Menu
Publications
2025
2024
2023
2022
2021
2020
2019
2018
2017
2016
2015
2014
2013
2012
2011
2010
2009
2008
2007
2006
2005
2004
2003
2002
2001
Editor-in-Chief
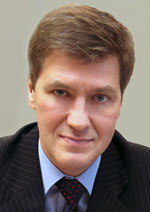
Nikiforov
Vladimir O.
D.Sc., Prof.
Partners
doi: 10.17586/2226-1494-2021-21-4-463-472
Nature-inspired metaheuristic scheduling algorithms in cloud: a systematic review
Read the full article

Article in English
For citation:
Abstract
For citation:
Bothra S.K., Singhal S. Nature-inspired metaheuristic scheduling algorithms in cloud: a systematic review. Scientific and Technical Journal of Information Technologies, Mechanics and Optics, 2021, vol. 21, no. 4, pp. 463–472.
doi: 10.17586/2226-1494-2021-21-4-463-472
Abstract
Complex huge-scale scientific applications are simplified by workflow to execute in the cloud environment. The cloud is an emerging concept that effectively executes workflows, but it has a range of issues that must be addressed for it to progress. Workflow scheduling using a nature-inspired metaheuristic algorithm is a recent central theme in the cloud computing paradigm. It is an NP-complete problem that fascinates researchers to explore the optimum solution using swarm intelligence. This is a wide area where researchers work for a long time to find an optimum solution but due to the lack of actual research direction, their objectives become faint. Our systematic and extensive analysis of scheduling approaches involves recently high-cited metaheuristic algorithms like Genetic Algorithms (GA), Whale Search Algorithm (WSA), Ant Colony Optimization (ACO), Bat Algorithm, Artificial Bee Colony (ABC), Cuckoo Algorithm, Firefly Algorithm and Particle Swarm Optimization (PSO). Based on various parameters, we do not only classify them but also furnish a comprehensive striking comparison among them with the hope that our efforts will assist recent researchers to select an appropriate technique for further undiscovered issues. We also draw the attention of present researchers towards some open issues to dig out unexplored areas like energy consumption, reliability and security for considering them as future research work.
Keywords: genetic algorithm, literature review, nature inspired algorithm, metaheuristic scheduling algorithm, swarm intelligence
References
References
-
Ibrahim S., He B., Jin H. Towards pay-as-you-consume cloud computing. Proc. of the IEEE International Conference on Services Computing (SCC), 2011, pp. 370–377. https://doi.org/10.1109/scc.2011.38
-
Kumar P., Kumar R. Issues and challenges of load balancing techniques in cloud computing: A survey. ACM Computing Surveys, 2019, vol. 51, no. 6, pp. 120. https://doi.org/10.1145/3281010
-
Aljoumah E., Al-Mousawi F., Ahmad I., Al-Shammri M., Al-Jady Z. SLA in cloud computing architectures: A comprehensive study. International Journal of Grid and Distributed Computing, 2015, vol. 8, no. 5, pp. 7–32. https://doi.org/10.14257/ijgdc.2015.8.5.02
-
Weinman J. Cloud computing is NP-complete. Proc. Tech. Symp. ITU Telecom World, 2011, pp. 75–81.
-
Fister Jr, I., Yang X.S., Fister I., Brest J., Fister D. A brief review of nature-inspired algorithms for optimization. Elektrotehniski Vestnik, 2013, vol. 80, no. 3, pp. 116–122.
-
Osman I.H., Kelly J.P. Meta-heuristics: an overview. Meta-Heuristics, Springer, 1996, pp. 1–21. https://doi.org/10.1007/978-1-4613-1361-8_1
-
Laporte G., Osman I.H. Routing problems: A bibliography. Annals of Operations Research, 1995, vol. 61, no. 1, pp. 227–262. https://doi.org/10.1007/bf02098290
-
Xu R., Wang Y., Cheng Y., Zhu Y., Xie Y., Sani A.S., Yuan D. Improved particle swarm optimization based workflow scheduling in cloud-fog environment. Lecture Notes in Business Information Processing, 2018, vol. 342, pp. 337–347. https://doi.org/10.1007/978-3-030-11641-5_27
-
Shubham, Gupta R., Gajera V., Jana P.K. An effective multi-objective workflow scheduling in cloud computing: A PSO based approach. Proc. 9th International Conference on Contemporary Computing (IC3 2016), 2016, pp. 7880196. https://doi.org/10.1109/ic3.2016.7880196
-
Zhan S., Huo H. Improved PSO-based task scheduling algorithm in cloud computing. Journal of Information & Computational Science, 2012, vol. 9, no. 13, pp. 3821–3829.
