Menu
Publications
2025
2024
2023
2022
2021
2020
2019
2018
2017
2016
2015
2014
2013
2012
2011
2010
2009
2008
2007
2006
2005
2004
2003
2002
2001
Editor-in-Chief
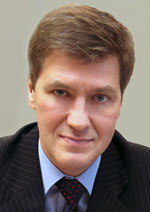
Nikiforov
Vladimir O.
D.Sc., Prof.
Partners
doi: 10.17586/2226-1494-2021-21-5-755-766
Protecting facial images from recognition on social media: solution methods and their perspective
Read the full article

Article in Russian
For citation:
Abstract
For citation:
Kukharev G.A., Maulenov K.S., Shchegoleva N.L. Protecting facial images from recognition on social media: solution methods and their perspective. Scientific and Technical Journal of Information Technologies, Mechanics and Optics, 2021, vol. 21, no. 5, pp. 755–766 (in Russian). doi: 10.17586/2226-1494-2021-21-5-755-766
Abstract
The paper deals with the problem of unauthorized use in deep learning of facial images from social networks and analyses methods of protecting such images from their use and recognition based on de-identification procedures and the newest of them — the “Fawkes” procedure. The proposed solution uses a comparative analysis of images subjected to the Fawkes-transformation procedure, representation and description of textural changes and features of structural damage in facial images. Multilevel parametric estimates of these damages were applied for their formal and numerical assessment. The reasons for the impossibility of using images of faces destroyed by the Fawkes procedure in deep learning tasks are explained. It has been theoretically proven and experimentally shown that facial images subjected to the Fawkes procedure are well recognized outside of deep learning methods. It is argued that the use of simple preprocessing methods for facial images (subjected to the Fawkes procedure) at the entrance to convolutional neural networks can lead to their recognition with high efficiency, which destroys the myth about the importance of protecting facial images with the Fawkes-procedure.
Keywords: social networks, unauthorized access, deep learning, face image protection, de-identification, Fawkes procedure, deterministic recognition methods
References
References
1. Schegoleva N.L. Face image models for criminalistics. Proceedings of Saint Petersburg Electrotechnical University Journal, 2016, no. 7, pp. 37–47. (in Russian)
2. Newton E.M., Sweeney L., Malin B. Preserving privacy by de-identifying face images. IEEE Transactions on Knowledge and Data Engineering, 2005, vol. 17, no. 2, pp. 232–243. https://doi.org/10.1109/TKDE.2005.32
3. Jourabloo A., Yin X., Liu X. Attribute preserved face de-identification. Proc. 8th International Conference on Biometrics (ICB), 2015, pp. 278–285. https://doi.org/10.1109/ICB.2015.7139096
4. Kukharev G., Oleinik A. Face photo-sketch transformation and population generation. Lecture Notes in Computer Science, 2016, vol. 9972, pp. 329–340. https://doi.org/10.1007/978-3-319-46418-3_29
5. Wu Y., Yang F., Xu Y., Ling H. Privacy-protective-GAN for privacy preserving face de-identification. Journal of Computer Science and Technology, 2019, vol. 34, no. 1, pp. 47–60. https://doi.org/10.1007/s11390-019-1898-8
6. Nousi P., Papadopoulos S., Tefas A., Pitas I. Deep autoencoders for attribute preserving face de-identification. Journal Signal Processing: Image Communication, 2020, vol. 81, pp. 115699. https://doi.org/10.1016/j.image.2019.115699
7. Shan S., Wenger E., Zhang J., Li H., Zheng H., Zhao B.Y. Fawkes: Protecting privacy against unauthorized deep learning models. Proc. 29th USENIX Security Symposium, 2020, pp. 1589–1604.
8. Wang Z., Bovik A.C., Sheikh H.R., Simoncelli E.P. Image quality assessment: from error visibility to structural similarity. IEEE Transactions on Image Processing, 2004, vol. 13, no. 4, pp. 600–612. https://doi.org/10.1109/TIP.2003.819861
9. Kukharev G.A., Kamenskaya E.I., Matveev Y.N., Shchegoleva N.L. Methods for Face Image Processing and Recognition in Biometric Applications. St. Petersburg, Politekhnika Publ., 2013, 388 p. (in Russian)
10. De Vel O., Aeberhard S. Line-based face recognition under varying pose. IEEE Transactions on Pattern Analysis and Machine Intelligence, 1999, vol. 21, no. 10, pp. 1081–1088. https://doi.org/10.1109/34.799912
11. Kukharev G.A., Shchegoleva N.L. Algorithms of two-dimensional projection of digital images in eigensubspace: History of development, implementation and application. Pattern Recognition and Image Analysis, 2018, vol. 28, no. 2, pp. 185–206. https://doi.org/10.1134/S1054661818020116
12. Kazemi V., Sullivan J. One millisecond face alignment with an ensemble of regression trees. Proc. 27th IEEE Conference on Computer Vision and Pattern Recognition (CVPR), 2014, pp. 1867–1874. https://doi.org/10.1109/CVPR.2014.241
13. Evtimov I., Sturmfels P., Kohno T. FoggySight: A Scheme for facial lookup privacy. Proceedings on Privacy Enhancing Technologies, 2021, vol. 3, pp. 204–226. https://doi.org/10.2478/popets-2021-0044