Menu
Publications
2024
2023
2022
2021
2020
2019
2018
2017
2016
2015
2014
2013
2012
2011
2010
2009
2008
2007
2006
2005
2004
2003
2002
2001
Editor-in-Chief
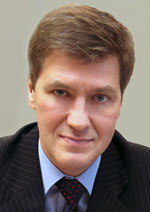
Nikiforov
Vladimir O.
D.Sc., Prof.
Partners
doi: 10.17586/2226-1494-2022-22-1-101-113
An optimal swift key generation and distribution for QKD
Read the full article

Article in English
For citation:
Abstract
For citation:
Suma M.R., Madhumathy P. An optimal swift key generation and distribution for QKD. Scientific and Technical Journal of Information Technologies, Mechanics and Optics, 2022, vol. 22, no. 1, pp. 101–113. doi: 10.17586/2226-1494-2022-22-1-101-113
Abstract
Secured transmission between users is essential for communication system models. Recently, cryptographic schemes were introduced for secured transmission and secret transmission between cloud users. In a cloud environment, there are many security issues that occur among the cloud users such as, account hacking, data breaches, broken authentication, compromised credentials, and so on. Quantum mechanics has been implemented in cryptography that made it efficient for strong security concerns over outsourced data in a cloud environment. Therefore, the present research focuses on providing excellent security for cloud users utilizing a swift key generation model for QKD cryptography. The Quantum Key Distribution (QKD) is an entirely secure scheme known as Cloud QKDP. Initially, a random bit sequence is generated to synchronize the channel. An eavesdropper will not permit to synchronize parameters between them. In this key reconciliation technique, the random bit sequence is concatenated with the photon polarisation state. BB84 protocol is improved by optimizing its bit size using FireFly Optimization (FFO) at the compatibility state, and in the next state, both transmitter and receiver generate a raw key. Once the key is generated, it is then used for the transmission of messages between cloud users. Furthermore, a Python environment is utilized to execute the proposed architecture, and the accuracy rate of the proposed model attained 98 %, and the error rate is 2 %. This proves the performance of the proposed firefly optimization algorithm based swift key generation model for QKD performs better than previous algorithms.
Keywords: cryptography, quantum mechanics, quantum key distribution (QKD), eavesdropper: BB84 protocol, reconciliation and firefly optimization
References
References
-
Varma S., Shinde M., Chavan S.S. Analysis of PCA and LDA features for facial expression recognition using SVM and HMM classifiers. Techno-Societal 2018. Proc. of the 2nd International Conference on Advanced Technologies for Societal Applications.Vol. 1, 2020, pp. 109–119. https://doi.org/10.1007/978-3-030-16848-3_11
-
Ryumina E.V. Karpov A.A Analytical review of methods for emotion recognition by human face expressions. Scientific and Technical Journal of Information Technologies, Mechanics and Optics, 2020, vol. 20, no. 2, pp. 163–176. (in Russian). https://doi.org/10.17586/2226–1494-2020-20-2-163-176
-
Akhmetshin R.I., Kirpichnikov A.P., Shleimovich M.P. Human emotion recognition in images. Vestnik Technologicheskogo Universiteta, 2015, vol. 18, no. 11, pp. 160–163. (in Russian)
-
Erman E.A., Mamdouh Mokhammed Gomaa Mokhammed. Face detection in image by using a combination of Viola - Jones method and skin detection algorithms. Vestnik of Astrakhan State Technical University. Series: Management, Computer Sciences and Informatics, 2015, no. 1, pp. 49–55. (in Russian)
-
Mukhamadieva K.B. Comparative analysis of facial recognition algorithms. Modern Materials, Equipment, and Technology, 2017, no. 7(15), pp. 58–62. (in Russian)
-
Amelkin S. A., Zakharov A.V., Khachumov V.M. Generalized Mahalanobis Euclidean distance and its properties. Information Technologies and Computer Systems, 2006, no. 4, pp. 40–44. (in Russian)
-
KaramizadehS., AbdullahS.M., ManafA.A., ZamaniM., HoomanA. An overview of principal component analysis. Journal of Signal and Information Processing, 2013, vol. 4,pp. 173–175. https://doi.org/10.4236/jsip.2013.43B031
-
Dino H.I., Abdulrazzaq M.B. Facial expression classification based on SVM, KNN and MLP classifiers. Proc. of the International Conference on Advanced Science and Engineering (ICOASE 2019), 2019, pp. 70–75. https://doi.org/10.1109/ICOASE.2019.8723728
-
Greche L., Es-Sbai N., Lavendelis E. Histogram of oriented gradient and multi layer feed forward neural network for facial expression identification. Proc. of the International Conference on Control, Automation and Diagnosis (ICCAD 2017), 2017, pp. 333–337. https://doi.org/10.1109/CADIAG.2017.8075680
-
Greche L., Akil M., Kachouri R., Es-Sbai N. A new pipeline for the recognition of universal expressions of multiple faces in a video sequence. Journal of Real-Time Image Processing, 2020, vol. 17, no. 5, pp. 1389–1402. https://doi.org/10.1007/s11554-019-00896-5
-
Kulke L., Brümmer L., Pooresmaeili A., Schacht A. Overt and covert attention shifts to emotional faces: Combining EEG, eye tracking, and a go/no-go paradigm. Psychophysiology, 2021, vol. 58, no. 8, pp. e13838. https://doi.org/10.1111/psyp.13838
-
Aleksic P.S., Katsaggelos A.K. Automatic facial expression recognition using facial animation parameters and multistream HMMs. IEEE Transactions on Information Forensics and Security, 2006, vol. 1, no. 1, pp. 3–11. https://doi.org/10.1109/TIFS.2005.863510
-
Gardner M.W., Dorling S.R. Artificial neural networks (the multilayer perceptron) – a review of applications in the atmospheric sciences. Atmospheric Environment, 1998, vol. 32, no. 14-15, pp. 2627–2636. https://doi.org/10.1016/S1352-2310(97)00447-0
-
Cao H., Cooper D.G., Keutmann M.K., Gur R.C., Nenkova A., Verma R. CREMA-D: Crowd-sourced emotional multimodal actors dataset. IEEE Transactions on Affective Computing, 2014, vol. 5, no. 4, pp. 377–390. https://doi.org/10.1109/TAFFC.2014.2336244
-
Challenges in Representation Learning Facial Expression Recognition Challenge. Available at: https://www.kaggle.com/c/challenges-in-representation-learning-facial-expression-recognition-challenge (accessed: 13.11.2021).