Menu
Publications
2025
2024
2023
2022
2021
2020
2019
2018
2017
2016
2015
2014
2013
2012
2011
2010
2009
2008
2007
2006
2005
2004
2003
2002
2001
Editor-in-Chief
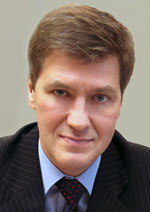
Nikiforov
Vladimir O.
D.Sc., Prof.
Partners
doi: 10.17586/2226-1494-2021-21-1-85-91
HUMAN PSYCHE CREATION BY APPLICATION OF NATURAL LANGUAGE PROCESSING TECHNOLOGIES
Read the full article

Article in русский
For citation:
Abstract
For citation:
Tatarnikova T.M., Bogdanov P.Yu. Human psyche creation by application of natural language processing technologies. Scientific and Technical Journal of Information Technologies, Mechanics and Optics, 2021, vol. 21, no. 1, pp. 85–91 (in Russian). doi: 10.17586/2226-1494-2021-21-1-85-91
Abstract
Subject of Research. The paper proposes a solution for the human psyche automatic creation based on his speech behavior analysis. It is shown that messages in social networks, instant messengers and chats can be used to form a training data set, both in the format of text messages and audio and video calls. The functions of the psychological type classifier constituents are revealed by the human speech behavior. A comparison is made between multiclass and binary classification based on the loss function minimization. Methods. The human psyche corresponded to the Myers-Briggs type indicator, which subsumes a person to one of 16 types. The technologies of Text Mining for natural language processing and a deep learning model for speech processing were used. The data set for training and testing was formed by recordings of people’s speech translated into text format. Class labels were formed by the content of a text parameters vector, which is a dictionary of frequently encountered words. A deep learning algorithm was used for the human psyche automatic creation and was based on recurrent neural networks of the Long Short-Term Memory type. The algorithm was tested both for multiclass and binary classification. The objectivity of the proposed approach to a human psyche creation was ensured by the variety of content created by a person at various time in accordance with life situations, profession, hobbies and other circumstances. Main Results. A new approach to the automatic human psyche creation is proposed, based on the binary classification and a deep learning model. The convergence of the binary classification results with the test set of the speech behavior of various people is demonstrated. The Long Short-Term Memory network application in binary classification makes it possible to achieve an accuracy equal to 83 % of the psychological type correct determination and reduce the losses to 25 %. Practical Relevance. Automatic human psyche creation based on his speech behavior enables various specialists (such as psychologists, sociologists, human resources staff members) to make decisions when working with a specific person. Analysis of a human personal qualities by his speech behavior is software-implemented.
Keywords: human psyche, speech behavior, natural language processing, classification by temperament type, Text Mining, machine learning, deep learning
References
References
1. Eisenstein J. Introduction to Natural Language Processing. Cambridge, MIT Press, 2019, 536 p.
2. Gary S.J., Alexander R.A., Friedt L. Item analysis of the Myers- Briggs Type Indicator. Educational and Psychological Measurement, 1985, vol. 45, no. 4, pp. 789–796. doi: 10.1177/0013164485454009
3. Thompson B., Borrello G. Construct Validity of the Myers-Briggs Type Indicator. Educational and Psychological Measurement, 1986, vol. 46, no. 3, pp. 745–752. doi: 10.1177/0013164486463032
4. Tatarnikova T.M. Data Analysis. St. Petersburg, SPbSEU Publ., 2018, 85 p. (in Russian)
5. Bogdanov D.S. Speech recognition systems: classification, methods, and algorithms for implementation. Alley of Sciences, 2018, vol. 7, no. 11(27), pp. 819–823. (in Russian)
6. Sovetov B.Ya., Tatarnikova T.M., Yashin A.I. Use of technology TextMining for identifying hidden threats in communications distributed by social networks. Izvestiya SPbGETU LETI, 2019, no. 4, pp. 26–32. (in Russian)
7. Aggarwal C.C., Zhai C. Mining Text Data. Springer, 2012, 527 p. doi: 10.1007/978-1-4614-3223-4
8. Sheremetyeva S.O., Osminin P.G. On methods and models of keyword automatic extraction. Bulletin of the South Ural State University. Series: Linguistics, 2015, vol. 12, no. 1, pp. 76–81. (in Russian)
9. Forsyth D. Applied Machine Learning. Springer, 2019, 494 p. doi: 10.1007/978-3-030-18114-7
10. Weiss K., Khoshgoftaar T.M., Wang D. A survey of transfer learning. Journal of Big Data, 2016, vol. 3, no. 1, pp. 9. doi: 10.1186/s40537-016-0043-6
11. Yosinski J., Clune J., Bengio Y., Lipson H. How transferable are features in deep neural networks? Advances in Neural Information Processing Systems, 2014, vol. 4, pp. 3320–3328.
12. Liu D., Tan Y., Khoram E., Yu Z. Training deep neural networks for the inverse design of nanophotonic structures. ACS Photonics, 2018, vol. 5, no. 4, pp. 1365–1369. doi: 10.1021/acsphotonics.7b01377
13. Krizhevsky A., Sutskever I., Hinton G.E. ImageNet classification with deep convolutional neural networks. Advances in Neural Information Processing Systems, 2012, vol. 2, pp. 1097–1105.
14. Müller A.C., Guido S. Introduction to Machine Learning with Python: A Guide for Data Scientists. O’Reilly Media, 2016, 400 p.
15. Berrar D., Lopes P., Dubitzky W. Incorporating domain knowledge in machine learning for soccer outcome prediction. Machine Learning, 2019, vol. 108, no. 1, pp. 97–126. doi: 10.1007/s10994-018-5747-8
16. Mohri M., Rostamizadeh A., Talwalkar A. Foundations of Machine Learning. Second Edition. Cambridge, MIT Press, 2018.
17. Alpaydin E. Machine Learning: The New AI. Cambridge, MIT Press, 2017, 206 p.