-
Chen Z., Zhan Z., Lin Y., Gong Y., Gu T., Zhao F., Yuan H., Chen X., Li Q., Zhang J. Multiobjective cloud workflow scheduling: A multiple populations ant colony system approach. IEEE Transactions on Cybernetics, 2019, vol. 49, no. 8, pp. 2912–2926. https://doi.org/10.1109/tcyb.2018.2832640
-
Lal A., Krishna C.R. Critical path-based ant colony optimization for scientific workflow scheduling in cloud computing under deadline constraint. Advances in Intelligent Systems and Computing, 2018, vol. 696, pp. 447–461. https://doi.org/10.1007/978-981-10-7386-1_39
-
Sharma S., Jain R. EACO: an enhanced ant colony optimization algorithm for task scheduling in cloud computing. International Journal of Security and Its Applications, 2019, vol. 13, no. 4, pp. 91–100. https://doi.org/10.33832/ijsia.2019.13.4.09
-
Meshkati J., Safi-Esfahani F. Energy-aware resource utilization based on particle swarm optimization and artificial bee colony algorithms in cloud computing. Journal of Supercomputing, 2019, vol.75, no. 5, pp. 2455–2496. https://doi.org/10.1007/s11227-018-2626-9
-
Thanka M.R., Maheswari P.U., Edwin E.B. An improved efficient: Artificial Bee Colony algorithm for security and QoS aware scheduling in cloud computing environment. Cluster Computing, 2019, vol. 22, no. 5, pp. 10905–10913. https://doi.org/10.1007/s10586-017-1223-7
-
Yang X.S. Multiobjective firefly algorithm for continuous optimization. Engineering with Computers, 2013, vol. 29, no. 2, pp. 175–184. https://doi.org/10.1007/s00366-012-0254-1
-
Chakravarthi K.K., Shyamala L., Vaidehi V. Cost-effective workflow scheduling approach on cloud under deadline constraint using firefly algorithm. Applied Intelligence, 2021, vol. 51, no. 3, pp. 1629–1644. https://doi.org/10.1007/s10489-020-01875-1
-
Pradeep K., Jacob T.P. A hybrid approach for task scheduling using the cuckoo and harmony search in cloud computing environment. Wireless Personal Communications, 2018, vol. 101, no. 4, pp. 2287–2311. https://doi.org/10.1007/s11277-018-5816-0
-
Ghasemi S., Hanani A. A cuckoo-based workflow scheduling algorithm to reduce cost and increase load balance in the cloud environment. International Journal on Informatics Visualization, 2019, vol. 3, no. 1, pp. 79–85. https://doi.org/10.30630/joiv.3.1.220
-
Masadeh R., Sharieh A., Mahafzah B. Humpback whale optimization algorithm based on vocal behavior for task scheduling in cloud computing. International Journal of Advanced Science and Technology, 2019, vol. 13, no. 3, pp. 121–140.
-
Thennarasu S.R., Selvam M., Srihari K. A new whale optimizer for workflow scheduling in cloud computing environment. Journal of Ambient Intelligence and Humanized Computing, 2021, vol. 12, no. 3, pp. 3807–3814. https://doi.org/10.1007/s12652-020-01678-9
-
Hemasian-Etefagh F., Safi-Esfahani F. Dynamic scheduling applying new population grouping of whales meta-heuristic in cloud computing. Journal of Supercomputing, 2019, vol. 75, no. 10, pp. 6386–6450. https://doi.org/10.1007/s11227-019-02832-7
-
Kaur N., Singh S. A budget-constrained time and reliability optimization bat algorithm for scheduling workflow applications in clouds. Procedia Computer Science, 2016, vol. 98, pp. 199–204. https://doi.org/10.1016/j.procs.2016.09.032
-
Gu Y., Budati C. Energy-aware workflow scheduling and optimization in clouds using bat algorithm. Future Generation Computer Systems, 2020, vol. 113, pp. 106–112. https://doi.org/10.1016/j.future.2020.06.031
-
Ullah A., Nawi N.M., Khan M.H. BAT algorithm used for load balancing purpose in cloud computing: an overview. International Journal of High Performance Computing and Networking, 2020, vol. 16, no. 1, pp. 43–54. https://doi.org/10.1504/ijhpcn.2020.110258
-
Abdel-Basset M., Abdel-Fatah L., Sangaiah A.K. Metaheuristic algorithms: A comprehensive review. Computational Intelligence for Multimedia Big Data on The Cloud With Engineering Applications, 2018, pp. 185–231. https://doi.org/10.1016/b978-0-12-813314-9.00010-4
-
Yang X.S. Nature-Inspired Metaheuristic Algorithms. Luniver press, 2010, 148 p.
