Menu
Publications
2025
2024
2023
2022
2021
2020
2019
2018
2017
2016
2015
2014
2013
2012
2011
2010
2009
2008
2007
2006
2005
2004
2003
2002
2001
Editor-in-Chief
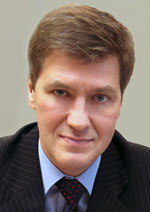
Nikiforov
Vladimir O.
D.Sc., Prof.
Partners
doi: 10.17586/2226-1494-2020-20-6-893-897
CLINICAL DECISIONSUPPORT SYSTEM WITH PROCESSING OF MULTIMODAL MEDICAL DATA FOR RADIOLOGIST EFFICIENCY IMPROVEMENT PRACTICE
Read the full article

Article in Russian
For citation:
Abstract
For citation:
Lobantsev A.A.Clinical decision support system with processing of multimodal medical data for radiologist efficiency improvement practice. Scientific and Technical Journal of Information Technologies, Mechanics and Optics, 2020, vol. 20, no. 6, pp. 893-897 (in Russian). doi: 10.17586/2226-1494-2020-20-6-893-897
Abstract
Subject of Research.The paper proposes a clinical decision support system using multimodal data. Method. The system is based on neural network models. The author proposes the methods of transfer learning with domain adaptation techniques, training on multimodal data and taking into account the negative knowledge transfer for the quality improvement of preliminary analysis of medical data with application of neural network models. Algorithms of neural networks for preliminary analysis of the patient study provide the analysis of multimodal data with consideration of the combination peculiarities of modalities, training of neural networks with specific domain adaptation techniques, and taking into account the phenomenon of the knowledge negative transfer for the increase of the neural network robustness to the natural equipment noise. Main Results. The proposed system is implemented as a web-platform. The implemented system effectiveness was assessed by involving 30 experts: doctors, students, and patients. A comparative assessment of the problems solution in various scenarios was carried out with the proposed system and without it. Experiments have shown that the proposed system application reduces the execution time of the most critical stages of scenarios. Practical Relevance. The proposed system is located in the ITMO domain at the uniform resource locatoraddress: http://mcp.itmo.ru and is used in the practice of the Almazov National Medical Research Centre of the Ministry of Health of Russia, and the “City Children's Infectious Disease Clinical Hospital” Healthcare Institution in Minsk. The system is recommended for the application in clinical hospitals, individual practitioners and teaching radiologists.
Keywords: clinical decision support systems, neural networks, knowledge transfer, multimodal data processing, radiological study analysis
Acknowledgements. This work was financially supported by the Russian Science Foundation, Grant N19-19-00696.
References
Acknowledgements. This work was financially supported by the Russian Science Foundation, Grant N19-19-00696.
References
1. Rawson T., Moore L.S.P., Charani E., Castro-Sanchez E., Holmes A.H., Hernandez B., Herrero P., Georgiou P., Hayhoe B. A systematic review of clinical decision support systems for antimicrobial management: are we failing to investigate these interventions appropriately? Clinical Microbiology and Infection, 2017, vol. 23, no. 8, pp. 524–532. doi: 10.1016/j.cmi.2017.02.028
2. Reis W.C., Bonetti A.F., Bottacin W.E., Reis A.S.,Jr., Souza T.T., Pontarolo R., Correr C.J., Fernandez-Llimos F. Impact on process results of clinical decision support systems (CDSSs) applied to medication use: overview of systematic reviews. Pharmacy Practice, 2017, vol. 15, no. 4, pp. 1036. doi: 10.18549/PharmPract.2017.04.1036
3. Maninis K.-K., Caelles S., Pont-Tuset J., Van Gool L. Deep extreme cut: From extreme points to object segmentation. Proc. 31st Meeting of the IEEE/CVF Conference on Computer Vision and Pattern Recognition (CVPR 2018), 2018, pp. 616–625. doi: 10.1109/CVPR.2018.00071
4. Aresta G., Jacobs C., Araújo T., Cunha A., Ramos I., van Ginneken B., Campilho A. iW-Net: an automatic and minimalistic interactive lung nodule segmentation deep network. Scientific Reports, 2019, vol. 9, no. 1, pp. 11591. doi: 10.1038/s41598-019-48004-8
5. Zheng S., Guo J., Cui X., Veldhuis R.N.J., Oudkerk M., Van Ooijen P.M.A. Automatic pulmonary nodule detection in CT scans using convolutional neural networks based on maximum intensity projection. IEEE Transactions on Medical Imaging, 2020, vol. 39, no. 3, pp. 797–805. doi: 10.1109/TMI.2019.2935553
6. Orenstein B. Virtually indispensable. Radiology Today, 2018, vol. 19, no. 12, pp. 18.
7. Vatian A., Lobantsev A., Gorokhov N., Mirzayanov M., Korneev G., Gusarova N., Shalyto A. Data processing approaches for lung CT-image analysis under resource constraints. Multi Conference on Computer Science and Information Systems, MCCSIS 2019 – Proceedings of the 11th International Conference on e-Health 2019, 2019, pp. 19–26. doi: 10.33965/eh2019_201910l003
8. Vatian A., Gusarova N., Dobrenko N., Dudorov S., Nigmatullin N., Shalyto A., Lobantsev A. Impact of adversarial examples on the efficiency of interpretation and use of information from high-tech medical images. Proc. 24th Conference of Open Innovations Association (FRUCT), 2019, pp. 472–478. doi: 10.23919/FRUCT.2019.8711974
9. Lobantsev A.A., Gusarova N.F., Vatian A.S., Kapitonov A.A., Shalyto A.A. Comparative assessment of text-image fusion models for medical diagnostics. Informatsionno-Upravliaiushchie Sistemy, 2020, no. 5, pp. 70–79. doi: 10.31799/1684-8853-2020-5-70-79