Menu
Publications
2025
2024
2023
2022
2021
2020
2019
2018
2017
2016
2015
2014
2013
2012
2011
2010
2009
2008
2007
2006
2005
2004
2003
2002
2001
Editor-in-Chief
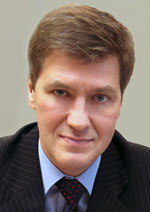
Nikiforov
Vladimir O.
D.Sc., Prof.
Partners
doi: 10.17586/2226-1494-2018-18-3-447-456
CROSS-DOMAIN WEB AUTHOR IDENTIFICATION
Read the full article

Article in Russian
For citation: Vorobeva A.A., Pozvolenko V.A., Korobitsyna A.S., Sharafiev A.A. Cross-domain web author identification. Scientific and Technical Journal of Information Technologies, Mechanics and Optics, 2018, vol. 18, no. 3, pp. 447–456 (in Russian). doi: 10.17586/2226-1494-2018-18-3-447-456
Abstract
For citation: Vorobeva A.A., Pozvolenko V.A., Korobitsyna A.S., Sharafiev A.A. Cross-domain web author identification. Scientific and Technical Journal of Information Technologies, Mechanics and Optics, 2018, vol. 18, no. 3, pp. 447–456 (in Russian). doi: 10.17586/2226-1494-2018-18-3-447-456
Abstract
The paper is devoted to the cross-domain web author attribution (identification), where user's messages are obtained from several sources (web-sites). We focused on the problem of one web-site user identification by his messages from another web-site. We found that there is a stylistic difference between the texts of messages created by one user on different web-sites. The possibility of a single feature space forming for texts received from various sources was determined providing sufficient accuracy of linguistic identification. Two subtasks were studied: 1) mixed sources – training and test datasets include messages from mixed sources (web-sites); 2) separated sources – the text messages sources of the training and test datasets do not intersect; training dataset includes texts from one source, test dataset includes texts from another.The experiment results showed that identification accuracy in mixed sources task is 0.82. The accuracy in separated sources task is 0.74. It is concluded that there is a stylistic difference between texts created by one user, but on the various web-sites. But at the same time, it is possible to form a single feature space for text messages received from various web-sites, ensuring sufficient identification accuracy.
Keywords: web users' identification, forensic linguistics, linguistic identification, cross-domain identification, author attribution
References
References
-
Chen C., Wu K., Srinivasan V., Zhang X. Battling the internet water army: detection of hidden paid posters. Proc. IEEE/ACM Int. Conf. on Advances in Social Networks Analysis and Mining, ASONAM. Niagara Falls, Canada, 2013, pp. 116–120. doi: 10.1145/2492517.2492637
-
Lebedev I.S., Borisov Y.B. Formalization models of natural-language messages in information security monitoring systems of open computer networks. Information and Control Systems, 2011, no. 2, pp. 37–43.(in Russian)
-
Kataeva V.A., Pantyuhin I.S., Yurin I.V. Estimation method of the cohesion degree for the users’ profiles of social network based on open data. Open Education, 2017, vol. 21, no. 6, pp. 14–22. (in Russian) doi: 10.21686/1818-4243-2017-6-14-22
-
Vorobeva A.A. Dynamic feature selection for web user identification on linguistic and stylistic features of online texts. Scientific and Technical Journal of Information Technologies, Mechanics and Optics, 2017, vol. 17, no. 1, pp. 117–128. (in Russian) doi: 10.17586/2226-1494-2017-17-1-117-128
-
Sidorova M.Yu. Internet Linguistics: Russian Language. Interpersonal Communication. Moscow, 1989.ru Publ., 2006, 193 p. (in Russian)
-
Schwartz M.B.An Examination of Cross-Domain Authorship Attribution Techniques. CUNY Academic Works, 2016,32 p.
-
Overdorf R., Greenstadt R. Blogs, twitter feeds, and reddit comments: cross-domain authorship attribution. Proceedings on Privacy Enhancing Technologies, 2016, no. 3,pp. 155–171.
-
VorobevaA.A. Analizvozmozhnostiprimeneniyarazlichnykhlingvisticheskikhkharakteristikdlyaidentifikatsiiavtoraanonimnykhkorotkikhsoobshcheniivglobal'noisetiInternet. Informatsia i Kosmos, 2014, no. 1, pp. 42–46. (in Russian)
-
ZhengR., Li J., Chen H., Huang Z. A framework for authorship identification of online messages: writing-style features and classification techniques. Journal of the American Society for Information Science and Technology, 2006, vol. 57, no. 3, pp. 378–393. doi: 10.1002/asi.20316
-
Vorobeva A.A. Technique of web-user identification based on stylistic and linguistic features of online texts.Informatsia i Kosmos, 2017, no. 1, pp. 127–130. (in Russian)
-
Stamatatos E.A survey of modern authorship attribution methods. Journal of the American Society for information Science and Technology, 2009, vol. 60, no. 3, pp. 538–556. doi: 10.1002/asi.21001
-
Nugumanova A.B., Bessmertnyi I.A., Petsina P., Baiburin E.M. Semantic relations in text classification based on Bag-of-words model.Software & Systems, 2016, no. 2, pp. 89–99. (in Russian)
-
Houvardas J., Stamatatos E. N-gram feature selection for authorship identification. Lecture Notes in Computer Science, 2006, vol. 4183, pp. 77–86.
-
Gomez-Adorno H. et al. Document embeddings learned on various types of n-grams for cross-topic authorship attribution. Computing, 2018, pp. 1–16. doi: 10.1007/s00607-018-0587-8
-
Maitra P., Ghosh S., Das D. Authorship verification: an approach based on random forest. Proc. 6th Conference and Labs of the Evaluation Forum, CLEF 2015. Toulouse, France, 2015.
-
Pacheco M.L., Fernandes K., Porco A. Random forest with increased generalization: a universal background approach for authorship verification. Proc. Conference and Labs of the Evaluation Forum, CLEF 2015. Toulouse, France, 2015.
-
Vorobeva A.A. Influence of features discretization on accuracy of random forest classifier for web user identification. Proc. 20th Conf. on Open Innovations Association, FRUCT. St. Petersburg, Russia, 2017, pp. 498–504. doi: 10.23919/FRUCT.2017.8071354
-
Brownlee J. Classification Accuracy is Not Enough: More Performance Measures You Can Use. 2014. Available at: http://machinelearningmastery.com/classification-accuracy-is-not-enough-more-performance-measures-you-can-use/(accesssed 20.03.2018).
-
Fomenko V.P., Fomenko T.G. Avtorskii invariant russkikh literaturnykh tekstov. In Fomenko A.T. Novaya Khronologiya Gretsii. Moscow, MSU Publ., 1995, vol. 2. (in Russian)