Menu
Publications
2025
2024
2023
2022
2021
2020
2019
2018
2017
2016
2015
2014
2013
2012
2011
2010
2009
2008
2007
2006
2005
2004
2003
2002
2001
Editor-in-Chief
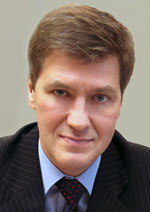
Nikiforov
Vladimir O.
D.Sc., Prof.
Partners
doi: 10.17586/2226-1494-2021-21-6-887-894
An approach to the identification of the state of elements in cyber-physical systems based on principal component analysis
Read the full article

Article in Russian
For citation:
Abstract
For citation:
Semenov V.V. An approach to the identification of the state of elements in cyber-physical systems based on principal component analysis. Scientific and Technical Journal of Information Technologies, Mechanics and Optics, 2021, vol. 21, no. 6, pp. 887–894 (in Russian). doi: 10.17586/2226-1494-2021-21-6-887-894
Abstract
The close integration of modern cyber-physical systems with production and technological ones as well as with critical information infrastructure requires improving the monitoring process. The monitoring process is necessary with a constant increase in the possible points of entry into the system. The processing of a large amount of data coming from monitoring systems requires significant computing power. In this regard, it is relevant to reduce the dimension of the feature space while maintaining an acceptable monitoring accuracy. The proposed solution should be invariant to the dimension and orders of magnitude from which the time series supplied to the input of the monitoring system are composed. To obtain the most informative features in the formation of their set, it is proposed to use principal component analysis, and a method based on decision trees for their classification. A computational experiment was performed to confirm the applicability of the developed approach. The data of the network traffic for the research stand of the cyber-physical system water treatment were used in the experiment. The accuracy of the set of methods on the analyzed data was 98.74 %. The comparison with known studies showed an increase in the F-measure up to 0.925, which is 4.8 % higher than the most effective method used to date, namely the Isolation Forest method. The developed approach allows one to significantly increase the speed of identification and to detect anomalies of information security and functional safety of cyber-physical systems with high accuracy by reducing the dimension of the original feature space. The proposed approach can be used in event monitoring systems that deal with information security. The presented theoretical results can be useful for researchers of information security and functional safety of cyber-physical systems.
Keywords: information security, functional safety, cyber-physical systems, identification of anomalies, time series analysis, principal component analysis, monitoring systems
References
References
-
Cardenas A., Amin S., Sinopoli B., Giani A., Perrig A., Sastry S. Challenges for securing cyber physical systems.Workshop on Future Directions in Cyber-Physical Systems Security, 2009.
-
ZegzhdaD., VasilevU., PoltavtsevaM., KefeleI., BorovkovA. Advanced production technologies security in the era of digital transformation. Voprosy kiberbezopasnosti, 2018, no. 2(26), pp. 2–15. (inRussian). https://doi.org/10.21681/2311-3456-2018-2-2-15
-
Semenov V., Salakhutdinova K., Lebedev I., Sukhoparov M. Identification of abnormal functioning during the operation devices of cyber-physical systems. Journal of Applied Informatics, 2019, vol. 14, no. 6(84), pp. 114–122. (in Russian). https://doi.org/10.24411/1993-8314-2019-10053
-
Zegzhda D.P. Sustainability as a criterion for information security in cyber-physical systems. Automatic Control and Computer Sciences, 2016,vol. 50, no. 8, pp. 813–819. https://doi.org/10.3103/S0146411616080253