-
Iranmanesh A., Naji H.R. DCHG-TS: a deadline-constrained and cost-effective hybrid genetic algorithm for scientific workflow scheduling in cloud computing. Cluster Computing, 2021, vol. 24, no. 2, pp. 667–681. https://doi.org/10.1007/s10586-020-03145-8
-
Zhou Z., Li F., Zhu H., Xie H., Abawajy J.H., Chowdhury M.U. An improved genetic algorithm using greedy strategy toward task scheduling optimization in cloud environments. Neural Computing and Applications, 2020, vol. 32, no. 6, pp. 1531–1541. https://doi.org/10.1007/s00521-019-04119-7
-
Aziza H., Krichen S. A hybrid genetic algorithm for scientific workflow scheduling in cloud environment. Neural Computing and Applications, 2020, vol. 32, no. 18, pp. 15263–15278. https://doi.org/10.1007/s00521-020-04878-8
-
Sardaraz M., Tahir M. A parallel multi-objective genetic algorithm for scheduling scientific workflows in cloud computing. International Journal of Distributed Sensor Networks, 2020, vol. 16, no. 8. https://doi.org/10.1177/1550147720949142
-
Pang S., Li W., He H., Shan Z., Wang X. An EDA-GA hybrid algorithm for multi-objective task scheduling in cloud computing. IEEE Access, 2019, vol. 7, pp. 146379–146389. https://doi.org/10.1109/access.2019.2946216
-
Wangsom P., Lavangnananda K., Bouvry P. Multi-objective scientific-workflow scheduling with data movement awareness in cloud. IEEE Access, 2019, vol. 7, pp. 177063–177081. https://doi.org/10.1109/access.2019.2957998
-
Hammed S.S., Arunkumar B. Efficient workflow scheduling in cloud computing for security maintenance of sensitive data. International Journal of Communication Systems, 2019, pp. e4240. https://doi.org/10.1002/dac.4240
-
Gupta I., Gupta S., Choudhary A., Jana P.K. A hybrid meta-heuristic approach for load balanced workflow scheduling in IaaS cloud. Lecture Notes in Computer Science, 2019, vol. 11319, pp. 73–89. https://doi.org/10.1007/978-3-030-05366-6_6
-
Rehman A., Hussain S.S., ur Rehman Z., Zia S., Shamshirband S. Multi-objective approach of energy efficient workflow scheduling in cloud environments. Concurrency and Computation: Practice and Experience, 2019, vol. 31, no. 8, pp. e4949. https://doi.org/10.1002/cpe.4949
-
Ashouraei M., Khezr S.N., Benlamri R., Navimipour N.J. A new SLA-aware load balancing method in the cloud using an improved parallel task scheduling algorithm. Proc. 6th International Conference on Future Internet of Things and Cloud (FiCloud), 2018, pp. 71–76. https://doi.org/10.1109/ficloud.2018.00018
-
Jana B., Poray J. A hybrid task scheduling approach based on genetic algorithm and particle swarm optimization technique in cloud environment. Advances in Intelligent Systems and Computing, 2018, vol. 695, pp. 607–614. https://doi.org/10.1007/978-981-10-7566-7_61
-
Shishido H.Y., Estrella J.C., Toledo C.F.M., Arantes M.S. Genetic-based algorithms applied to a workflow scheduling algorithm with security and deadline constraints in clouds. Computers & Electrical Engineering, 2018, vol. 69, pp. 378–394. https://doi.org/10.1016/j.compeleceng.2017.12.004
-
Kaur G., Kalra M. Deadline constrained scheduling of scientific workflows on cloud using hybrid genetic algorithm. Proc. 7th International Conference on Cloud Computing, Data Science and Engineering, Confluence, 2017, pp. 276–280. https://doi.org/10.1109/confluence.2017.7943162
-
Wangsom P., Lavangnananda K., Bouvry P. The application of nondominated sorting genetic algorithm (NSGA-III) for scientific-workflow scheduling on cloud. Proc. 8th Multidisciplinary International Conference on Scheduling: Theory and Applications (MISTA 2017), 2017, pp. 269–287.
