Menu
Publications
2024
2023
2022
2021
2020
2019
2018
2017
2016
2015
2014
2013
2012
2011
2010
2009
2008
2007
2006
2005
2004
2003
2002
2001
Editor-in-Chief
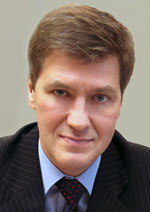
Nikiforov
Vladimir O.
D.Sc., Prof.
Partners
doi: 10.17586/2226-1494-2021-21-5-791-794
The architecture of a system for full-text search by speech data based on a global search index
Read the full article

Article in Russian
For citation:
Abstract
For citation:
Petrov O.E. The architecture of a system for full-text search by speech data based on a global search index. Scientific and Technical Journal of Information Technologies, Mechanics and Optics, 2021, vol. 21, no. 5, pp. 791–794 (in Russian). doi: 10.17586/2226-1494-2021-21-5-791-794
Abstract
This paper presents the architecture of a system for full-text search by speech data based on a global search index that combines information about all speech recordings in the archive. The architecture includes two independent blocks: an indexing block, and a block for building and performing a search query. In order to process speech recordings, it uses an automatic speech recognition system (ASR) with a linguistic decoder based on weighted finite-state transducers framework (WFST), which generates word lattices. Lattices are sequentially converted to confusion networks and inverse indexes. It allows taking into account all the word hypotheses generated during decoding. The proposed solution expands the applicability of speech analytics systems for those cases when the word error rate is high, such as the processing of speech recordings collected under difficult acoustic conditions or in low-resource languages.
Keywords: full-text search, speech analytics, spoken term detection, search index, automatic speech recognition
References
References
1. Zobel J., Moffat A. Inverted files for text search engines. ACM Computing Surveys, 2006, vol. 38, no. 2, pp. 6–es. https://doi.org/10.1145/1132956.1132959
2. Saon G., Povey D., Zweig G. Anatomy of an extremely fast LVCSR decoder. Proc. 9th European Conference on Speech Communication and Technology, 2005, pp. 549–552. https://doi.org/10.21437/Interspeech.2005-338
3. Mohri M., Pereira F., Riley M. Weighted finite-state transducers in speech recognition. Computer Speech and Language, 2002, vol. 16, no. 1, pp. 69–88. https://doi.org/10.1006/csla.2001.0184
4. Laptev A., Andrusenko A., Podluzhny I., Mitrofanov A., Medennikov I., Matveev Y. Dynamic acoustic unit augmentation with BPE-dropout for low-resource end-to-end speech recognition. Sensors, 2021, vol. 21, no. 9, pp. 3063. https://doi.org/10.3390/s21093063
5. Mangu L., Brill E., Stolcke A. Finding consensus in speech recognition: word error minimization and other applications of confusion networks. Computer Speech and Language, 2000, vol. 14, no. 4, pp. 373–400. https://doi.org/10.1006/csla.2000.0152
6. Lagogiannis G. Query-optimal partially persistent B-trees with constant worst-case update time. International Journal of Foundations of Computer Science, 2017, vol. 28, no. 2, pp. 141–169. https://doi.org/10.1142/S0129054117500101
7. Mangu L., Kingsbury B., Soltau H., Kuo H.-K., Picheny M. Efficient spoken term detection using confusion networks. Proc. of the IEEE International Conference on Acoustics, Speech, and Signal Processing (ICASSP), 2014, pp. 7844–7848. https://doi.org/10.1109/ICASSP.2014.6855127
8. Allauzen C., Riley M., Schalkwyk J. A filter-based algorithm for efficient composition of finite-state transducers. International Journal of Foundations of Computer Science, 2011, vol. 22, no. 8, pp. 1781–1795. https://doi.org/10.1142/S0129054111009033