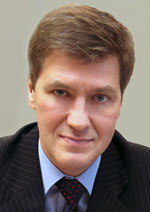
Nikiforov
Vladimir O.
D.Sc., Prof.
doi: 10.17586/2226-1494-2019-19-4-704-713
PATTERN RECOGNITION IN EXPERT DECISION-MAKING SYSTEMS
Read the full article

For citation:
Abstract
The paper presents a review of pattern recognition methods in various expert decision-making systems. In particular, the methods of visual images recognition are studied. The relevance of the proposed study is that in almost all spheres of life and production computer processes huge amounts of information at high speed, using certain algorithms, but people should anyway participate in decision-making. Pattern recognition in various processes gives the possibility to achieve maximum production results. Implementation of neural networks into production processes provides for maximum production optimization. The main task for artificial intelligence is to create certain information systems with an effective level of solutions for various non-standard tasks. Considering that, neural networks make it possible to recognize images in different decision-making systems. The subject of research is a review of pattern recognition methods in expert decision-making systems. The ability of pattern recognition in the considered systems is shown. It is demonstrated that the variety and complexity of recognition tasks do not provide implementation of one universal approach to the solution. The paper proposes modified classification of pattern recognition methods and implementation of neural networks into production process of Kamaz Public Company.
Acknowledgements. The author expresses personal gratitude to Professor Boris S. Padun
References
-
Goze E., Johnsonbaugh R., Jost S. Pattern Recognition & Image Analysis. PrenticeHall, 1996,483 p.
-
GritsanovA.A. (ed.) The Newest Philosophical Dictionary. Postmodernism. Minsk, Sovremennyi Literator Publ., 2007. 816 p.
-
Automated Image Processing of Natural Complexes of Siberia. Eds. A.L. Yanshin, V.A. Solov’ev. Novosibirsk, NaukaPubl., 1988, 226 p. (inRussian)
-
Oganezov A. Neuro-matrix object recognition model. Bulletin of the Georgian National Academy of Sciences, 2006, vol. 173, no. 3.
-
Kochladze Z.Yu., Oganezov A.L. About one possible approach to the problem of recognition of flat figures. Proceedings of Tbilisi State University, 2005, no. 364, pp. 149–155.
-
Chaban L.N. Theory and Algorithms of Pattern Recognition. Moscow, MIIGAIK Publ., 2004, 70 p. (in Russian)
-
Jain A.K., Dubes R.C. Algorithms for Clustering Data. Prentice-Hall, 1988, 334 p.
-
ChernukhinYu.V. Neuroprocessors. Taganrog, TRTUPubl., 1994, 175 p. (in Russian)
-
Giarratano J.C., Riley G.D. Expert Systems: Principles and Programming. 4th ed. Thomson, 2005.
-
Yablonskii S.V. Introduction to Discrete Mathematics. Moscow, Nauka Publ., 1986, 384 p. (in Russian)
-
Hähnle R. Automated theorem proving in multiple-valued logics. Proc. ISMIS’93.
-
NovikovaN.M.Structural Pattern Recognition. Voronezh, VSUPubl., 2006, 29 p. (inRussian)
-
Szegedy C., Toshev A., Erhan D. Deep neural networks for object detection. In Adv. in Neural Information Processing Systems, 2013.
-
SannerS. Rowley-Baluja-KanadeFaceDetector. Available at: http://users.cecs.anu.edu.au/~ssanner/Software/Vision/Project.html#I (accessed: 12.06.2019).
-
Thai B., Healey G. Invariant subpixel material detection in hiperspectral imagery. IEEE Transaction of Geoscience and Remote Sensing, 2002, vol. 40, no. 3, pp. 599–608. doi: 10.1109/tgrs.2002.1000320
-
Patrick E.A. Fundamentals of Pattern Recognition. Englewood Cliffs, Prentice-Hall, 1972.
-
Tsai F., Philpot W.D. A derivative-aided hiperspecrtal image analysis system for land-cover classification. IEEE Transaction of Geoscience and Remote Sensing, 2002, vol. 40, no. 2, pp. 416–425. doi: 10.1109/36.992805
-
Tsygankov V.D. Neurocomputer and Its Application. Moscow, SolSistem Publ., 1993, 117 p. (in Russian)
-
Wasserman P.D. Neural Computing. Theory and Practice. New York, Van Nostrand Reinhold Co, 1989, 230 p.
-
Jannson Т., Karagaleff C., Stoll K.M. Photo-refractive LiNbO3 as a storage medium for high-density optical neural networks. Optical Society of America Annual Meeting, 1986.
