Menu
Publications
2025
2024
2023
2022
2021
2020
2019
2018
2017
2016
2015
2014
2013
2012
2011
2010
2009
2008
2007
2006
2005
2004
2003
2002
2001
Editor-in-Chief
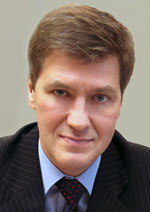
Nikiforov
Vladimir O.
D.Sc., Prof.
Partners
doi: 10.17586/2226-1494-2019-19-6-1094-1105
IDENTIFICATION OF EQUIPMENT DEGRADATION PHASE IN PREVENTATIVE MAINTENANCE SYSTEMS
Read the full article

Article in Russian
For citation:
Abstract
For citation:
Timofeev A.V., Denisov V.M. Identification of equipment degradation phase in preventative maintenance systems. Scientific and Technical Journal of Information Technologies, Mechanics and Optics, 2019, vol. 19, no. 6, pp. 1094–1105 (in Russian). doi: 10.17586/2226-1494-2019-19-6-1094-1105
Abstract
Subject of Research. The paper proposes a novel organization technique for preventive maintenance systems (including condition-based and predictive maintenance systems) based on the use of modern machine learning methods. The systems are operating using an original, non-parametric identification method for the current degradation phase of serviced equipment. Method. The proposed method comprises reducing the task of the current phase identification of the equipment degradation phase to interval estimation of the value of the so-called “health index” parameter of the equipment. This parameter is represented as a step function with the arguments in terms of a set of the measurable equipment objective parameters. The current equipment degradation phase is determined by classification approach. At this, based on the analysis of the observed data, it is decided upon what class (state phase) these data correspond to. Measurements from a group of sensors, in general, of various physical nature, which are located both on the surface and inside the equipment being monitored are used as data for identification of the equipment degradation stage. Mathematically, the proposed approach is reduced to a weighted combination of two classifiers. One of the classifiers of this combination is based on solving a group of binary classification problems. The second classifier is based on “Remaining Useful Life” parameter estimation by the method of nonparametric regression. Main Results. As distinguished from traditional approaches, the proposed approach uses a minimum of a priori information about the principles of operation and the internal structure of the equipment being serviced. The approach is based on the usage of the “health index” equipment parameter presented in the form of a step function. The novelty of the approach lies in the simultaneous use of the “health index” step function and the weighted combination of two classifiers with various structure. The proposed method showed good results when being tested on the C-MAPPS Dataset database, which contains data on failures of turbofan engines modeled using a thermodynamic simulation model. The pre-failure status of the equipment is identified with the probability of 99%. Practical Relevance. The obtained results and algorithms can be used in preventive maintenance systems aimed at reliable identification of the equipment degradation current stage.
Keywords: predictive maintenance, condition-based maintenance, machine learning, ML PdM, XGBoost, SVM-regression
References
References
- Peng Y., Dong M., Zuo M.-J. Current status of machine prognostics in condition-based maintenance: a review. International Journal of Advanced Manufacturing Technology, 2010, vol. 50, no. 1-4, pp. 297–313. doi: 10.1007/s00170-009-2482-0
- Grall A., Dieulle L., Berenguer C., Roussignol M. Continuous-time predictive-maintenance scheduling for a deteriorating system. IEEE Transactions on Reliability, 2002, vol. 51, no. 2, pp. 141–150. doi: 10.1109/TR.2002.1011518
- Ramasso E. Investigating computational geometry for failure prognostics. International Journal of Prognostics and Health Management, 2014, vol. 5, no. 1, pp. 005.
- Fumeo E., Oneto L., Anguita D. Condition based maintenance in railway transportation systems based on big data streaming analysis. Procedia Computer Science, 2015, vol. 53, pp. 437–446. doi: 10.1016/j.procs.2015.07.321
- Li Y., Billington S., Zhang C., Kurfess T., Danyluk S., Liang S. Adaptive prognostics for rolling element bearing condition. Mechanical Systems and Signal Processing, 1999, vol. 13, no. 1, pp. 103–113. doi: 10.1006/mssp.1998.0183
- Frelicot C. A fuzzy-based prognostic adaptive system. Journal Europeen des Systemes Automatises, 1996, vol. 30, no. 2-3, pp. 281–299.
- Kothamasu R., Huang S.H., VerDuin W.H. System health monitoring and prognostics – a review of current paradigms and practices. International Journal of Advanced Manufacturing Technology, 2006, vol. 28, no. 9, pp. 1012–1024. doi: 10.1007/s00170-004-2131-6
- Goebel K., Saha B., Saxena A., Celaya J.R., Christophersen J.P. Prognostics in battery health management. IEEE Instrumentation and Measurement Magazine, 2008. vol. 11, no. 4, pp. 33–40. doi: 10.1109/MIM.2008.4579269
- Dong M., He D., Banerjee P., Keller J. Equipment health diagnosis and prognosis using hidden semi-Markov models. International Journal of Advanced Manufacturing Technology, 2006, vol. 30, no. 7-8, pp. 738–749. doi: 10.1007/s00170-005-0111-0
- Wang W.Q., Golnaraghi M.F., Ismail F. Prognosis of machine health condition using neuro-fuzzy systems. Mechanical Systems and Signal Processing, 2004, vol. 18, no. 4, pp. 813–831. doi: 10.1016/S0888-3270(03)00079-7
- Shetty P., Mylaraswamy D., Ekambaram T. A hybrid prognostic model formulation and health estimation of auxiliary power units. Journal of Engineering for Gas Turbines and Power, 2008, vol. 130, no. 2, pp. 021601. doi: 10.1115/1.2795761
- Lee J.-M., Yoo C., Lee I.-B. On-line batch process monitoring using a consecutively updated multiway principal component analysis model. Computers and Chemical Engineering, 2003, vol. 27, no. 12, pp. 1903–1912. doi: 10.1016/S0098-1354(03)00151-0
- Garga A.K., McClintic K.T., Campbell R.L., Yang C.-C., Lebold M.S., Hay T.A. Byington CS. Hybrid reasoning for prognostic learning in CBM systems. IEEE Aerospace Conference Proceedings, 2001, vol. 6, pp. 2957–2969. doi: 10.1109/AERO.2001.931316
- Ramasso E. Investigating computational geometry for failure prognostics in presence of imprecise health indicator: Results and comparisons on C-MAPSS datasets. Proc. 2nd European Conference of the PHM Society 2014 (PHME14). 2014, vol. 5.
