Menu
Publications
2024
2023
2022
2021
2020
2019
2018
2017
2016
2015
2014
2013
2012
2011
2010
2009
2008
2007
2006
2005
2004
2003
2002
2001
Editor-in-Chief
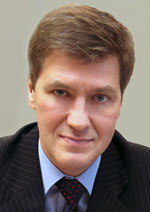
Nikiforov
Vladimir O.
D.Sc., Prof.
Partners
doi: 10.17586/2226-1494-2020-20-6-815-827
CONCEPT OF DIGITAL TWINS AT LIFE CYCLE STAGES OF PRODUCTION SYSTEMS
Read the full article

Article in Russian
For citation:
Abstract
For citation:
Shvedenko V.N., Mozokhin A.E. Concept of digital twins at life cycle stages of production systems. Scientific and Technical Journal of Information Technologies, Mechanics and Optics, 2020, vol. 20, no. 6, pp. 815-827 (in Russian). doi: 10.17586/2226- 1494-2020-20-6-815-827
Abstract
Subject of Research. The paper analyzes the current concept of digital twins in relation to production systems. The review is given for characteristics and parameters of the digital twin that create advantages of using this concept for production systems at their life cycle stages. Technical implementation variants for the concept of digital twins are proposed aimed at improvement of the technological processes of production systems at the following stages of the life cycle: an idea, project, realization, operation, and disposal. Method. We performed a retrospective analysis of scientific papers on the methodology and practical application of software-based approaches to the design, implementation and operation of industrial systems in Industry 4.0. An expert assessment and applicability analysis of the digital twins at the stages of the life cycle of production systems are given. Main Results. The main characteristics of the digital twin affecting the effectiveness of the concept application in production systems are determined and arranged into groups according to their importance based on the analysis of the foreign studies over the past 10 years. The advantages and disadvantages of a software-oriented approach application to the design, implementation and operation of production systems are formulated. Practical Relevance. The digital twin characteristics are determined that affect the efficiency of its usage at the life cycle stages of production systems. Variants of the modern concept technical implementation of digital twins for production systems are proposed by applying of advanced digital technologies and intelligent electronic devices. Requirements are systematized concerning functionality, performance, correspondence accuracy of the virtual and physical environment state, as well as the qualitative characteristics of the digital twin. They provide for the assessment about the applicability of digital twins for solving existing problems of control and management in production systems.
Keywords: digital twin, digital environment design, digital twin application, product life cycle, cyber-physical systems
References
References
1. Radhakisan B., Gill H. Cyber-physical systems. The Impact of Control Technology, 2011, pp. 161–166.
2. Rosen R., Wichert G., Lo G., Bettenhausen K.D. About the importance of autonomy and digital twins for the future of manufacturing. IFAC-PapersOnLine, 2015, vol. 48, no. 3, pp. 567–572. doi: 10.1016/j.ifacol.2015.06.141
3. Laengle S., Modak N.M., Merigó J.M., De La Sotta C. Thirty years of the international journal of computer integrated manufacturing: a bibliometric analysis. International Journal of Computer Integrated Manufacturing, 2018, vol. 31, no. 12, pp. 1247–1268. doi: 10.1080/0951192X.2018.1529434
4. Abramovici M., Göbel J.C., Savarino P. Reconfiguration of smart products during their use phase based on virtual product twins. CIRP Annals - Manufacturing Technology, 2017, vol. 66, no. 1, pp. 165–168. doi: 10.1016/j.cirp.2017.04.042
5. Ayani M., Ganebäck M., Ng A.H.C. Digital Twin: applying emulation for machine reconditioning. Procedia CIRP, 2018, vol. 82, pp. 243–248. doi: 10.1016/j.procir.2018.03.139
6. Baruffaldi G., Accorsi R., Manzini R. Warehouse management system customization and information availability in 3pl companies: a decision-support tool. Industrial Management & Data Systems, 2019, vol. 119, no. 2, pp. 251–273. doi: 10.1108/IMDS-01-2018-0033
7. Stark R., Kind S., Neumeyer S. Innovations in digital modelling for next generation manufacturing system design. CIRP Annals - Manufacturing Technology, 2017, vol. 66, no. 1, pp. 169–172. doi: 10.1016/j.cirp.2017.04.045
8. Guerineau B., Bricogne M., Durupt A., Rivest L. Mechatronics vs. cyber physical systems: Towards a conceptual framework for a suitable design methodology. Proc. 11th France–Japan and 9th Europe-Asia Congress on Mechatronics (MECATRONICS) / 17th International Conference on Research and Education in Mechatronics (REM), Compiègne, France, 2016, pp. 314–320. doi: 10.1109/MECATRONICS.2016.7547161
9. Lee E.A. Cyber physical systems: design challenges. Proc. 11th IEEE International Symposium on Object/Component/Oriented Real-Time Distributed Computing (ISORC 2008), Orlando, FL, USA, 2008, pp. 363–369. doi: 10.1109/ISORC.2008.25
10. Lee J., Bagheri B., Kao H.-A. A Cyber-Physical Systems architecture for Industry 4.0-based manufacturing systems. Manufacturing Letters, 2015, vol. 3, pp. 18–23. doi: 10.1016/j.mfglet.2014.12.001
11. Grieves M. Digital twin: manufacturing excellence through virtual factory replication. White paper, 2014, vol. 1, pp. 1–7.