-
Trakhtengerts E.A. Computer Decision Support. Moscow, Sinteg Publ., 1998, 376 p. (in Russian)
-
Kohonen T. The self-organizing map. Proceedings of the IEEE, 1990, vol. 78, no. 9, pp. 1464–1480. doi: 10.1109/5.58325
-
Kohonen T. Learning vector quantization. In Abib M.A. (ed.), The Handbook of Brain Theory and Neural Networks. Cambridge, MIT Press, 1995, pp. 537–540.
-
Arsiry E.A., Sayenko A.A. Neural network pattern recognition of the public library readers to provide specialized information services. Trudy Odesskogo Politekhnicheskogo Universiteta, 2011, no. 1, pp. 118–124. (in Russian)
-
Wang H., Raj B. On the Origin of Deep Learning. ArXiv:1702.07800v4, 2017.
-
Kashkin V.B., Sukhinin A.I.Remote Sensing of the Earth from Space. Digital Image Processing. Moscow, Logos Publ., 2001, 264 p. (in Russian)
-
Gorelik A.L., Skripkin V.A. Recognition Methods. Moscow, Vysshaya Shkola Publ., 1977, 222 p.
-
Duran B.S., Odell P.L. Cluster Analysis. Springer, 1974, 146 p.
-
Burr D.J. Experiments with a connectionist text reader. Proc. 1st Int. Conf. on Neural Networks. San Diego, 1987, vol. 4, pp. 717–724.
-
Mirzoyan A.S. Development of systems for recognition and positioning of aircraft and ground objects based on computational geometry methods. Dis. PhD Eng. Sci. St. Petersburg, 2017, 150 p. (in Russian)
-
KozynchenkoV.A., PrusA.I. Neural network in approximation problems. St. Petersburg, 2014. (in Russian)
-
Statistica Neural Networks. Moscow, Goryachaya Liniya-Telekom Publ., 2000, 182 p. (inRussian)
-
PrasolovV.V., TikhomirovV.M. Geometry. Moscow, MTsNMOPubl., 2007, 328 p. (inRussian)
-
RojasR. NeuralNetworks. A Systematic Introduction. Springer-Verlag, 1996,512 p.
-
S. Neural Networks. Macmillan, 1994.
-
TuT.M., ChenA.H., WuJ.L., ChangC. Afasttwo-stage classification method for high-dimensional remote sensing data. IEEE Transaction of Geoscience and Remote Sensing, 1998, vol. 36, no. 1, pp. 182–191. doi: 10.1109/36.655328
-
Gallant S.I. Connectionist expert system. Communications of the ACM, 1998, vol. 31, no. 2, pp. 152–169. doi: 10.1145/42372.42377
-
Hebb D.O. Organization of Behavior. New York, Wiley, 1991.
-
Sejnowski T.J., Rosenberg C.R. Parallel networks that learn to pronounce English text. Complex Systems, 1987, vol. 3, pp. 145–168.
-
Ionov S.D. Distributed launch of neural networks on a set of computing nodes.Vestnik UGATU, 2013, vol. 17, no. 2, pp. 85–91. (in Russian)
-
Zenin A.V. Research possibilities of neural networks using. Young Scientist, 2017, no. 16, pp. 125–130. (in Russian)
-
Ionov S.D. Distributed streaming neural network. Proc. 42nd All-Russian Conf. on Modern Problems of Mathematics, 2011, pp. 288–290. (in Russian)
-
Voit J. The Statistical Mechanics of Financial Markets. Springer, 2003. doi: 10.1007/978-3-662-05125-2
-
Weston J.F., Copeland T.E. Financial Theory and Corporate Policy. 3rd ed. Addison Wesley, 1988.
-
Arnol'd V.I. Catastrophe Theory. 3rd ed. Berlin: Springer-Verlag, 1992.
-
Willenbrock H. Secrets of decision making. GEO, 2009, no. 7, pp. 70–87. (in Russian)
-
Maeterlinck M. The Life of Termites. 1926.
-
Adya M., Collopy F. How effective are neural networks at forecasting and prediction? A review and evaluation. Journal of Forecasting, 1998, vol. 17, no.56, pp. 481–495.
-
Andrighetto G., Rome I.C., Verhagen H. Social Networks and MultiAgent Systems Symposium (SNAMAS-09) Introduction. Proceedings of the SNAMAS Symposium. Edinburgh, 2009, pp. 1–3.