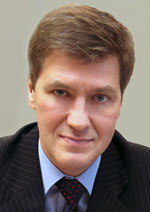
Nikiforov
Vladimir O.
D.Sc., Prof.
doi: 10.17586/2226-1494-2018-18-6-1108-1117
MAINTAINING OF INTERNAL CONSISTENCY OF ALGEBRAIC BAYESIAN NETWORKS WITH LINEAR AND STELLATE STRUCTURE
Read the full article

For citation:
Abstract
Subject of Research. When working with algebraic Bayesian networks, it is necessary to ensure their correctness in terms of the consistency of the probability estimates of their constituent elements.There are several approaches to automating the maintenance of consistency, characterized by their computational complexity (execution time). This complexity depends on the network structure and the chosen type of consistency. The time for internal consistency maintenance in algebraic Bayesian networks with linear and stellate structure is compared with the time for consistency maintenance of a knowledge pattern covering such networks. The comparison is based on statistical estimates. Method.The essence of the method lies in reducing the number of variables and conditions in linear programming problems which solution ensures the maintenance of internal consistency. An experiment was carried out demonstrating the differences between the time of consistency maintenance for different algebraic Bayesian networks with a global structure. Main Results. An improved version of the algorithm for internal consistency maintenance is presented.Solvable linear programming problems are simplified in comparison with the previous version of the algorithm. Two theorems are formulated and proved, refining the estimates of the number of variables and conditions in the linear programming problems to be solved, as well as the number of the problems themselves. An experiment is performed, which showed that the proposed software implementation of internal consistency maintenanceis superior in working time to software implementation of the consistency maintenanceof a complete knowledge pattern. Practical Relevance. The results obtained can be applied in machine learning of algebraic Bayesian networks (including the synthesis of their global structures). The proposed method provides optimal synthesis of global network structures for which it is enough to use the maintenance of internal consistency during learning and further network processing. Owing to the method application these processes will have acceptable computational complexity.
Acknowledgements. The research was carried out in the framework of the project on SPIIRAS state assignment No. 0073-2018-0001, with the financial support of the RFBR (project No. 18-01-00626 Methods of representation, synthesis of truth estimates and machine learning in algebraic Bayesian networks and related knowledge models with uncertainty: the logic-probability approach and graph systems).
References
-
Tulup'ev A.L., Nikolenko S.I., Sirotkin A.V. Bayesian Networks: Logical-Probabilistic Approach. St. Petersburg, Nauka Publ., 2006, 607 p. (in Russian)
-
Tulup'ev A.L., Sirotkin A.V., Nikolenko S.I. Bayesian Belief Networks: Logical-Probabilistic Inference in the Acyclic
Directed Graph. St. Petersburg, SPbSU Publ., 2009, 400 p. (in Russian) -
Tulup'ev A.L. Algebraic Bayesian Networks: a Local Logic-Probabilistic Inference. Tutorial. St. Petersburg, SPbGU Publ., Anatoliya Publ., 2007, 80 p. (in Russian)
-
Augustin T., Seising R. Weichselberger's contribution to impreciseprobabilities and statistical inference. International Journal of Approximate Reasoning, 2018, vol. 98, pp. 132–145. doi: 10.1016/j.ijar.2018.04.009
-
Quost B., Destercke S. Classification by pairwise coupling of imprecise probabilities. Pattern Recognition, 2018, vol. 77, pp. 412–425. doi: 10.1016/j.patcog.2017.10.019
-