12. Grieves M., Vickers J. Digital Twin: Mitigating unpredictable, undesirable emergent behavior in complex systems. Transdisciplinary Perspectives on Complex Systems: New Findings and Approaches. Springer, 2017, pp. 85–113. doi: 10.1007/978-3-319-38756-7_4
13. Xiang F., Zhi Z. Jiang G. Digital twins technology and its data fusion in iron and steel product life cycle. Proc. 15th IEEE International Conference on Networking, Sensing and Control (ICNSC), 2018, pp. 1–5. doi: 10.1109/ICNSC.2018.8361293
14. Söderberg R., Wärmefjord K., Carlson J.S., Lindkvist L. Toward a Digital Twin for real-time geometry assurance in individualized production. CIRP Annals - Manufacturing Technology, 2017, vol. 66, pp. 137–140. doi: 10.1016/j.cirp.2017.04.038
15. Guo F., Zou F., Liu J., Wang Z. Working mode in aircraft manufacturing based on digital coordination model. International Journal of Advanced Manufacturing Technology, 2018, vol. 98, no. 5-8, pp. 1547–1571. doi: 10.1007/s00170-018-2048-0
16. Zhang M., Zuo Y., Tao F. Equipment energy consumption management in digital twin shop-floor: A framework and potential applications. Proc. 15th IEEE International Conference on Networking, Sensing and Control (ICNSC), 2018, pp. 1–5. doi: 10.1109/ICNSC.2018.8361272
17. Miled Z.B., French M.O. Towards a reasoning framework for digital clones using the digital thread. Proc. 55th AIAA Aerospace Sciences Meeting, 2017, pp. 0873. doi: 10.2514/6.2017-0873
18. Schroeder G.N., Steinmetz C., Pereira C.E., Espindola D.B. Digital twin data modeling with automationML and a communication methodology for data exchange. IFAC-PapersOnLine, 2016, vol. 49, no. 30, pp. 12–17. doi: 10.1016/j.ifacol.2016.11.115
19. Talkhestani B.A., Jazdi N., Schloegl W., Weyrich M. Consistency check to synchronize the Digital Twin of manufacturing automation based on anchor points. Procedia CIRP, 2018, vol. 72, pp. 159–164. doi: 10.1016/j.procir.2018.03.166
20. Torkamani A., Andersen K.G., Steinhubl S.R., Topol E.J. High-definition medicine. Cell, 2017, vol. 170, no. 5, pp. 828–843. doi: 10.1016/j.cell.2017.08.007
21. Glaessgen E., Stargel D. The digital twin paradigm for future NASA and U.S. Air Force vehicles. Proc. 53rd AIAA/ASME/ASCE/AHS/ASC Structures, Structural Dynamics and Materials Conference 20th AIAA/ASME/AHS Adaptive Structures Conference 14th AIAA, 2012, pp. 1818. doi: 10.2514/6.2012-1818
22. Cai Y., Starly B., Cohen P., Lee Y.-S. Sensor data and information fusion to construct digital-twins virtual machine tools for cyber-physical manufacturing. Procedia Manufacturing, 2017, vol. 10, pp. 1031–1042. doi: 10.1016/j.promfg.2017.07.094
23. Zhang H., Liu Q., Chen X., Zhang D., Leng J. A digital twin-based approach for designing and multi-objective optimization of hollow glass production line. IEEE Access, 2017, vol. 5, pp. 26901–26911. doi: 10.1109/ACCESS.2017.2766453
24. Weyer S., Meyer T., Ohmer M., Gorecky D., Zühlke D. Future modeling and simulation of cps-based factories: an example from the automotive industry. IFAC-PapersOnLine, 2016, vol. 49, no. 31, pp. 97–102. doi: 10.1016/j.ifacol.2016.12.168
25. Guo J., Zhao N., Sun L., Zhang S. Modular based flexible digital twin for factory design. Journal of Ambient Intelligence and Humanized Computing, 2019, vol. 10, no. 3, pp. 1189–1200. doi: 10.1007/s12652-018-0953-6
