Menu
Publications
2025
2024
2023
2022
2021
2020
2019
2018
2017
2016
2015
2014
2013
2012
2011
2010
2009
2008
2007
2006
2005
2004
2003
2002
2001
Editor-in-Chief
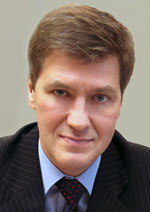
Nikiforov
Vladimir O.
D.Sc., Prof.
Partners
doi: 10.17586/2226-1494-2020-20-2-301-305
NEURAL NETWORK APPLICATION FOR DETECTION OF ROAD ACCIDENTS
Read the full article

Article in Russian
For citation:
Abstract
For citation:
Zikratova T.V., Zikratov I.A. Neural network application for detection of road accidents. Scientific and Technical Journal of Information Technologies, Mechanics and Optics, 2020, vol. 20, no. 2, pp. 301–305 (in Russian). doi: 10.17586/2226-1494-2020-20-2-301-305
Abstract
Subject of Research. The paper considers the issues of neural network application for detection and prediction of road accidents. The overtaking process of cars with crossing into oncoming traffic is analyzed. The potential possibility of road accident reduction while overtaking is shown owing to intellectual assessment of road situation dynamics development. Method. We proposed to use a two-class classifier based on a neural network. Road situations while overtaking with crossing into oncoming traffic were the objects of classification. The data on them was transmitted to the neural network input in the form of the frame set, that is, a graphical representation of discrete states of the “group of vehicles — section of the road” system. Frame formation was expected to be carried out as a result of information exchange between detectors and vehicle-mounted sensors, and road infrastructure, which is developed within the framework of the “smart city” paradigm. Main Results. The road situation is classified as “Dangerous” in case of high vehicle collision probability while overtaking and “Safe”, otherwise. If the situation is considered as “Dangerous”, the vehicle central processor generates an appropriate effect on the vehicle control elements to prevent an accident. Results of situation simulation implemented on Tensor Flow open software library for machine learning are obtained. They showed high prediction accuracy (0.96) on artificial data set. Practical Relevance. The results of the work can be used in promising unmanned and manned vehicles having radio communication with road infrastructure elements within the “smart city” concept to prevent road accidents caused by dangerous overtaking.
Keywords: prediction, detection, simulation, Bayesian classifier, neural networks, safety, unmanned vehicles
References
References
-
Carlino D., Boyles S.D., Stone P. Auction-based autonomous intersection management. Proc. 16th International IEEE Intelligent Transportation Systems: Intelligent Transportation Systems for All Modes (ITSC 2013), 2013, pp. 529–534. doi: 10.1109/ITSC.2013.6728285
-
Ho C., Reed N., Spence C. Multisensory in-car warning signals for collision avoidance. Human Factors: The Journal of the Human Factors and Ergonomics Society, 2007, vol. 49, no. 6, pp. 1107–1114. doi: 10.1518/001872007X249965
-
Au T., Zhang S., Stone P. Autonomous intersection management for semi-autonomous vehicles. The Routledge Handbook of Transportation, Routledge, Taylor&Francis Group, 2016, pp. 88–104.
-
Zohdy I.H., Kamalanathsharma R.K., Rakha H. Intersection management for autonomous vehicles using iCACC. Proc. 15th International IEEE Conference on Intelligent Transportation Systems (ITSC 2012), 2012, pp. 1109–1114. doi: 10.1109/ITSC.2012.6338827
-
Hallé S., Chaib-draa B. A collaborative driving system based on multiagent modelling and simulations. Transportation Research Part C: Emerging Technologies, 2005, vol. 13, no. 4, pp. 320–345. doi: 10.1016/j.trc.2005.07.004
-
Cremer M., Ludwig J. A fast simulation model for traffic flow on the basis of Boolean operations. Mathematics and Computers in Simulation, 1986, vol. 28, no. 4, pp. 297–303. doi: 10.1016/0378-4754(86)90051-0
-
Alvarez I., Poznyak A., Malo A. Urban traffic control problem via a game theory application. Proc. 46th IEEE Conference on Decision and Control (CDC 2007), 2007, pp. 2957–2961. doi: 10.1109/CDC.2007.4434820
-
Zikratov I.A., Viksnin I.I., Zikratova T.V. Optimization of dangerous section passage for unmanned vehicles. Scientific and Technical Journal of Information Technologies, Mechanics and Optics, 2019, vol. 19, no. 1, pp. 155–165. (in Russian). doi: 10.17586/2226-1494-2019-19-1-155-165
-
Yuzaeva A.G., Kukartsev V.V. Unmanned cars: the risk and prospects. Aerospace Topical Issues, 2016, vol. 2, no. 12, pp. 120–122. (in Russian)
-
Dai J., Li Y., He K., Sun J. R-FCN: Object detection via region-based fully convolutional networks. Proc. 30th Annual Conference on Neural Information Processing Systems (NIPS 2016), 2016, pp. 379–387.
-
Redmon J., Divvala S., Girshick R., Farhadi A. You only look once: Unified, real-time object detection. Proc. 29th IEEE Conference on Computer Vision and Pattern Recognition (CVPR 2016), 2016, pp. 779–788. doi: 10.1109/CVPR.2016.91
-
Ahmadinurov M.M., Zavalishchin D.S., Timofeeva G.A. Mathematical models for traffic management. Ekaterinburg, USURT Publ., 2011, 120 p. (in Russian)
-
Zikratov I.A., Viksnin I.I., Zikratova T.V. Multiagent planning of intersection passage by autonomous vehicles. Scientific and Technical Journal of Information Technologies, Mechanics and Optics, 2016, vol. 16, no. 5, pp. 839–849. (in Russian). doi: 10.17586/2226-1494-2016-16-5-839-849
-
Witten I.H., Frank E., Hall M.A. Data Mining: Practical Machine Learning Tools and Techniques. 3rd ed. Elsevier, 2011, 664 p.