Menu
Publications
2024
2023
2022
2021
2020
2019
2018
2017
2016
2015
2014
2013
2012
2011
2010
2009
2008
2007
2006
2005
2004
2003
2002
2001
Editor-in-Chief
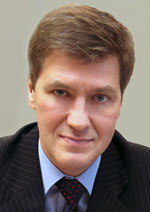
Nikiforov
Vladimir O.
D.Sc., Prof.
Partners
doi: 10.17586/2226-1494-2020-20-5-683-691
COMPARATIVE ANALYSIS OF METHODS FOR IMBALANCE ELIMINATION OF EMOTION CLASSES IN VIDEO DATA OF FACIAL EXPRESSIONS
Read the full article

Article in Russian
For citation:
Abstract
For citation:
Ryumina E.V., Karpov A.A. Comparative analysis of methods for imbalance elimination of emotion classes in video data of facial expressions. Scientific and Technical Journal of Information Technologies, Mechanics and Optics, 2020, vol. 20, no. 5, pp. 683–691 (in Russian). doi: 10.17586/2226-1494-2020-20-5-683-691
Abstract
Subject of Research. The imbalance of classes in datasets has a negative impact on machine classification systems used in applications of artificial intelligence, such as: medical diagnostics, fraud detection and risk management. This problem in facial expression datasets also degrades the performance of classification algorithms. Method. The paper discusses the main approaches for the class imbalance reduction: resampling methods and setting the weight of classes depending on the number of samples observed for an each class. A histogram of oriented gradients is used for the face area localization in the frame stream, then an active shape model is applied, which detects the coordinates of 68 key facial landmarks. Using the coordinates of key landmarks, informative features are extracted that characterize the dynamics of facial expressions. Main Results. The results of the study have shown that the proposed approach to the extraction of visual features exceeds the accuracy of human emotion recognition by facial expressions. The considered methods of the class imbalance reduction in the set of facial expressions have provided the improvement of machine classifier performance and showed that the existing class imbalance in a training set has a significant effect on the accuracy. Practical Relevance. The proposed approach to the extraction of visual features can be used in automatic systems for human emotion recognition by facial expressions, and result analysis of applying methods that reduce class imbalance can be useful for researchers in the field of machine learning.
Keywords: data class imbalance, under-sampling, over-sampling, classification, facial expression recognition, visual feature extraction, active shape model
Acknowledgements. This research was supported by the Russian Science Foundation
References
Acknowledgements. This research was supported by the Russian Science Foundation
References
1. Pandey S.K., Janghel R.R. Automatic detection of arrhythmia from imbalanced ECG database using CNN model with SMOTE. Australasian Physical & Engineering Sciences in Medicine, 2019, vol. 42, no. 4, pp. 1129–1139. doi: 10.1007/s13246-019-00815-9
2. Han W., Huang Z., Li S., Jia Y. Distribution-sensitive unbalanced data oversampling method for medical diagnosis. Journal of Medical Systems, 2019, vol. 43, no. 2, pp. 39. doi: 10.1007/s10916-018-1154-8
3. Ahammad J., Hossain N., Alam M.S. Credit card fraud detection using data pre-processing on imbalanced data - Both oversampling and undersampling. Proc. of the International Conference on Computing Advancements, 2020. doi: 10.1145/3377049.3377113
4. Velichko A., Karpov A. A study of data scarcity problem for automatic detection of deceptive speech utterances. CEUR Workshop Proceedings, 2020, vol. 2552, pp. 38–46.
5. Sun J., Lang J., Fujita H., Li H. Imbalanced enterprise credit evaluation with DTE-SBD: Decision tree ensemble based on SMOTE and bagging with differentiated sampling rates. Information Sciences, 2018, vol. 425, pp. 76–91. doi: 10.1016/j.ins.2017.10.017
6. Leong C.K. Credit risk scoring with bayesian network models. Computational Economics, 2016, vol. 47, no. 3, pp. 423–446. doi: 10.1007/s10614-015-9505-8
7. Li S., Deng W. Real world expression recognition: A highly imbalanced detection problem. Proc. 9th International Conference on Biometrics (ICB 2016), 2016, pp. 7550074. doi: 10.1109/ICB.2016.7550074
8. Kaya H., Karpov A.A. Introducing weighted kernel classifiers for handling imbalanced paralinguistic corpora: Snoring, addressee and cold. Proc. 18th Annual Conference of the International Speech Communication Association (INTERSPEECH 2017), 2017, pp. 3527–3531. doi: 10.21437/Interspeech.2017-653
9. Johnson J.M., Khoshgoftaar T.M. Survey on deep learning with class imbalance. Journal of Big Data, 2019, vol. 6, no. 1, pp. 27. doi: 10.1186/s40537-019-0192-5
