Menu
Publications
2025
2024
2023
2022
2021
2020
2019
2018
2017
2016
2015
2014
2013
2012
2011
2010
2009
2008
2007
2006
2005
2004
2003
2002
2001
Editor-in-Chief
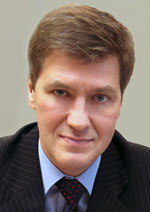
Nikiforov
Vladimir O.
D.Sc., Prof.
Partners
doi: 10.17586/2226-1494-2018-18-5-709-718
AUTOMATIC OBJECT CLASSIFICATION ACCORDING TO 3D-LIDAR DATA BASED ON SINGLE-PHOTON COUNTING TECHNOLOGY
Read the full article

Article in Russian
For citation:
Abstract
For citation:
Timofeev A.V., Denisov V.M. Automatic object classification according to 3d-lidar data based on single-photon counting technology. Scientific and Technical Journal of Information Technologies, Mechanics and Optics, 2018, vol. 18, no. 5, pp. 709–718 (in Russian). doi: 10.17586/2226-1494-2018-18-5-709-718
Abstract
Subject of Research. We compare the effectiveness of the procedure for automatic object classification according to 3d-lidar data, built on the basis of single-photon counting technology, with the efficiency of the classification procedure with the usage of the conventional linear lidar data. Lidars based on the single-photons counting technology (SPCT) significantly exceed the ordinary linear lidars for a whole range of target parameters, including: spatial resolution - by more than an order of magnitude; the performance of the land surface scanning process - by an order of magnitude; by weight and size indicators - few times less. Unlike linear lidars, each laser point in the case of a SPCT-lidar is described not only by its coordinates and the intensity of the reflected signal, but by coordinates and an additional data block that characterizes the surface relief of the probed object in the direction of the probing photon flux. The presence of this additional data block for each laser point makes it possible to consider the received images as 3d-images, that simplifies the solution of not only the photogrammetric problem, but also the task of automatic objects classification on SPCT-image. Method. We consider the automatic object classification task solution based on SPCT-data with the use of the machine learning methods (XGBoost and multilayered neural networks - ANN). Main Results. The results of the numerical simulation carried out within the framework of the present study showed that, other things being equal, the efficiency of solving the classification problem based on SPCT-data, in practically important cases, increases up to 20% compared with the case where the linear-lidar data was used. Practical Relevance. The obtained results can be used in the design of the mobile SPCT-lidars enabling the detection and classification of objects on the Earth's surface in real time with high reliability.
Keywords: Single-Photon Counting Technology, 3d-lidar, Geiger-mode lidar, single-photon lidar, XGBoost, ANN
References
References
-
Priedhorsky W.C., Smith R.C., Ho C. Laser ranging and mapping with a photon-counting detector. Applied Optics, 1996, vol. 35, no. 3, pp. 441–452. doi: 10.1364/AO.35.000441
-
Degnan J., Wells D., Machan R., Leventhal E. Second generation airborne 3D imaging lidars based on photon counting. Proc. SPIE, 2007, vol. 6771. doi: 10.1117/12.732086
-
Williams G.M. Limitations of Geiger-mode arrays for Flash LADAR applications. Proc. SPIE, 2010, vol. 7684. doi: 10.1117/12.853382
-
Aull B.F., Loomis A.H., Young D.J., Stern A., Felton B.J., Daniels P.J., Landers D.J., Retherford L., Rathman D.D., Heinrichs R.M. et al. Three-dimensional imaging with arrays of geiger-mode avalanche photodiodes. Proc. SPIE, 2004, vol. 5353, pp. 105–116. doi: 10.1117/12.532723
-
Li Q., Dengan J., Barrett T., Shan J. First evaluation on single photon-sensitive lidar data. Photogrammetric Engineering and Remote Sensing, 2016, vol. 82, no. 7, pp. 455–463. doi: 10.14358/PERS.82.7.455
-
Moussavi M.S., Abdalati W., Scambos T., Neuenschwander A. Applicability of an automatic surface detection approach to micro-pulse photon-counting lidar altimetry data: implications for canopy height retrieval from future ICESat-2 data. International Journal of Remote Sensing, 2014, vol. 35, no. 13, pp. 5263–5279. doi: 10.1080/01431161.2014.939780
-
Marino R.M., Davis W.R.Jigsaw: a foliage-penetrating 3D imaging laser. Lincoln Laboratory Journal, 2005, vol. 15, pp. 23–36.
-
Single Photon Lidar: Sigma-Space. Available at: http://www.sigmaspace.com/single-photon-lidar (accessed 10.07.2018).
-
Geiger-Mode Lidar: Raising the Standard to New Heights. Harris, 2007. Available at: www.harrisgeospatial.com/Portals/0/pdfs/HG_Geiger-mode_LiDAR_brochure_WEB.pdf (accessed 10.07.2018).
-
Chen T., Guestrin C. XGBoost: a scalable tree boosting system. Proc. 22nd ACM SIGKDD Int. Conf. on Knowledge Discovery and Data Mining. San Francisco, USA, 2016, vol. 13-17, pp. 785–794. doi: 10.1145/2939672.2939785