Menu
Publications
2024
2023
2022
2021
2020
2019
2018
2017
2016
2015
2014
2013
2012
2011
2010
2009
2008
2007
2006
2005
2004
2003
2002
2001
Editor-in-Chief
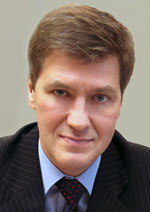
Nikiforov
Vladimir O.
D.Sc., Prof.
Partners
doi: 10.17586/2226-1494-2022-22-1-155-166
Imputation and system modeling of acid-base state parameters for different groups of patients
Read the full article

Article in English
For citation:
Abstract
For citation:
Kurapeev D.I., Lushnov M.S., Man T., Zhukova N.A. Imputation and system modeling of acid-base state parameters for different groups of patients. Scientific and Technical Journal of Information Technologies, Mechanics and Optics, 2022, vol. 22, no. 1, pp. 155–166. doi: 10.17586/2226-1494-2022-22-1-155-166
Abstract
The paper investigated the possibility of correct replacement of missing values in sets of acid-base state in the artery and vein in different groups of patients with different outcomes of the disease: “discharged”, “died”, “transferred to another medical institution”, as well as prospects for the application of individual optimization multidimensional estimates of these biomedical parameters in the form of projections on a one-dimensional space. The relevance of the above tasks is determined by the need for the full use of medical data in the analysis of large repositories of information of medical organizations and the provision of verified multidimensional assessments of biomedical systems to doctors from a large range of patient health indicators. A statistical method has been applied to verify the correctness of imputation data sets using discriminant analysis procedures. Further, the imputed data set was processed to obtain a symmetric correlation matrix optimized in a certain way and the accompanying logarithms of criterion functions that are individual system assessments of the condition of each patient in different groups of patients at a certain point in the study. After that, to identify differences in the logarithms of the criterion functions of the acid-base state parameters between groups of patients with different outcomes, the authors used the method of calculating multidimensional Hotelling T2 statistics. The correctness of the application of discriminant analysis procedures to verify the imputation of data sets is shown. Differences in the logarithms of the criteria functions of the acid-base state indicators between venous and arterial blood by patient outcome groups were revealed. Significant differences in the parameters of acid-base state based on the multidimensional statistics of T2 Hotelling between groups of patients with different outcomes were revealed. It is found that data imputation significantly increases the volume and representativeness of the sample under study. It is demonstrated that the substituted data make it possible to carry out a systematic statistical assessment of the totality of body parameters based on the calculation of the logarithms of the criterion functions of the acid-base state. Such logarithms make it possible to reliably distinguish patients in different groups of patients by outcomes in three groups: “discharged”, “deceased”, “transferred to another medical institution”. 100 % differences of biochemical parameters according to the multivariate T2 Hotelling statistics between these three groups of patients with COVID-19 are shown. The results of the study can be applied in the development of information systems of individual medical biochemical and hematological devices and analyzers and corresponding artificial intelligence systems in the future.
Keywords: acid-base state, imputation, medical information system, COVID-19, criterion function
Acknowledgements. The research was supported by the Russian Science Foundation under Grant No. 17-15-01177. The research was carried out within the framework of the budget theme No. 0060-2019-0011.
References
Acknowledgements. The research was supported by the Russian Science Foundation under Grant No. 17-15-01177. The research was carried out within the framework of the budget theme No. 0060-2019-0011.
References
-
Anokhin P.K. Theory of a functional system. Uspekhi Fiziologicheskikh Nauk, 1970, vol. 1, no. 1, pp. 19–54. (in Russian)
-
Haken G. Information and Self-Organization: A Macroscopic Approach to Complex Systems. Springer-Verlag, 1988, 188 p.
