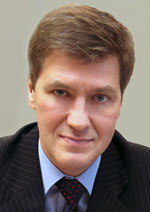
Nikiforov
Vladimir O.
D.Sc., Prof.
doi: 10.17586/2226-1494-2019-19-2-209-215
MULTI-ROTOR UNMANNED AERIAL VEHICLE CONTROL SYSTEM BASED ON HYBRID NEUROREGULATOR
Read the full article

For citation:
Abstract
Compact multirotor unmanned aerial vehicles are used to solve a variety of problems, from aerial photography to reconnaissance and goods delivery, but at present, their widespread use is constrained by the imperfection of stabilization and navigation systems. The problem can be solved by methods of neurocontrol, which are known to be an essential tool when nonlinear behavior of the craft should be taken into account. A new approach for creation of a multirotor aircraft control system is considered based on a hybrid parallel neurocontroller that uses an artificial neural network of radial basis functions. Parallel neurocontrol provides for the simultaneous use of both the conventional proportional-integral-derivative controller and the neurocontroller in the control loop, their outputs being combined. In order to optimize the calculations performed during the second layer of the neurocontroller network training, a piecewise linear function of N segments, symmetric about zero, was chosen as the function for activating the neurons of the second layer. A low-cost method is proposed for parameter optimization of the activation functions of the hidden layer neurons. It allows for network training in real time. The proposed hybrid parallel neurocontroller is implemented and studied based on an artificial neural network of radial basis functions. Bench tests have shown that the stabilization of the three axes of the hybrid neurocontroller provides quality control better than a conventional manually tuned proportional-integral-derivative controller, namely, reducing the transition time, lower amplitude oscillations in the stabilization of the unmanned aerial vehicle and increase the stability of the craft to external influences. The use of hybrid parallel neurocontroller will help to solve the problems of maneuvering and stabilization of the craft for the transition to the local navigation problem solution.
References
-
Bobtsov A.A., Pyrkin A.A. Adaptive and Robust Control with Uncertainties Compensation. St. Petersburg, NRU ITMO, 2013, 135 p. (in Russian)
-
Salichon M., Tumer K. A neuro-evolutionary approach to micro aerial vehicle control. Proc. 12th Annual Genetic and Evolutionary Computation Conference,GECCO’10. Portland, USA, 2010, pp. 1123–1130. doi: 10.1145/1830483.1830692
-
Shepherd III J.F., Tumer K. Robust neuro-control for a micro quadrotor. Proc. 12th Annual Genetic and Evolutionary Computation Conference,GECCO’10. Portland, USA, 2010, pp. 1131–1138. doi: 10.1145/1830483.1830693
-
Lendaris G.G. A retrospective on adaptive dynamic programming for control. Proc. Int. Joint Conference on Neural Networks. Atlanta, USA, 2009, pp. 1750–1757. doi: 10.1109/ijcnn.2009.5178716
-
Anuradha D.B., Prabhaker G., Murthy J.S.N. Direct inverse neural network control of a continuous stirred tank reactor. Proc. Int. MultiConference of Engineers and Computer Scientists, 2009, vol. II.
-
Zareh S.H., Abbasi M., Mahdavi H., Osgouie K.G. Semi-active vibration control of an eleven degrees of freedom suspension system using neuro inverse model of magnet or heological dampers. Journal of Mechanical Science and Technology, 2012, vol. 26, no. 8, pp. 2459–2467. doi: 10.1007/s12206-012-0628-8
-
Ni Z., Fang X., He H., Xu X. Real-time tracking on adaptive critic design with uniformly ultimately bounded condition. Proc. 2013 IEEE Symposium on Adaptive Dynamic Programming and Reinforcement Learning. Singapore,2013. doi: 10.1109/adprl.2013.6614987
-
Shafiekhani A., Mahjoob M.J., Akraminia M. Design and implementation of an adaptive critic-based neuro-fuzzy controller on an unmanned bicycle. Mechatronics, 2015, vol. 28, pp. 115–123. doi: 10.1016/j.mechatronics.2015.04.010
-
Mohammadzaheri M., Ghanbari M., Mirsepahi A., Behnia-Willson F. Efficient neuro-predictive control of a chemical plant. Proc. 5th Symposium on Advances in Science & Technology. Iran, 2011.
-
Nguen H.G., Shin J., Kim W. A study on autotuning controller for servo system. Intelligent Control and Automation, 2014, vol. 5, no. 3, pp. 102–110. doi: 10.4236/ica.2014.53012
-
Omatu S., Yoshioka M., Fujinaka T. Neuro-PID control for electric vehicle. Journal of Advanced Computational Intelligence and Intelligent Informatics, 2011, vol. 15, no. 7, pp. 846–852. doi: 10.20965/jaciii.2011.p0846
-
Zeng S., Hu H., Xu L., Li G. Nonlinear adaptive PID control for greenhouse environment based on RBF network. Sensors, 2012, vol. 12, no. 5, pp. 5328–5348. doi: 10.3390/s120505328
-
Stuart M., Manic M. Survey of progress in deep neural networks for resource-constrained applications. Proc. IECON 2017 – 43rd Annual Conference of the IEEE Industrial Electronics Society. doi: 10.1109/IECON.2017.8217271
-
Sak H., Senior A.W., Beaufays F. Long short-term memory recurrent neural network architectures for large scale acoustic modeling. Proc. INTERSPEECH 2014. Singapore, 2014, pp. 338–342.
-
Belyaev S.S., Budko M.B., Budko M.Y., Guirik A.V., Zhigulin G.P. Functional design of flight and navigation controller unit for multirotor unmanned aerial vehicle. Radiopromyshlennost', 2015, no. 4, pp. 77–87. (in Russian)