26. Uhlemann T.H.J., Schock C., Lehmann C., Freiberger S., Steinhilper R. The digital twin: demonstrating the potential of real time data acquisition in production systems. Procedia Manufacturing, 2017, vol. 9, pp. 113–120. doi: 10.1016/j.promfg.2017.04.043
27. Kraft E.M. Developing a digital thread / digital twin aerodynamic performance authoritative truth source. Proc. 2018 Aviation Technology, Integration, and Operations Conference, 2018, pp. 4003. doi: 10.2514/6.2018-4003
28. Hu L., Nguyen N.T., Tao W., Leu M.C., Liu X.F., Shahriar M.R., Al Sunn S.M.N. Modeling of cloud-based digital twins for smart manufacturing with MT connect. Procedia Manufacturing, 2018, vol. 26, pp. 1193–1203. doi: 10.1016/j.promfg.2018.07.155
29. Cheng J., Chen W., Tao F., Lin C.-L. Industrial IoT in 5G environment towards smart manufacturing. Journal of Industrial Information Integration, 2018, vol. 10, pp. 10–19. doi: 10.1016/j.jii.2018.04.001
30. Qi Q., Tao F. Digital twin and big data towards smart manufacturing and industry 4.0: 360 degree comparison. IEEE Access, 2018, vol. 6, pp. 3585–3593. doi: 10.1109/ACCESS.2018.2793265
31. Schleich B., Anwer N., Mathieu L., Wartzack S. Shaping the digital twin for design and production engineering. CIRP Annals - Manufacturing Technology, 2017, vol. 66, no. 1, pp. 141–144. doi: 10.1016/j.cirp.2017.04.040
32. Lorenz M., Rüßmann M., Gerbert P., Waldner M., Justus J., Engel P., Harnisch M. Industry 4.0: the future of productivity and growth in manufacturing industries. Boston Consulting Group, 2015, 20 p. Available at: https://image-src.bcg.com/Images/Industry_40_Future_of_Productivity_April_2015_tcm9-61694.pdf (accessed: 04.09.2020).
33. Kuts V., Modoni G.E., Terkaj W., Tähemaa T., Sacco M., Otto T. Exploiting factory telemetry to support virtual reality simulation in robotics cell. Lecture Notes in Computer Science (including subseries Lecture Notes in Artificial Intelligence and Lecture Notes in Bioinformatics), 2017, vol. 10324, pp. 212–221. doi: 10.1007/978-3-319-60922-5_16
34. Zhang H., Zhang G., Yan Q. Dynamic resource allocation optimization for digital twin-driven smart shopfloor. Proc. 15th IEEE International Conference on Networking, Sensing and Control (ICNSC), 2018, pp. 1–5. doi: 10.1109/ICNSC.2018.8361283
35. Leng J., Zhang H., Yan D., Liu Q., Chen X., Zhang D. Digital twin-driven manufacturing cyber-physical system for parallel controlling of smart workshop. Journal of Ambient Intelligence and Humanized Computing, 2019, vol. 10, no. 3, pp. 1155–1166. doi: 10.1007/s12652-018-0881-5
36. Cheng Y., Zhang Y., Ji P., Xu W., Zhou Z., Tao F. Cyber-physical integration for moving digital factories forward towards smart manufacturing: a survey. International Journal of Advanced Manufacturing Technology, 2018, vol. 97, no. 1-4, pp. 1209–1221. doi: 10.1007/s00170-018-2001-2
37. Zheng Y., Yang S., Cheng H. An application framework of digital twin and its case study. Journal of Ambient Intelligence and Humanized Computing, 2019, vol. 10, no. 3, pp. 1141–1153. doi: 10.1007/s12652-018-0911-3
38. Botkina D., Hedlind M., Olsson B., Henser J., Lundholm T. Digital twin of a cutting tool. Procedia CIRP, 2018, vol. 72, pp. 215–218. doi: 10.1016/j.procir.2018.03.178
39. Kritzinger W., Karner M., Traar G., Henjes J., Sihn W. Digital twin in manufacturing: a categorical literature review and classification. IFAC-PapersOnLine, 2018, vol. 51, no. 11, pp. 1016–1022. doi: 10.1016/j.ifacol.2018.08.474
