Menu
Publications
2025
2024
2023
2022
2021
2020
2019
2018
2017
2016
2015
2014
2013
2012
2011
2010
2009
2008
2007
2006
2005
2004
2003
2002
2001
Editor-in-Chief
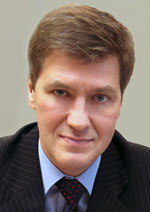
Nikiforov
Vladimir O.
D.Sc., Prof.
Partners
doi: 10.17586/2226-1494-2020-20-6-807-814
METHOD OF JOINT CLUSTERING IN NETWORK AND CORRELATION SPACES
Read the full article

Article in Russian
For citation:
Abstract
For citation:
Gainullina A.N., Artyomov M., Sergushichev A.A. Method of joint clustering in network and correlation spaces. Scientific and Technical Journal of Information Technologies, Mechanics and Optics, 2020, vol. 20, no. 6, pp. 807-814 (in Russian). doi: 10.17586/2226-1494-2020-20-6-807-814
Abstract
Subject of Research. The joint clustering method in network and correlation context is designed to identify active modules in metabolic graphs based on transcriptomic data represented by a large number of samples. The active modules obtained by this method describe the dynamic metabolic regulation in all samples of the analyzed dataset. The paper presents modifications of the proposed method for application on real data. Method. For results stability study the modified method was repeatedly run on real data with small variations of the initial parameters. For result analysis, several metrics were formulated that display modules similarity and representation under various start-up conditions. Main Results. The analysis results are sufficiently robust. For the most modules, their profiles are detected well in the noisy data, and the most genes are also preserved. Practical Relevance. The results of the presented study have shown that the modified method analyzes successfully real data by producing active modules that are stable and easy in interpretation.
Keywords: clustering, correlation, graphs, metabolic networks, gene expression, transcriptomic data
Acknowledgements. This work was supported by the Government of the Russian Federation, Investigation Research Grant 08-08.
References
Acknowledgements. This work was supported by the Government of the Russian Federation, Investigation Research Grant 08-08.
References
1. Van den Bossche J., O’Neill L.A., Menon D. Macrophage immunometabolism: where are we (going)? Trends in Immunology, 2017, vol. 38, no. 6, pp. 395–406. doi: 10.1016/j.it.2017.03.001
2. Al-Khami A.A., Rodriguez P.C., Ochoa A.C. Energy metabolic pathways control the fate and function of myeloid immune cells. Journal of Leukocyte Biology, 2017, vol. 102, no. 2, pp. 369–380. doi: 10.1189/jlb.1VMR1216-535R
3. Wculek S.K., Khouili S.C., Priego E., Heras-Murillo I., Sancho D. Metabolic control of dendritic cell functions: digesting information. Frontiers in immunology, 2019, vol. 10, pp. 775. doi: 10.3389/fimmu.2019.00775
4. Wang Z., Gerstein M., Snyder M. RNA-Seq: a revolutionary tool for transcriptomics. Nature Reviews Genetics, 2009, vol. 10, no. 1, pp. 57–63. doi: 10.1038/nrg2484
5. Chen G., Ning B., Shi T. Single-cell RNA-Seq technologies and related computational data analysis. Frontiers in Genetics, 2019, vol. 10, pp. 317. doi: 10.3389/fgene.2019.00317
6. Beisser D., Grohme M.A., Kopka J., Frohme M., Schill R.O., Hengherr S., Dandekar T., Klau G.W., Dittrich M., Müller T. Integrated pathway modules using time-course metabolic profiles and EST data from Milnesium tardigradum. BMC Systems Biology, 2012, vol. 6, pp. 72. doi: 10.1186/1752-0509-6-72
7. Jha A.K., Huang S.C., Sergushichev A., Lampropoulou V., Ivanova Y., Loginicheva E., Chmielewski K., Stewart K., Ashall J., Everts B., Pearce E., Driggers E.M., Artyomov M.N. Network integration of parallel metabolic and transcriptional data reveals metabolic modules that regulate macrophage polarization. Immunity, 2015, vol. 42, no. 3, pp. 419–430. doi: 10.1016/j.immuni.2015.02.005