Menu
Publications
2025
2024
2023
2022
2021
2020
2019
2018
2017
2016
2015
2014
2013
2012
2011
2010
2009
2008
2007
2006
2005
2004
2003
2002
2001
Editor-in-Chief
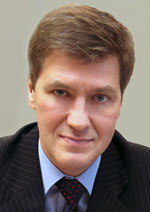
Nikiforov
Vladimir O.
D.Sc., Prof.
Partners
doi: 10.17586/2226-1494-2020-20-5-649-660
SEARCH QUALITY METHODOLOGY AND PARTICULAR FINDINGS FOR KEY POINTS BASED ON MATERIALS OF OPTICAL-ELECTRONIC AERIAL SURVEY
Read the full article

Article in Russian
For citation:
Abstract
For citation:
Altukhov A.I., Bilan V.I., Grigor’ev A.N., Popovich V.V. Search quality methodology and particular findings for key points based on materials of optical-electronic aerial survey. Scientific and Technical Journal of Information Technologies, Mechanics and Optics, 2020, vol. 20, no. 5, pp. 649–660 (in Russian). doi: 10.17586/2226-1494-2020-20-5-649-660
Abstract
Subject of Research. This paper presents the findings of the Scale-Invariant Feature Transform method for key points search. The method is used for the problems of photogrammetric processing of terrain images obtained from aircrafts and satellites. Method. The chosen method is widely used for spatial linking of images, tracking of changes and searching for objects, building of digital models and terrain orthophotoplans. The relevance of the Scale-Invariant Feature Transform method analysis lies in the fact that it was originally developed as a universal method for image processing in the field of technical vision. The existing modifications of this method, specialized for processing of terrain images, are applied in practice to a limited extent and have been studied without complete account of the image properties. In particular, the existing studies do not take into account the effect of the depicted plot on the key points search quality, which, in the general case, is characterized by a random combination of terrain objects. It is assumed that the plot features on the terrain image can cause significant variations in the distribution of the selected points in the image of a separate exposure when applying of the key points search method. To determine the dependence of the search quality for key points on the depicted plot, it is necessary to develop a methodology based on the features analysis of the Scale-Invariant Feature Transform method implementation and the use of a reference image set with various plot composition. As a content analysis result of the Scale-Invariant Feature Transform method, the criteria and rejection parameters of the determined key points are defined. The approach to the analysis of the image plot effect on the key points quality is based on the set of images classified by the characteristics of the plots on homogeneous and heterogeneous images. According to the proposed technique, the analysis is performed on the basis of the statistical and spatial distributions of key points obtained from individual images and their aggregates. Main Results. The research proposes a methodology for the dependence of the quality of the key points search result on the plot in the image. As a result of the experiment, factors are identified that cause a uniformity violation of the key points spatial distribution with the standard criterion for rejection of the key points. Practical Relevance. The results obtained make it possible to substantiate the need for development of a plot-oriented approach to terrain image processing by the key point search methods. The reason is that in order to perform and refine the spatial image linking, it is required to ensure the location uniformity of the key points used as control or joining points. It is revealed that the location density violation of the key points can be determined by the uneven image quality over the frame field. This phenomenon is associated, in particular, with different image sharpness in the central and peripheral zones.
Keywords: key points, optoelectronic photography, plot-oriented approach, image, rejection criterion, statistical and spatial distribution
References
References
1. Lobanova A., Ryzhova V., Korotaev V., Drozdova D. Solid-state optical radiation matrix receivers in robots’ vision systems. Studies in Systems, Decision and Control, 2020, vol. 261, pp. 171–188. doi: 10.1007/978-3-030-32710-1_13
2. Grigoriev A.N., Dmitrikov G.G., Popovich T.V., Pyatitskiy A.A., Smirnova O.V. Principles and examples of the remote sensing technology use for information support of spatial data infrastructures. Proceedings of the Mozhaisky Military Space Academy, 2018, no. 664, pp. 51–59. (in Russian)
3. Lazarenko V.P., Dzhamiykov T.S., Korotaev V.V., Yarishev S.N. Method for creation of spherical panoramas from images obtained by omnidirectional optoelectronic systems. Scientific and Technical Journal of Information Technologies, Mechanics and Optics, 2016, vol. 16, no. 1, pp. 46–53. (in Russian). doi: 10.17586/2226-1494-2016-16-1-46-53
4. Lowe D. Object recognition from local scale-invariant features. Proc. 7th IEEE International Conference on Computer Vision (ICCV'99), Kerkyra, Greece, 1999, vol. 2, pp. 1150–1157. doi: 10.1109/ICCV.1999.790410
