Menu
Publications
2025
2024
2023
2022
2021
2020
2019
2018
2017
2016
2015
2014
2013
2012
2011
2010
2009
2008
2007
2006
2005
2004
2003
2002
2001
Editor-in-Chief
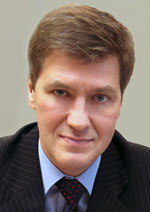
Nikiforov
Vladimir O.
D.Sc., Prof.
Partners
doi: 10.17586/2226-1494-2020-20-3-425-431
U-NET ARCHITECTURE NEURAL NETWORK FOR LOCALIZATION OF DIGITAL IMAGES INTEGRITY VIOLATION
Read the full article

Article in Russian
For citation:
Abstract
For citation:
Abdullina K.M., Spivak A.I. U-Net architecture neural network for localization of digital images integrity violation. Scientific and Technical Journal of Information Technologies, Mechanics and Optics, 2020, vol. 20, no. 3, pp. 425–431 (in Russian). doi: 10.17586/2226-1494-2020-20-3-425-431
Abstract
Subject of Research. The paper presents the study of the U-Net architecture neural network applicability to localization problem of image modifications. The implemented method provides the detecting of the modified image and getting a mask of the changed area. Method. The proposed method was based on deep machine learning – a neural network. U-Net neural network architecture was studied. The training dataset was created as a basis for model training with original images and images modified using a graphical editor. The implemented method represents image as a set of pixels. Main Results. The trained model has shown a high level of brightness recognition for image modifications, up to 80 %, and up to 64 % for copy-shift. Practical Relevance. The result can be practically applicable in the forensics for recognition of modified image blocks and for copyright protection.
Keywords: images, modification, neural networks, U-Net, information security, integrity violation
References
References
1. Serova A.I., Spivak A.I. Application of maсhine learning methods for detecting of JPEG image integrity violations. Scientific and Technical Journal of Information Technologies, Mechanics and Optics, 2018, vol. 18, no. 2, pp. 299–306. (in Russian). doi: 10.17586/2226-1494-2018-18-2-299-306
2. Al-Qershi O.-M., Khoo B.E. Passive detection of copy-move forgery in digital images: State-of-the-art. Forensic Science International, 2013, vol. 231, no. 1-3, pp. 284–295. doi: 10.1016/j.forsciint.2013.05.027
3. Amerini I., Ballan L., Caldelli R., Del Bimbo A., Serra G. A SIFT-based forensic method for copy-move attack detection and transformation recovery. IEEE Transactions on Information Forensics and Security, 2011, vol. 6, no. 3, pp. 1099–1110. doi: 10.1109/TIFS.2011.2129512
4. Cozzolino D., Poggi G., Verdoliva L. Efficient dense-field copy-move forgery detection. IEEE Transactions on Information Forensics and Security, 2015, vol. 10, no. 11, pp. 2284–2297. doi: 10.1109/TIFS.2015.2455334
5. Bravo-Solorio S., Nandi A.K. Automated detection and localisation of duplicated regions affected by reflection, rotation and scaling in image forensics. Signal Processing, 2011, vol. 91, no. 8, pp. 1759–1770. doi: 10.1016/j.sigpro.2011.01.022
6. Verdoliva L., Cozzolino D., Poggi G. A feature-based approach for image tampering detection and localization. Proc. IEEE International Workshop on Information Forensics and Security (WIFS), 2014, pp. 149–154. doi: 10.1109/WIFS.2014.7084319
7. Healey G., Kondepudy R. Radiometric CCD camera calibration and noise estimation. IEEE Transactions on Pattern Analysis and Machine Intelligence, 1994, vol. 16, no. 3, pp. 267–276. doi: 10.1109/34.276126
8. LeCun Y., Bottou L., Bengio Y., Haffner P. Gradient-based learning applied to document recognition. Proceedings of the IEEE, 1998, vol. 86, no. 11, pp. 2278–2324. doi: 10.1109/5.726791
9. Ronneberger O., Fischer Ph., Brox T. U-Net: Convolutional networks for biomedical image segmentation. Lecture Notes in Computer Science (including subseries Lecture Notes in Artificial Intelligence and Lecture Notes in Bioinformatics), 2015, vol. 9351, p. 234–241. doi: 10.1007/978-3-319-24574-4_28
10. Kingma D.P., Ba J.L. Adam: A method for stochastic optimization. Proc. 3rd International Conference on Learning Representations (ICLR 2015), 2015, pp. 149801
11. Rezatofighi H., Tsoi N., Gwak J., Sadeghian A., Reid I., Savarese S. Generalized intersection over union: A metric and a loss for bounding box regression. Proc. 32nd IEEE/CVF Conference on Computer Vision and Pattern Recognition (CVPR), 2019, pp. 658–666. doi: 10.1109/CVPR.2019.00075