606. doi: 10.21437/Interspeech.2017-1212
-
Medennikov I.P. Methods, Algorithms and Software for Recognition of Russian Spontaneous Phone Speech. Dis. PhD Eng. Sci. St. Petersburg, Russia, 200 p.
-
Peddinti V., Povey D., Khudanpur S. A time delay neural network architecture for efficient modeling of long temporal contexts. Proc. INTERSPEECH. Dresden, Germany, 2015, pp. 3214–3218.