Menu
Publications
2025
2024
2023
2022
2021
2020
2019
2018
2017
2016
2015
2014
2013
2012
2011
2010
2009
2008
2007
2006
2005
2004
2003
2002
2001
Editor-in-Chief
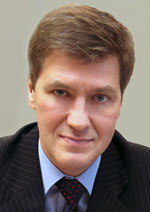
Nikiforov
Vladimir O.
D.Sc., Prof.
Partners
doi: 10.17586/2226-1494-2021-21-3-418-425
Mathematical modeling and identification of surface vessel model parameters
Read the full article

Article in Russian
For citation:
Abstract
For citation:
Nguyen Khac Tung, Vlasov S.M., Skobeleva A.V. Mathematical modeling and identification of surface vessel model parameters. Scientific and Technical Journal of Information Technologies, Mechanics and Optics, 2021, vol. 21, no. 3, pp. 418–425 (in Russian). doi: 10.17586/2226-1494-2021-21-3-418-425
Abstract
The paper considers the problems of modeling and identification of parameters for models of surface ships. The proposed identification method is applied to a modified second order Nomoto model for ship steering. The identification algorithm is based on the Dynamic Regressor Extension and Mixing Method (DREM) that is performed in two steps. At the first stage parameterization is used for a regression model, in which the regressor and regression depend on the measured signals, namely, longitudinal, lateral and angular velocities and steering angle. At the second stage a new regression model is built using linear stable filters and delays. Finally, the parameters are estimated by the standard gradient descent method. The paper proposes a new algorithm which identifies the parameters for models of surface ships. The authors analyzed the prospects of the proposed estimating method by computer experiments. Experiments have shown the advantage of the method: when using the gradient descent method, the transient time spent to estimate the signal parameters is much longer than using the DREM method. At the same time, in the case of using the DREM method, there is no overshoot. The results of the work can serve as a basis for methods, algorithms and software for designing ship automated navigation systems and control systems for other modes of transport. This is confirmed by the simulation results.
Keywords: surface ship, identification, Nomoto model, DREM, gradient descent method, regressor
References
References
1. Vlasov S.M., Kirsanova A.S., Dobriborsci D., Borisov O.I., Gromov V.S., Pyrkin A.A., Maltsev M.V., Semenev A.N. Output adaptive controller design for robotic vessel with parametric and functional uncertainties. Proc. 26th Mediterranean Conference on Control and Automation (MED), 2018, pp. 547–552. doi: 10.1109/MED.2018.8442738
2. Vlasov S.M., Borisov O.I., Gromov V.S., Pyrkin A.A., Bobtsov A.A. Algorithms of adaptive and robust output control for a robotic prototype of a surface vessel. Mekhatronika, Avtomatizatsiya, Upravlenie, 2016, vol. 17, no. 1, pp. 18–25. (in Russian). doi: 10.17587/mau.17.18-25
3. Vlasov S.M., Borisov O.I., Gromov V.S., Pyrkin A.A., Bobtsov A.A. Robust system of dynamic positioning for robotized model of surface craft. Journal of Instrument Engineering, 2015, vol. 58, no. 9, pp. 713–719. (in Russian). doi: 10.17586/0021-3454-2015-58-9-713-719
4. Wang J., Pyrkin A.A., Bobtsov A.A., Borisov O.I., Gromov V.S., Kolyubin S.A., Vlasov S.M. Output control algorithms of dynamic positioning and disturbance rejection for robotic vessel. IFAC-PapersOnline, 2015, vol. 48, no. 11, pp. 295–300. doi: 10.1016/j.ifacol.2015.09.201
5. Pyrkin A.A., Bobtsov A.A., Kolyubin S.A., Surov M.O., Vedyakov A.A., Feskov A.D., Vlasov S.M., Krasnov A.Y., Borisov O.I., Gromov V.S. Dynamic positioning system for nonlinear MIMO plants and surface robotic vessel. IFAC Proceedings Volumes (IFAC-PapersOnline), 2013, vol. 46, no. 9, pp. 1867–1872. doi: 10.3182/20130619-3-RU-3018.00287
6. Van Amerongen J., Udink Ten Cate A.J. Model reference adaptive autopilots for ships. Automatica, 1975, vol. 11, no. 5, pp. 441–449. doi: 10.1016/0005-1098(75)90020-5
7. Åström J., Källström C.G. Identification of ship steering dynamics. Automatica, 1976, vol. 12, no. 1, pp. 9–22. doi: 10.1016/0005-1098(76)90064-9
8. Sheikhot A.K. Improvement of Marine Vessel Control Systems on the Base of Identification and Adaptation. Dissertation for the degree of candidate of technical sciences. The Admiral Nevelskoy Maritime State University, 2008, 130 p. (in Russian)
9. Ma F.C., Tóng S.H. Real time parameters identification of ship dynamic using the Extended Kalman Filter and the second order filter. IEEE Conference on Control Applications – Proceedings, 2003, vol. 2, pp. 1245–1250. doi: 10.1109/cca.2003.1223189
10. Di Mascio A., Dubbioso G., Notaro C., Viviani M. Investigation of twin-screw naval ships maneuverability behavior. Journal of Ship Research, 2011, vol. 55, no. 4, pp. 221–248. doi: 10.5957/JOSR.55.4.090031
11. Rhee K.-P., Lee S.-Y., Sung Y.-J. Estimation of manoeuvring coefficients from PMM test by genetic algorithm. Proc. of the International Symposium and Workshop on Force Acting on a Manoeuvring Vessel, Val de Reuil, France, 1998, pp. 77–87.