40. Lohtander M., Ahonen N., Lanz M., Ratava J., Kaakkunen J. Micro manufacturing unit and the corresponding 3D-model for the digital twin. Procedia Manufacturing, 2018, vol. 25, pp. 55–61. doi: 10.1016/j.promfg.2018.06.057
41. Zhuang C., Liu J., Xiong H. Digital twin-based smart production management and control framework for the complex product assembly shop-floor. International Journal of Advanced Manufacturing Technology, 2018, vol. 96, no. 1-4, pp. 1149–1163. doi: 10.1007/s00170-018-1617-6
42. Clement S.J., McKee D.W., Romano R., Xu J., Lopez J.M., Battersby D. The Internet of Simulation: enabling agile model based systems engineering for cyber-physical systems. Proc. 12th System of Systems Engineering Conference (SoSE), 2017, pp. 7994948. doi: 10.1109/SYSOSE.2017.7994948
43. Negri E., Fumagalli L., Macchi M. A review of the roles of digital twin in CPS-based production systems. Procedia Manufacturing, 2017, vol. 11, pp. 939–948. doi: 10.1016/j.promfg.2017.07.198
44. Stark J. Product lifecycle management. Product Lifecycle Management (V. 1). 21st Century Paradigm for Product Realisation. Springer, 2015, pp. 1–29. doi: 10.1007/978-3-319-17440-2_1
45. Damjanovic-Behrendt V. A digital twin-based privacy enhancement mechanism for the automotive industry. Proc. 9th International Conference on Intelligent Systems (IS), 2018, pp. 272–279. doi: 10.1109/IS.2018.8710526
46. Beregi R., Szaller Á., Kádár B. Synergy of multi-modelling for process control. IFAC-PapersOnLine, 2018, vol. 51, no. 11, pp. 1023–1028. doi: 10.1016/j.ifacol.2018.08.473
47. Zheng P., Lin T.J., Chen C.H., Xu X. A systematic design approach for service innovation of smart product-service systems. Journal of Cleaner Production, 2018, vol. 201, pp. 657–667. doi: 10.1016/j.jclepro.2018.08.101
48. Luo W., Hu T., Zhang C., Wei Y. Digital twin for CNC machine tool: modeling and using strategy. Journal of Ambient Intelligence and Humanized Computing, 2019, vol. 10, no. 3, pp. 1129–1140. doi: 10.1007/s12652-018-0946-5
49. Schroeder G., Steinmetz C., Pereira C.E., Muller I., Garcia N., Espindola D., Rodrigues R. Visualising the digital twin using web services and augmented reality. Proc. 14th IEEE International Conference on Industrial Informatics (INDIN), 2016, pp. 522–527. doi: 10.1109/INDIN.2016.7819217
50. El Saddik A. Digital twins: the convergence of multimedia technologies. IEEE MultiMedia, 2018, vol. 25, no. 2, pp. 87–92. doi: 10.1109/MMUL.2018.023121167
51. Qi Q., Tao F., Zuo Y., Zhao D. Digital twin service towards smart manufacturing. Procedia CIRP, 2018, vol. 72, pp. 237–242. doi: 10.1016/j.procir.2018.03.103
52. Macchi M., Roda I., Negri E., Fumagalli L. Exploring the role of digital twin for asset lifecycle management. IFAC-PapersOnLine, 2018, vol. 51, no. 11, pp. 790–795. doi: 10.1016/j.ifacol.2018.08.415
53. Landolfi G., Barni A., Menato S., Cavadini F.A., Rovere D., Dal Maso G. Design of a multi-sided platform supporting CPS deployment in the automation market. Proc. 1st IEEE International Conference on Industrial Cyber-Physical Systems (ICPS), 2018, pp. 684–689. doi: 10.1109/ICPHYS.2018.8390790
54. Bitton R., Gluck T., Stan O., Inokuchi M., Ohta Y., Yamada Y., Yagyu T., Elovici Y., Shabtai A. Deriving a cost-effective digital twin of an ICS to facilitate security evaluation. Lecture Notes in Computer Science (including subseries Lecture Notes in Artificial Intelligence and Lecture Notes in Bioinformatics), 2018, vol. 11098, pp. 533–554. doi: 10.1007/978-3-319-99073-6_26