Menu
Publications
2025
2024
2023
2022
2021
2020
2019
2018
2017
2016
2015
2014
2013
2012
2011
2010
2009
2008
2007
2006
2005
2004
2003
2002
2001
Editor-in-Chief
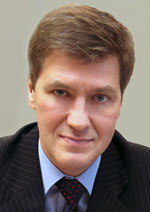
Nikiforov
Vladimir O.
D.Sc., Prof.
Partners
doi: 10.17586/2226-1494-2021-21-4-592-598
Modeling security violation processes in machine learning systems
Read the full article

Article in Russian
For citation:
Abstract
For citation:
Chekmarev M.A., Klyuev S.G., Shadskiy V.V. Modeling security violation processes in machine learning systems. Scientific and Technical Journal of Information Technologies, Mechanics and Optics, 2021, vol. 21, no. 4, pp. 592–598 (in Russian). doi: 10.17586/2226-1494-2021-21-4-592-598.
Abstract
The widespread use of machine learning, including at critical information infrastructure facilities, entails risks of security threats in the absence of reliable means of protection. The article views the processes in machine learning systems as the ones occurring in information systems susceptible to malicious influences. The results of modeling events leading to a security breach in machine learning systems operating at critical information infrastructure facilities are presented. For modeling, the technology of creating functional models SADT (Structured Analysis and Design Technique) and the IDEF0 (Integration definition for function modeling) methodology were used as a tool for transition from a verbal functional description of the process under study to a description in terms of mathematical representation. In order to study the scenarios of the transition of machine learning systems to a dangerous state and the numerical assessment of the probability of security violation, mathematical modeling of threats was carried out using the logical-probabilistic method. The authors obtained a visual functional model of system security violation in the form of a context diagram of the system and two levels of decomposition. The hazard function of the system is determined and the arithmetic polynomial of the probability function is derived. In further work the described models will allow researchers to develop methods and algorithms for protecting machine learning systems from malicious influences, as well as to apply them in assessing the level of security.
Keywords: machine learning, security breach, integrity, confidentiality, functional modeling, logical probabilistic modeling
Acknowledgements. The work was carried out at the Krasnodar Higher Military School as part of a dissertation research in the field of ensuring the security of machine learning systems
References
Acknowledgements. The work was carried out at the Krasnodar Higher Military School as part of a dissertation research in the field of ensuring the security of machine learning systems
References
-
Nelson B., Barreno M., Chi F.J., Joseph A.D., Rubinstein B.I.P., Saini U., Sutton C., Tygar J.D., Xia K. Exploiting machine learning to subvert your spam filter. Proc. of First USENIX Workshop on Large-Scale Exploits and Emergent Threats, 2008. Available at: https://people.eecs.berkeley.edu/~tygar/papers/SML/Spam_filter.pdf(accessed: 25.03.2021).
-
Biggio B., Nelson B., Laskov P. Poisoning attacks against support vector machines. Proc. of the 29th International Conference on Machine Learning (ICML 2012), 2012, pp. 1807–1814. Available at: https://icml.cc/2012/papers/880.pdf (accessed: 25.03.2021).
-
Steinhardt J., Koh P.W., Liang P. Certified defenses for data poisoning attacks. Advances in Neural Information Processing Systems, 2017, vol. 30, pp. 3518–3530.
-
Goodfellow I.J., Shlens J., Szegedy C. Explaining and harnessing adversarial examples. Proc. of the 3rd International Conference on Learning Representations, 2015.
-
Sharif M., Bhagavatula S., Bauer L., Reiter M.K. Accessorize to a crime: Real and stealthy attacks on state-of-the-art face recognition. Proc. of the 23th ACM Conference on Computer and Communications Security (CCS), 2016. Available at: https://www.cs.cmu.edu/~sbhagava/papers/face-rec-ccs16.pdf(accessed: 11.04.2021). https://doi.org/10.1145/2976749.2978392
-
TramèrF., ZhangF., JuelsA., ReiterM.K., RistenpartT. Stealing machine learning models via prediction APIs. Proc. of the 25th USENIX Conference on Security Symposium, 2016, pp. 601–608.
-
Carlini N., Liu C., Erlingsson Ú., Kos J., Song D. The secret sharer: Evaluating and testing unintended memorization in neural networks. Proc. of the 28th USENIX Security Symposium, 2019, pp. 267–284.
-
AtenieseG., ManciniL.V.,SpognardiA., VillaniA., VitaliD., FeliciG. Hacking smart machines with smarter ones: How to extract meaningful data from machine learning classifiers. International Journal of Security and Networks, 2015, vol. 10, no. 3, pp. 137–150. https://doi.org/10.1504/IJSN.2015.071829
-
Fredrikson M., Jha S., Ristenpart T. Model inversion attacks that exploit confidence information and basic countermeasures. Proc. of the 22nd ACM SIGSAC Conference on Computer and Communications Security, 2015, pp. 1322–1333 Available at: https://rist.tech.cornell.edu/papers/mi-ccs.pdf(accessed: 25.03.2021).https://doi.org/10.1145/2810103.2813677
-
Fredrikson M., Lantz E., Jha S., Lin S., Page D., Ristenpart T. Privacy in pharmacogenetics: An end-to-end case study of personalized warfarin dosing. Proc. of the 23rd USENIX Security Symposium, 2014, pp. 17–32. Available at: https://www.usenix.org/system/files/conference/usenixsecurity14/sec14-paper-fredrikson-privacy.pdf(accessed: 11.04.2021).
-
ZapechnikovS.V. Modelsandalgorithmsofprivacy-preservingmachinelearning. IT Security (Russia), 2020, vol. 27, no. 1, pp. 51–67. (in Russian). https://doi.org/10.26583/bit.2020.1.05
-
FinkoO.A., SokolovskyE.P. Risk assessment information security algorithm is based on the I. Ryabinin logical-probabilistic method. Izvestiya SFedU. Engineering Sciences, 2013, no. 12(149), pp. 172–178. (in Russian)
-
Riabinin I.A. Reliability and Safety of Structural Complex Systems. St. Petersburg, Politehnika Publ., 2000, 248 p. (in Russian)
-
Kolmogorov A.N., Dragalin A.G. Mathematical logic: Introduction to the Mathematical Logic. Moscow, LENAND Publ., 2017, 240 p. (in Russian)
-
Mkhitarian V.S., Astafeva E.V., Mironkina Iu.N., Troshin L.I. Probability Theory and Mathematical Statistics. Moscow, SynergyUniversityPubl., 2013, 336 p. (in Russian)
-
Finko O. Modular Arithmetic of Parallel Logic Computing. Ed by V.D. Maliugin. Moscow, Institute of Control Sciences of the Russian Academy of Sciences Publ., 2003, 224 p. (in Russian)