5. Bay H., Tuytelaars T., Van Gool L. SURF: speeded up robust features. Lecture Notes in Computer Science (including subseries Lecture Notes in Artificial Intelligence and Lecture Notes in Bioinformatics), 2006, vol. 3951, pp. 404–417. doi: 10.1007/11744023_32
6. Rublee E., Rabaud V., Konolige K., Bradski G. ORB: an efficient alternative to SIFT or SURF. Proc. of the IEEE International Conference on Computer Vision (ICCV 2011), 2011, pp. 2564–2571. doi: 10.1109/ICCV.2011.6126544
7. Alcantarilla P.F., Bartoli A., Davison A.J. KAZE features. Lecture Notes in Computer Science (including subseries Lecture Notes in Artificial Intelligence and Lecture Notes in Bioinformatics), 2012, vol. 7577, pp. 214–227. doi: 10.1007/978-3-642-33783-3_16
8. Markushin G.N., Korotaev V.V., Koshelev A.V., Samokhina I.A., Vasil’ev A.S., Vasil’eva A.V., Yaryshev S.N. Image fusion in a dual-band scanning optoelectronic system for the search and detection of poaching activity. Journal of Optical Technology, 2020, vol. 87, no. 6, pp. 365–370. doi: 10.1364/JOT.87.000365
9. Rey-Otero I., Delbracio M. Anatomy of the SIFT method. Image Processing On Line, 2014, pp. 370–396. doi: 10.5201/ipol.2014.82
10. Ai M., Hu Q., Li J., Wang M., Yuan H., Wang S. A robust photogrammetric processing method of low-altitude UAV images. Remote Sensing, 2015, vol. 7, no. 3, pp. 2302–2333. doi: 10.3390/rs70302302
11. Fan B., Huo C., Pan C., Kong Q. Registration of optical and SAR satellite images by exploring the spatial relationship of the improved SIFT. IEEE Geoscience and Remote Sensing Letters, 2013, vol. 10, no. 4, pp. 657–661. doi: 10.1109/LGRS.2012.2216500
12. Berveglieri A., Tommaselli A. Multi-scale matching for the automatic location of control points in large scale aerial images using terrestrial scenes. ISPRS - International Archives of the Photogrammetry, Remote Sensing and Spatial Information Sciences, 2014, vol. 40, no. 3W1, pp. 27–31. doi: 10.5194/isprsarchives-XL-3-W1-27-2014
13. Wu C., Zhang L., Zhang L. A scene change detection framework for multi-temporal very high resolution remote sensing images. Signal Processing, 2016, vol. 124, pp. 184–197. doi: 10.1016/j.sigpro.2015.09.020
14. Liu F., Bi F., Chen L., Shi H., Liu W. Feature-area optimization: A novel sar image registration method. IEEE Geoscience and Remote Sensing Letters, 2016, vol. 13, no. 2, pp. 242–246. doi: 10.1109/LGRS.2015.2507982
15. Lingua A., Marenchino D., Nex F. Performance analysis of the SIFT operator for automatic feature extraction and matching in photogrammetric applications. Sensors, 2009, vol. 9, no. 5, pp. 3745–3766. doi: 10.3390/s90503745
16. Long T., Jiao W., He G., Zhang Z. A Fast and reliable matching method for automated georeferencing of remotely-sensed imagery. Remote Sensing, 2016, vol. 8, no. 1, pp. 56. doi: 10.3390/rs8010056
17. Ma Y., Chen F., Liu J., He Y., Duan J., Li X. An automatic procedure for early disaster change mapping based on optical remote sensing. Remote Sensing, 2016, vol. 8, no. 4, pp. 272. doi: 10.3390/rs8040272
18. Sima A.A., Buckley S.J. Optimizing SIFT for matching of short wave infrared and visible wavelength images. Remote Sensing, 2013, vol. 5, no. 5, pp. 2037–2056. doi: 10.3390/rs5052037
19. Sirmacek B., Unsalan C. Urban-area and building detection using SIFT keypoints and graph theory. IEEE Transactions on Geoscience and Remote Sensing, 2009, vol. 47, no. 4, pp. 1156–1167. doi: 10.1109/TGRS.2008.2008440
20. Sun Y., Zhao L., Huang S., Yan L., Dissanayake G. L2-SIFT: SIFT feature extraction and matching for large images in large-scale aerial photogrammetry. ISPRS Journal of Photogrammetry and Remote Sensing, 2014, vol. 91, pp. 1–16. doi: 10.1016/j.isprsjprs.2014.02.001
21. Suri S., Schwind P., Uhl J., Reinartz P. Modifications in the SIFT operator for effective SAR image matching. International Journal of Image and Data Fusion, 2010, vol. 1, no. 3, pp. 243–256. doi: 10.1080/19479832.2010.495322
22. Tong X., Liu X., Chen P., Liu S., Luan K., Li L., Liu S., Liu X., Xie H., Jin Y., Hong Z. Integration of UAV-based photogrammetry and terrestrial laser scanning for the three-dimensional mapping and monitoring of open-pit mine areas. Remote Sensing, 2015, vol. 7, no. 6, pp. 6635–6662. doi: 10.3390/rs70606635
23. Yang K., Pan A., Yang Y., Zhang S., Ong S.H., Tang H. Remote sensing image registration using multiple image features. Remote Sensing, 2017, vol. 9, no. 6, pp. 581. doi: 10.3390/rs9060581
24. Song Z.-I., Li S., George T.F. Remote sensing image registration approach based on a retrofitted SIFT algorithm and Lissajous-curve trajectories.Optic s Express, 2010, vol. 18, no. 2, pp. 513–522. doi: 10.1364/OE.18.000513
25. Hintze J.L., Nelson R.D. Violin plots: A box plot-density trace synergism. American Statistician, 1998, vol. 52, no. 2, pp. 181–184. doi: 10.1080/00031305.1998.10480559
26. Morris S., Tuttle J., Essic J. A partnership framework for geospatial data preservation in North Carolina. Library Trends, 2009, vol. 57, no. 3, pp. 516–540. doi: 10.1353/lib.0.0050