10. Chawla N.V., Bowyer K.W., Hall L.O., Kegelmeyer W.P. SMOTE: synthetic minority over-sampling technique. Journal of Artificial Intelligence Research, 2002, vol. 16, pp. 321–357. doi: 10.1613/jair.953
11. He H., Bay Y., Garcia E.A., Li S. ADASYN: Adaptive Synthetic Sampling Approach for Imbalanced Learning. Proc. of the IEEE International Joint Conference on Neural Networks (IJCNN 2008), 2008, pp. 1322–1328. doi: 10.1109/IJCNN.2008.4633969
12. Liu Z.-T., Wu B.-H., Li D.-Y., Xiao P., Mao J.-W. Speech emotion recognition based on selective interpolation synthetic minority over-sampling technique in small sample environment. Sensors, 2020, vol. 20, no. 8, pp. 2297. doi: 10.3390/s20082297
13. Li S., Deng W. Deep emotion transfer network for cross-database facial expression recognition. Proc. 24th International Conference on Pattern Recognition (ICPR 2018), 2018, pp. 3092–3099. doi: 10.1109/ICPR.2018.8545284
14. Rashid T.A. Convolutional neural networks based method for improving facial expression recognition. Advances in Intelligent Systems and Computing, 2016, vol. 530, pp. 73–84. doi: 10.1007/978-3-319-47952-1_6
15. Yi W., Sun Y., He S. Data augmentation using conditional GANs for facial emotion recognition. Proc. Progress in Electromagnetics Research Symposium (PIERS-Toyama 2018), 2018, pp. 710–714. doi: 10.23919/PIERS.2018.8598226
16. Cao H., Cooper D.G., Keutmann M.K., Gur R.C., Nenkova A., Verma R. CREMA-D: Crowd-sourced emotional multimodal actors dataset. IEEE Transactions on Affective Computing, 2014, vol. 5, no. 4, pp. 377–390. doi: 10.1109/TAFFC.2014.2336244
17. Wilson D.L. Asymptotic properties of nearest neighbor rules using edited data. IEEE Transactions on Systems, Man and Cybernetics, 1972, vol. 2, no. 3, pp. 408–421. doi: 10.1109/TSMC.1972.4309137
18. Tomek I. Two modifications of CNN. IEEE Transactions on Systems, Man and Cybernetics, 1976, vol. 6, no. 11, pp. 769–772. doi: 10.1109/TSMC.1976.4309452
19. Kubat M., Matwin S. Addressing the curse of imbalanced training sets: one-sided selection. Proc. 14th International Conference on Machine Learning, 1997, pp. 179–186.
20. Zhang I., Mani I. kNN approach to unbalanced data distributions: a case study involving information extraction. Proc. of Workshop on Learning from Imbalanced Datasets, 2003, pp. 42–48.
21. Lemaître G., Nogueira F., Aridas C.K. Imbalanced-learn: A python toolbox to tackle the curse of imbalanced datasets in machine learning. Journal of Machine Learning Research, 2017, vol. 18, pp. 559–563.
22. Han H., Wang W.-Y., Mao B.-H. Borderline-SMOTE: a new over-sampling method in imbalanced data sets learning. Lecture Notes in Computer Science, 2005, vol. 3644, pp. 878–887. doi: 10.1007/11538059_91
23. Nguyen H.M., Cooper E.W., Kamei K. Borderline over-sampling for imbalanced data classification. International Journal of Knowledge Engineering and Soft Data Paradigms (IJKESDP), 2011, vol. 3, no. 1, pp. 4–21. doi: 10.1504/IJKESDP.2011.039875
24. Déniz O., Bueno G., Salido J., De La Torre F. Face recognition using histograms of oriented gradients. Pattern Recognition Letters, 2011, vol. 32, no. 12, pp. 1598–1603. doi: 10.1016/j.patrec.2011.01.004
25. Cootes T.F., Taylor C.J., Cooper D.H., Graham J. Active shape models-their training and application. Computer Vision and Image Understanding, 1995, vol. 61, no. 1, pp. 38–59. doi: 10.1006/cviu.1995.1004
26. King D.E. Dlib-ml: A machine learning toolkit. Journal of Machine Learning Research, 2009, vol. 10, pp. 1755–1758.
27. Van Gent P. Emotion Recognition Using Facial Landmarks Python DLib and OpenCV. A tech blog about fun things with Python Embed. Electron, 2016. Available at: http://www.paulvangent.com/2016/08/05/emotion-recognition-using-facial-landmarks/ (accessed: 19.07.2020).
28. Mollahosseini A., Hasani B., Mahoor M.H. AffectNet: A database for facial expression, valence, and arousal computing in the wild. IEEE Transactions on Affective Computing, 2019, vol. 10, no. 1, pp. 18–31. doi: 10.1109/TAFFC.2017.2740923
29. Carrier P. L., Courville A., Goodfellow I. J., Mirza M., Bengio Y. FER-2013 face database. Technical report 1365. Universit de Montral, 2013.
30. Li S., Deng W., Du J. Reliable crowdsourcing and deep locality-preserving learning for expression recognition in the wild. Proc. 30th IEEE Conference on Computer Vision and Pattern Recognition (CVPR 2017), 2017, pp. 2584–2393. doi: 10.1109/CVPR.2017.277
31. Ghaleb E., Popa M., Asteriadis S. Metric Learning-Based Multimodal Audio-Visual Emotion Recognition. IEEE Multimedia, 2020, vol. 27, no. 1, pp. 37–48. doi: 10.1109/MMUL.2019.2960219