Abellan J., Mantas C.J., Castellano J.G., Moral-Garcia S. Increasing diversity in random forest learning algorithm via
imprecise probabilities. Expert Systems with Applications, 2018, vol. 97, pp. 228–243. doi: 10.1016/j.eswa.2017.12.029 -
Zhang J., Shields M.D. On the quantification and efficient propagation of imprecise probabilities resulting from small
datasets. Mechanical Systems and Signal Processing, 2018, vol. 98, pp. 465–483. doi: 10.1016/j.ymssp.2017.04.042 -
Romanov A.V., Levenets D.G., Zolotin A.A., Tulupyev A.L. Incremental synthesis of the tertiary structure of algebraic Bayesian networks. Proc. 19th Int. Conf. on Soft Computing and Measurements, SCM 2016. St. Petersburg, Russia, 2016, pp. 28–30. doi: 10.1109/SCM.2016.7519673
-
Kharitonov N.A., Tulupyev A.L., Zolotin A.A. Software implementation of reconciliation algorithms in algebraic Bayesian networks. Proc. 20th Int. Conf. on Soft Computing and
Measurements, SCM 2017. St. Petersburg, Russia, 2017, pp. 8–10. -
Zolotin A.A., Tulupyev A.L. Sensitivity statistical estimates for local a posteriori inference matrix-vector equations in algebraic Bayesian networks over quantum propositions. Vestnik St. Petersburg University: Mathematics, 2018, vol. 51, no. 1, pp. 42–48. doi: 10.3103/s1063454118010168
-
Kang H.G., Lee S.H. et al. Development of a Bayesian belief network model for software reliability quantification of digital protection systems in nuclear power plants. Annals of Nuclear Energy, 2018, vol. 120, pp. 62–73. doi: 10.1016/j.anucene.2018.04.045
-
Dal F.N., Quinn C., Morari F. A Bayesian belief network framework to predict SOC dynamics of alternative management
scenarios. Soil and Tillage Research, 2018, vol. 179, pp. 114–124. doi: 10.1016/j.still.2018.01.002 -
Liu H., Kim J., Shlizerman E. Functional connectomics from neural dynamics: probabilistic graphical models for neuronal network of Caenorhabditis elegans. Philosophical Transactions of the Royal Society B: Biological Sciences, 2018, vol. 373, no. 1758. doi: 10.1098/rstb.2017.0377
-
Kang Z., Yang J. A probabilistic graphical model for the classification of mobile LiDAR point clouds. ISPRS Journal of Photogrammetry and Remote Sensing, 2018, vol. 143, pp. 108–123. doi: 10.1016/j.isprsjprs.2018.04.018
-
Tulup'ev A.L. Probabilistic estimates consistency in conjuncts and disjuncts ideals. Vestnik of the St. Petersburg University: Seriya 10: Prikladnaya Matematika. Informatika. Protsessy Upravleniya, 2009, no. 3, pp. 143–150. (in Russian)
-
Tulup'ev A.L. Bayesian Networks: Logical-Probabilistic Output in Cycles. St. Petersburg, SPbSU Publ., 2008, 140 p. (in Russian)
-
Sirotkin A.V. Algebraic bayesian networks reconciliation: computational complexity. Trudy SPIIRAN, 2010, vol. 15, pp. 162–192.
-
Dalkiran E., Ghalami L. On linear programming relaxations for solving polynomial programming problems. Computers and
OperationsResearch,2018,vol. 99,pp. 67–77. doi: 10.1016/j.cor.2018.06.010 -
Kolev L., Skalna I. Exact solution to a parametric linear programming problem. Numerical Algorithms, 2018, vol. 78, no. 4, pp. 1183–1194. doi: 10.1007/s11075-017-0418-6
-
Feng J., Che A. Novel integer linear programming models for the facility layout problem with fixed-size rectangular departments.Computers and Operations Research, 2018, vol. 95, pp. 163–171. doi: 10.1016/j.cor.2018.03.013
-
Abramov M.V. Automation of the social networks websites content analysis in the problems of forecasting the protection of the information systems users from social engineering attacks. Automation of Control Processes, 2018, no. 1, pp. 34–40. (in Russian)
-
Azarov A.A., Tulup'eva T.V., Suvorova A.V., Tulup'ev A.L., Abramov M.V., Yusupov R.M. Social Engineering Attacks. Analysis Problems. St. Petersburg, Nauka Publ., 2016, 352 p.
(in Russian)