- Medjaher K., Zerhouni N., Baklouti J. Data-driven prognostics based on health indicator construction: Application to pronostia's data. Proc. 12th European Control Conference (ECC 2013), 2013, pp. 1451–1456.
- Susto G.A., Schirru A., Pampuri S., McLoone S., Beghi A. Machine learning for predictive maintenance: A multiple classifier approach. IEEE Transactions on Industrial Informatics, 2015, vol. 11, no. 3, pp. 812–820. doi:10.1109/TII.2014.2349359
- Summary of the 2018 Department of Defense Artificial Intelligence Strategy. Harnessing AI to Advance Our Security and Prosperity. Available at: https://media.defense.gov/2019/Feb/12/2002088963/-1/-1/1/SUMMARY-OF-DOD-AI-STRATEGY.PDF (accessed: 18.10.2019).
- Kohavi R. A study of cross-validation and bootstrap for accuracy estimation and model selection. Proceedings of the Fourteenth International Joint Conference on Artificial Intelligence (IJCAI), 1995, vol. 2(12), pp. 1137–1143.
- Chen T., Guestrin C. XGBoost: A scalable tree boosting system. Proc. 22nd ACM SIGKDD International Conference on Knowledge Discovery and Data Mining, San Francisco, CA, USA, August 13-17, 2016, pp. 785–794. doi:10.1145/2939672.2939785
- Mason L., Baxter J., Bartlett P.L., Frean M. Boosting algorithms as gradient descent. Proc. 13th Annual Neural Information Processing Systems Conference, 1999, P. 512–518.
- Li Q., Racine J.S. Nonparametric Econometrics: Theory and Practice. Princeton University Press, 2007, 768 p.
- Zemel R.S., Pitassi T. A gradient-based boosting algorithm for regression problems. Advances in Neural Information Processing Systems, 2001, pp. 696–702.
- Smola A.J., Schölkopf B. A tutorial on support vector regression. Statistics and Computing, 2004, vol. 14, no. 3, pp. 199–222. doi: 10.1023/B:STCO.0000035301.49549.88
- Timofeev A.V. Parametric optimization of the multimodal decision-level fusion scheme in automatic biometric person’s identification systems. Scientific and Technical Journal of Information Technologies, Mechanics and Optics, 2014, vol. 14, no. 3(91), pp. 96–102. (in Russian)
- Saxena A., Goebel K. Turbofan Engine Degradation Simulation Data Set. Available at: https://ti.arc.nasa.gov/tech/dash/groups/pcoe/prognostic-data-repository (accessed: 14.06.2018).
- Saxena A., Goebel K., Simon D., Eklund N. Damage propagation modeling for aircraft engine run-to-failure simulation. Proc. International Conference on Prognostics and Health Management (PHM 2008), 2008, pp. 22–34. doi: 10.1109/PHM.2008.4711414
- Al-Salah T.H., Zein-Sabatto S., Bodruzzaman M. Decision fusion software system for turbine engine fault diagnostics. Proc. IEEE SoutheastCon, (SOUTHEASTCON 2012), 2012, pp. 1–6. doi: 10.1109/SECon.2012.6197000
- Coble J., Hines J.W. Applying the general path model to estimation of remaining useful life. International Journal of Prognostics and Health Management, 2011, vol. 2, pp. 1–13.
- Hu C., Youn B., Wang P., Taek Yoon J. Ensemble of data-driven prognostic algorithms for robust prediction of remaining useful life. Reliability Engineering and System Safety, 2012, vol. 103, pp. 120–135. doi: 10.1016/j.ress.2012.03.008
- Jianzhong S., Hongfu Z., Haibin Y., Pecht M. Study of ensemble learning-based fusion prognostics. Proc. Prognostics and System Health Management Conference, PHM'10, 2010, pp. 5414582. doi: 10.1109/PHM.2010.5414582
- Liu K., Gebraeel N.Z., Shi J. A data-level fusion model for developing composite health indices for degradation modeling and prognostic analysis. IEEE Transactions on Automation Science and Engineering, 2013, vol. 10, no. 3, pp. 652–664. doi: 10.1109/TASE.2013.2250282
- Pitz K., Anderl R. Implementing clustering and classification approaches for big data with MATLAB. Advances in Intelligent Systems and Computing, 2019, vol. 880, pp. 458–480. doi: 10.1007/978-3-030-02686-8_35
- Peng H.C., Long F., Ding C. Feature selection based on mutual information: criteria of max-dependency, max-relevance, and min-redundancy. IEEE Transactions on Pattern Analysis and Machine Intelligence, 2005, vol. 27, no. 8, pp. 1226–1238. doi: 10.1109/TPAMI.2005.159