Menu
Publications
2025
2024
2023
2022
2021
2020
2019
2018
2017
2016
2015
2014
2013
2012
2011
2010
2009
2008
2007
2006
2005
2004
2003
2002
2001
Editor-in-Chief
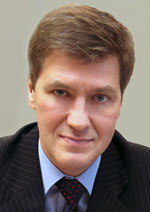
Nikiforov
Vladimir O.
D.Sc., Prof.
Partners
doi: 10.17586/2226-1494-2021-21-3-433-436
Automatic allergy classification based on Russian unstructured medical texts
Read the full article

Article in Russian
For citation:
Abstract
For citation:
Lenivtceva Iu.D., Kopanitsa G.D. Automatic allergy classification based on Russian unstructured medical texts. Scientific and Technical Journal of Information Technologies, Mechanics and Optics, 2021, vol. 21, no. 3, pp. 433–436 (in Russian). doi: 10.17586/2226-1494-2021-21-3-433-436
Abstract
Most of the medical data in hospital information systems databases are stored in an unstructured form. Techniques for processing unstructured records are widely presented in scientific papers focused on English data. This paper proposes a method for intellectual analysis of unstructured allergy anamnesis in Russian in order to identify the presence and type of allergy and intolerance of a patient. The method is based on machine learning algorithms and uses international standards for the exchange of medical data and terminology standards, such as FHIR and SNOMED CT. As a result of the experiment, about 12 thousand medical records were processed. F-measure for the developed classification models ranged from 0.93 to 0.96. The models showed high values of metrics for evaluating the effectiveness of the models. In the future, structured data can be used in models for predicting medical risks. Further development of methods for structuring medical texts will ensure the interoperability of medical data.
Keywords: medical data structuring, allergy, intolerance, machine learning, unstructured text analysis, interoperability
References
References
1. Lenivtceva I.D., Kopanitsa G. Evaluating manual mappings of Russian proprietary formats and terminologies to FHIR. Methods of Information in Medicine, 2019, vol. 58, no. 4-5, pp. 151–159. doi: 10.1055/s-0040-1702154
2. Fung K.W., Xu J., Rosenbloom S.T., Campbell J.R. Using SNOMED CT-encoded problems to improve ICD-10-CM coding—A randomized controlled experiment. International Journal of Medical Informatics, 2019, vol. 126, pp. 19–25. doi: 10.1016/j.ijmedinf.2019.03.002
3. Fiebeck J., Gietzelt M., Ballout S., Christmann M., Fradziak M., Laser H., Ruppel J., Schönfeld N., Teppner S., Gerbel S. Implementing LOINC: Current status and ongoing work at the Hannover Medical School. Studies in Health Technology and Informatics, 2019, vol. 258, pp. 247–248. doi: 10.3233/978-1-61499-959-1-247
4. Mascia C., Uva P., Leo S., Zanetti G. OpenEHR modeling for genomics in clinical practice. International Journal of Medical Informatics, 2018, vol. 120, pp. 147–156. doi: 10.1016/j.ijmedinf.2018.10.007
5. Santos M.R., Bax M.P., Kalra D. Building a logical EHR architecture based on ISO 13606 standard and semantic web technologies. Studies in Health Technology and Informatics, 2010, vol. 160, no. 1, pp. 161–165. doi: 10.3233/978-1-60750-588-4-161
6. Ulrich H., Kock A.-K., Duhm-Harbeck P., Habermann J.K., Ingenerf J. Metadata repository for improved data sharing and reuse based on HL7 FHIR. Studies in Health Technology and Informatics, 2017, vol. 228, pp. 162–166. doi: 10.3233/978-1-61499-678-1-162
7. Hong N., Wen A., Mojarad M.R., Sohn S., Liu H., Jiang G. Standardizing heterogeneous annotation corpora using HL7 FHIR for facilitating their reuse and integration in clinical NLP. AMIA Annual Symposium Proceedings. American Medical Informatics Association, 2018, vol. 2018, pp. 574–583.
8. Dudchenko A., Dudchenko P., Ganzinger M., Kopanitsa G. Extraction from medical records. Studies in Health Technology and Informatics, 2019, vol. 261, pp. 62–67. doi: 10.3233/978-1-61499-975-1-62
9. Oleynik M., Kugic A., Kasáč Z., Kreuzthaler M. Evaluating shallow and deep learning strategies for the 2018 n2c2 shared task on clinical text classification. Journal of the American Medical Informatics Association, 2019, vol. 26, no. 11, pp. 1247–1254. doi: 10.1093/jamia/ocz149