-
Pavlenko E.Yu., Shtyrkina A.A., Zegzhda D.P. Estimating the cyber-physical system sustainability based on spectral graph theory. Information Security Problems. Computer Systems, 2019, no. 1, pp. 60–68. (in Russian)
-
Gómez A., Maimó L., Celdrán A., Clemente F. MADICS: A methodology for anomaly detection in industrial control systems. Symmetry, 2020, vol. 12, no. 10, pp. 1583. https://doi.org/10.3390/sym12101583
-
Wang X., Zhou Q., Harer J., Brown G., Qiu S., Dou Z., Wang J., Hinton A., Gonzalez C.A., Chin P. Deep learning-based classification and anomaly detection of side-channel signals. Proceedings of SPIE, 2018, vol. 10630, pp. 1063006. https://doi.org/10.1117/12.2311329
-
Semenov V.V., Lebedev I.S., Sukhoparov M.E., Salakhutdinova K.I. Application of an autonomous object behavior model to classify the cybersecurity state. Lecture Notes in Computer Science, 2019, vol. 11660, pp. 104–112. https://doi.org/10.1007/978-3-030-30859-9_9
-
Meleshko A.V., Desnitsky V.A., Kotenko I.V. Machine learning based approach to detection of anomalous data from sensors in cyber-physical water supply systems. IOP Conference Series: Materials Science and Engineering, 2020, vol. 709, pp. 033034. https://doi.org/10.1088/1757-899X/709/3/033034
-
Sukhoparov M.E., Semenov V.V., Lebedev I.S. Information security monitoring of elements of cyber-physical systems using artificial neural networks. Metody i Tekhnicheskie Sredstva Obespecheniya Bezopasnosti Informatsii, 2018, no. 27, pp. 59–60. (in Russian)
-
Osin A.V., Sheluhin O.I. Multifractal properties of the real-time traffic. Jelektrotehnicheskie i informacionnye kompleksy i sistemy, 2006, vol. 2, no. 3, pp. 36–43. (in Russian)
-
Semenov V., Sukhoparov M., Lebedev I. An approach to classification of the information security state of elements of cyber-physical systems using side electromagnetic radiation. Lecture Notes in Computer Science, 2018, vol. 11118, pp. 289–298. https://doi.org/10.1007/978-3-030-01168-0_27
-
Li D., Chen D., Jin B., Shi L., Goh J., Ng S.-K. MAD-GAN: Multivariate anomaly detection for time series data with generative adversarial networks. Lecture Notes in Computer Science, 2019, vol. 11730, pp. 703–716. https://doi.org/10.1007/978-3-030-30490-4_56
-
Medvednikova M.M. Principal component analysis for building integral indicators. Machine Learning and Data Analysis, 2012, vol. 1, no. 3, pp. 292–304. (in Russian)
-
Goh J., Adepu S., Junejo K.N., Mathur A. A dataset to support research in the design of secure water treatment systems. Lecture Notes in Computer Science,2017, vol. 10242, pp. 88–99. https://doi.org/10.1007/978-3-319-71368-7_8
-
Kravchik M., Shabtai A. Detecting cyber attacks in industrial control systems using convolutional neural networks.Proc. of the 2018 Workshop on Cyber-Physical Systems Security and PrivaCy, 2018, pp. 72–83. https://doi.org/10.1145/3264888.3264896
-
Shalyga D., Filonov P., Lavrentyev A. Anomaly detection for water treatment system based on neural network with automatic architecture optimization. arXiv, 2018, arXiv:1807.07282.
-
Inoue J., Yamagata Y., Chen Y., Poskitt C.M., Sun J. Anomaly detection for a water treatment system using unsupervised machine learning. Proc. of the 2017 IEEE International Conference on Data Mining Workshops (ICDMW), 2017, pp. 1058–1065. https://doi.org/10.1109/ICDMW.2017.149
-
Kravchik M., Shabtai A. Efficient cyber attack detection in industrial control systems using lightweight neural networks and PCA. IEEE Transactions on Dependable and Secure Computing, 2021, in press. https://doi.org/10.1109/TDSC.2021.3050101
-
Elnour M., Meskin N., Khan K., Jain R. A dual-isolation-forests-based attack detection framework for industrial control systems. IEEE Access, 2020, vol. 8, pp. 36639–36651. https://doi.org/10.1109/ACCESS.2020.2975066