12. Sutulo S., Guedes Soares C. An algorithm for offline identification of ship manoeuvring mathematical models from free-running tests. Ocean Engineering, 2014, vol. 79, pp. 10–25. doi: 10.1016/j.oceaneng.2014.01.007
13. Osokina E., Dmitriy O., Dyda A. Simple modles based nadaptive aidentification of ship parameters. Vestnik Gosudarstvennogo Universiteta Morskogo i Rechnogo Flota Imeni Admirala S.O. Makarova, 2015, no. 2, pp. 24–31. (in Russian). doi: 10.21821/2309-5180-2015-7-2-24-31
14. Muñoz-Mansilla R., Aranda J., Díaz J.M., de la Cruz J. Parametric model identification of high-speed craft dynamics. Ocean Engineering, 2009, vol. 36, no. 12-13, pp. 1025–1038. doi: 10.1016/j.oceaneng.2009.05.007
15. Alyshev A.S., Romaev D.V., Melnikov V.G., Titov A.M., Kovalenko A.E. Parametric identification of ship model by symmetric motions around roll angle. Scientific and Technical Journal of Information Technologies, Mechanics and Optics, 2019, vol. 19, no. 2, pp. 347–358. (in Russian). doi: 10.17586/2226-1494-2019-19-2-347-358
16. Moreira L., Guedes Soares C. Dynamic model of manoeuvrability using recursive neural networks. Ocean Engineering, 2003, vol. 30, no. 13, pp. 1669–1697. doi: 10.1016/S0029-8018(02)00147-6
17. Chiu F.C., Chang T.L., Go J., Chou S.-K., Chen W.-C. A recursive neural networks model for ship maneuverability prediction. Proc. of the Conference MTTS/IEEE TECHNO-OCEAN’04, 2004, vol. 3, pp. 1211–1218. doi: 10.1109/OCEANS.2004.1405752
18. Rajesh G., Bhattacharyya S.K. System identification for nonlinear maneuvering of large tankers using artificial neural network. Applied Ocean Research, 2008, vol. 30, no. 4, pp. 256–263. doi: 10.1016/j.apor.2008.10.003
19. Nomoto K., Taguchi T., Honda K., Hirano S. On the steering qualities of ships. International Shipbuilding Progress, 1957, vol. 4, no. 35, pp. 354–370. doi: 10.3233/ISP-1957-43504
20. Tzeng C., Chen J. Fundamental properties of linear ship steering dynamic models. Journal of Marine Science and Technology, 1999, vol. 7, no. 2, pp. 79–88.
21. Journée J.M.J. A Simple Method for determining the manoeuvring indices K and T from zigzag trial data. Report No. 0267. 1970.
22. Sutulo S., Guedes Soares C. Synthesis of experimental designs of maneuvering captive-model tests with a large number of factors. Journal of Marine Science and Technology, 2004, vol. 9, no. 1, pp. 32–42. doi: 10.1007/s00773-003-0169-z
23. Abkowitz M.A. Lectures on ship hydrodynamics steering and manoeuvrability. Tech. Rep. Hy-5. Hydro and Aerodynamics Laboratory, Lyngby, Denmark, 1964.
24. Caccia M., Bruzzone G., Bono R. A practical approach to modeling and identification of small autonomous surface craft. IEEE Journal of Oceanic Engineering, 2008, vol. 33, no. 2, pp. 133–145. doi: 10.1109/JOE.2008.920157
25. Fossen T.I. Marine Control Systems: Guidance, Navigation, and Control of Ships, Rigs and Underwater Vehicles. Trondheim, Norway, Marine Cybernetics, 2002, 570 p.
26. Vagushchenko L.L., Tcymbal N.N. Automatic Ship Motion Control Systems. Odessa, Fenіks, 2007, 328 p. (in Russian)
27. Davidson K.S.M., Schiff L.I. Turning and course keeping qualities. Transactions of SNAME, 1946, vol. 54, pp. 189–190.
28. Aranovskiy S., Bobtsov A., Ortega R., Pyrkin A. Parameters estimation via dynamic regressor extension and mixing. Proceedings of the American Control Conference, 2016, pp. 6971–6976. doi: 10.1109/ACC.2016.7526771
29. Le Van Tuana, Bobtsov A.A., Pyrkin A.A. New algorithm of variable parameters identification for linear regression model. Scientific and Technical Journal of Information Technologies, Mechanics and Optics, 2017, vol. 17, no. 5, pp. 952–955. (in Russian). doi: 10.17586/2226-1494-2017-17-5-952-955