-
Meena J., Kumar M., Vardhan M. Cost effective genetic algorithm for workflow scheduling in cloud under deadline constraint. IEEE Access, 2016, vol. 4, pp. 5065–5082. https://doi.org/10.1109/access.2016.2593903
-
Liu L., Zhang M., Buyya R., Fan Q. Deadline‐constrained coevolutionary genetic algorithm for scientific workflow scheduling in cloud computing. Concurrency and Computation: Practice and Experience, 2017, vol. 29, no. 5, pp. e3942. https://doi.org/10.1002/cpe.3942
-
Chen Z.G., Du K.J., Zhan Z.H., Zhang J. Deadline constrained cloud computing resources scheduling for cost optimization based on dynamic objective genetic algorithm. Proc. 2015 IEEE Congress on Evolutionary Computation (CEC), 2015, pp. 708–714. https://doi.org/10.1109/cec.2015.7256960
-
Xu Y., Li K., Hu J., Li K. A genetic algorithm for task scheduling on heterogeneous computing systems using multiple priority queues. Information Sciences, 2014, vol. 270, pp. 255–287. https://doi.org/10.1016/j.ins.2014.02.122
-
Delavar A.G., Aryan Y. HSGA: a hybrid heuristic algorithm for workflow scheduling in cloud systems. Cluster Computing, 2014, vol. 17, no. 1, pp. 129–137. https://doi.org/10.1007/s10586-013-0275-6
-
Wang T., Liu Z., Chen Y., Xu Y., Dai X. Load balancing task scheduling based on genetic algorithm in cloud computing. Proc. 12th International Conference on Dependable, Autonomic and Secure Computing (DASC), 2014, pp. 146–152. https://doi.org/10.1109/DASC.2014.35
-
Dasgupta K., Mandal B., Dutta P., Mandal J.K., Dam S. A genetic algorithm (GA) based load balancing strategy for cloud computing. Procedia Technology, 2013, vol. 10. pp. 340–347. https://doi.org/10.1016/j.protcy.2013.12.369
-
Ying C.-T., Yu J. Energy-aware genetic algorithms for task scheduling in cloud computing. Proc. 7th ChinaGrid Annual Conference (ChinaGrid 2012), 2012, pp. 43–48. https://doi.org/10.1109/chinagrid.2012.15
-
Zhao E.-D., Qi Y.-Q., Xiang X.-X., Chen Y. A data placement strategy based on genetic algorithm for scientific workflows. Proc. 8th International Conference on Computational Intelligence and Security (CIS 2012), 2012, pp. 146–149. https://doi.org/10.1109/cis.2012.40
-
Barrett E., Howley E., Duggan J. A learning architecture for scheduling workflow applications in the cloud. Proc. 9th European Conference on Web Services (ECOWS 2011), 2011, pp. 83–90. https://doi.org/10.1109/ecows.2011.27
-
Kessaci Y., Melab N., Talbi E.-G. A pareto-based GA for scheduling HPC applications on distributed cloud infrastructures. Proc. of the International Conference on High Performance Computing and Simulation (HPCS 2011), 2011, pp. 456–462. https://doi.org/10.1109/hpcsim.2011.5999860
-
Singhal S., Patel J. Load balancing scheduling algorithm for concurrent workflow. Computing and Informatics, 2018, vol. 37, no. 2, pp. 311–326. https://doi.org/10.4149/cai_2018_2_311
-
Bansal S., Hota C. Efficient Algorithm on heterogeneous computing system. Proc. of the International Conference on Recent Trends in Information Systems (ReTIS 2011), 2011, pp. 57–61. https://doi.org/10.1109/retis.2011.6146840
-
Khoruzhnikov S.E., Shevel A.Y. Management system for scalable geographically distributed data center. Scientific and Technical Journal of Information Technologies, Mechanics and Optics, 2019, vol. 19, no. 5, pp. 931–938. (in Russian). https://doi.org/10.17586/2226-1494-2019-19-5-931-938
-
Becker M., Gatchin Yu., Karmanovsky N., Terentiev A., Fyodorov D. Information security in cloud computing: problems and prospects. Scientific and Technical Bulletin of St. Petersburg State University of Information Technologies, Mechanics and Optics, 2011, no. 1(71), pp. 97–102. (in Russian)
-
Khan D.V., Razgulyaev K.A., Tyagunov D.M. Web portals for management of cloud services within data centres. Scientific and Technical Journal of Information Technologies, Mechanics and Optics, 2019, vol. 19, no. 6, pp. 1169–1171. (in Russian). https://doi.org/10.17586/2226-1494-2019-19-6-1169-1171