Menu
Publications
2024
2023
2022
2021
2020
2019
2018
2017
2016
2015
2014
2013
2012
2011
2010
2009
2008
2007
2006
2005
2004
2003
2002
2001
Editor-in-Chief
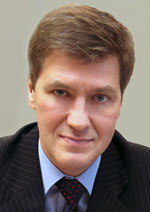
Nikiforov
Vladimir O.
D.Sc., Prof.
Partners
doi: 10.17586/2226-1494-2018-18-6-1091-1098
ANALYSIS OF NOISE COMPONENTS IN QUARTZ PENDULUM ACCELEROMETER WITH DIGITAL FEEDBACK AMPLIFIER
Read the full article

Article in Russian
For citation:
Abstract
For citation:
Deputatova E.A., Gnusarev D.S., Kalikhman D.M. Analysis of noise components in quartz pendulum accelerometer with digital feedback amplifier. Scientific and Technical Journal of Information Technologies, Mechanics and Optics , 2018, vol. 18, no. 6, pp. 1091–1098 (in Russian). doi: 10.17586/2226-1494-2018-18-6-1091-1098
Abstract
Subject of Research. The paper presents research of compensation-typequartz pendulum accelerometer with digital feedback amplifier. Noise components of the accelerometer output signal are studied. Method. Basedon a series of experimental data, the noise components and errors of the studied device are analyzed in accordance with the method adopted at a number of domestic industrial enterprises in compliance with the Russian standards, and also in accordance with the Allan variation method, which corresponds to the International standards. MainResults. We have performed the level estimation of noise components using the spectral density of noise power distribution method. The problem of discrete filter creation is solved for the output signal realized in a digital feedback amplifier based on an embedded microcontroller. The filter has been selected in accordance with two quality criteria. According to the first criterion, the root-mean-square error tends to a minimum. The second one is a complex quality criterion for which the studied device is viewed as a closed automatic control system, wherein the system bandwidth is expected to tend to the required value and the control time tends to a minimum. Mathematical simulation of operation of the accelerometer with a digital feedback amplifier and a filter is performed in the MATLAB environment in order to determine the parameters that correspond to the complex quality criterion. Practical Relevance. It is shown that the useof the second order Butterworth filter makes it possible to reduce the noise component of the accelerometer output signal by approximately 2.5 times and corresponds to both quality criteria outlined in the paper.
Keywords: accelerometer, digital feedback amplifier, noise components, errors, filter
References
References
-
Kalihman D.M., Kalihman L.Ja., Kaldymov N.A., Nahov S.F. Linear acceleration meter with precision quartz accelerometers as sensing elements. Proc. 9th St. Petersburg Int. Conf. on Integrated Navigation Systems. St. Petersburg, 2002, pp. 216–220. (in Russian)
-
Kalikhman D.M., Grebennikov V.I., Kalikhman L.Ya., Nakhov S.F.,Skorobogatov V.V., Ermakov R.V. The results of experimental testing of thermo-variant quartz pendulum accelerometer with digital feedback and reprogrammable measurement range. Proc. 13th St. Petersburg Int. Conf. on Integrated Navigation Systems. St. Petersburg, 2016, pp. 139–157. (in Russian)
-
Hou H., El-Sheimy N. Inertial sensors errors modeling using Allan variance. Proc. of ION GPS/GNSS 2003. Portland, 2003, pp. 2860–2867.
-
Allan D.W. Historicity, strengths, and weaknesses of Allan variances and their general applications. Gyroscopy and
Navigation, 2015, no. 4, pp. 3–28. (in Russian) -
Siraya T.N. Allan variances as a measurement error estimation. Gyroscopy and Navigation, 2010, no. 2, pp. 29–36. (in Russian)
-
Stepanov O.A., Chelpanov I.B., Motorin A.B. Accuracy of sensor bias estimation and its relationship with Allan variance. Gyroscopy and Navigation, 2017, vol. 8, no. 1, pp. 51–57. doi: 10.1134/S2075108717010072
-
Besekerskii V.A., Popov E.P. The Theory of Automatic Control Systems. Moscow, Nauka Publ., 1975, 768 p. (in Russian)
-
Nurminen H., Raitoharju M., Piche R. An efficient indoor positioning particle filter using a floor-plan based proposal
distribution. Proc. 19th IEEE Int. Conf. on Information Fusion, FUSION. Heidelberg, Germany, 2016, pp. 541–548. -
Piche R. Cramer-Rao lower bound for linear filtering with t-distributed measurement noise. Proc. 19th IEEE Int. Conf. on Information Fusion, FUSION. Heidelberg, Germany, 2016, pp. 536–540.
-
Raitoharju M., Piche R., Ala-Luhtala J., Ali-Loytty S. Partitioned update Kalman filter. Journal of Advances in Information Fusion, 2016, vol. 11, no. 1, pp. 3–14.
-
Sarkka S. Bayesian Filtering and Smoothing. Cambridge University Press, 2013, 252 p.
-
Stepanov O.A. Optimal and sub-optimal filtering in integrated navigation systems. In Aerospace Navigation Systems. NY, John Wiley and Sons, 2016, pp. 244–298. doi: 10.1002/9781119163060.ch8
-
Stepanov O.A. Basis of the Estimation Theory with Applications to the Tasks of Navigation Information Processing. Part 2. Introduction to the theory of filtration. St. Petersburg, Concern CSRI Elektropribor, 2017, 428 p. (in Russian)
-
D’yakonov V.P. Matlab 6.5 SP1/7 + Simulink 5/6. Application Basics. Moscow, Solon-Press, 2005, 800 p. (in Russian)
-
Znamenskii A.E., Teplyuk I.N. Active RC Filters. Moscow, Svyaz' Publ., 1970, 280 p. (in Russian).