-
Lushnov A.M., Lushnov M.S. Medical Information Systems: Multidimensional Analysis of Medical and Environmental Data. Saint Petersburg, Helikon Plus, 2013, 458 p. (in Russian)
-
Kurapeev D.I., Lushnov M.S., Osipov V.Yu., Vodyaho A.I., Zhukova N.A. Synthesis of integral models of system dynamics of an Acid-Base State (ABS) of patients at operative measures. Acta Scientific Medical Sciences, 2019, vol. 3, no. 3, pp. 16–29.
-
Kurapeev D.I., Lushnov M.S., Zhukova N.A. Comparison of integral models of systemic dynamics of Acid-Base State of venous and arterial circulating blood in patients with surgical interventions. Acta Scientific Medical Sciences, 2019, vol. 3, no. 6, pp. 38–48.
-
Lebedev S., Zhukova N., Vodyaho A., Kurapeev D., Lushnov M. An ontology-driven toolset for fast prototyping of medical data processing systems. International Journal of Biology and Biomedical Engineering, 2017, vol. 11, pp. 135–142.
-
Narenda P.M., Fukunaga K. A branch and bound algorithm for feature subset selection. IEEE Transactions Computers, 1977, vol. C-26, no. 9, pp. 917–922. https://doi.org/10.1109/TC.1977.1674939
-
Ridout M.S. An improved branch and bound algorithm for feature subset-selection. Journal of the Royal Statistical Society. Series C (Applied Statistics), 1988, vol. 37, no. 1, pp. 139–147. https://doi.org/10.2307/2347512
-
Bech C.N., Brabrand M., Mikkelsen S., Lassen A. Risk factors associated with short term mortality changes over time, after arrival to the emergency department. Scandinavian Journal of Trauma, Resuscitation and Emergency Medicine, 2018, vol. 26, no. 1, pp. 29. https://doi.org/10.1186/s13049-018-0493-2
-
Fuchs P.A., Del Junco D.J., Fox E.E., Holcomb J.B., Rahbar M.H., Wade C.A., Alarcon L.H., Brasel K.J., Bulger E.M., Cohen M.J., Myers J.G., Muskat P., Phelan H.A., Schreiber M.A., Cotton B.A. Purposeful variable selection and stratification to impute missing Focused Assessment with Sonography for Trauma data in trauma research. Journal of Trauma and Acute Care Surgery, 2013, vol. 75, no. 1 (suppl. 1), pp. S75–S81. https://doi.org/10.1097/TA.0b013e31828fa51c
-
Mantrova A.I. How can omissions in medical data affect the results of research? Scientific Review. Biological Sciences, 2019, no. 2, pp. 5–9. (in Russian)
-
Lefering R., Huber-Wagner S., Nienaber U., Maegele M., Bouillon B. Update of the trauma risk adjustment model of the TraumaRegister DGU™: the Revised Injury Severity Classification, version II. Critical Care, 2014, vol. 18, no. 5, pp. 476. https://doi.org/10.1186/s13054-014-0476-2
-
Seleno N., Vogel J., Liao M., Hopkins E., Byyny R., Moore E., Gravitz C., Haukoos J. Denver trauma organ failure score outperforms traditional methods of risk stratification in trauma. Academic Emergency Medicine, 2012, vol. 19, suppl. 1, pp. S144.
-
Zolin P.P. Statistical processing of censored samples in the study of extreme and terminal states. Pathogenesis, clinical findings and therapy of extreme and terminal states: Proceedings of scientific and practical conference. Omsk, 1998, pp. 39–43. (in Russian)
-
Fabian-Jessing B.K., Vallentin M.F., Secher N., Hansen F.B., Dezfulian C., Granfeldt A., Andersen L.W. Animal models of cardiac arrest: A systematic review of bias and reporting. Resuscitation, 2018, vol. 125, pp. 16–21. https://doi.org/10.1016/j.resuscitation.2018.01.047
-
Krantz M.J., Kaul S. The ATLAS ACS 2–TIMI 51 trial and the burden of missing data: (Anti-Xa Therapy to Lower Cardiovascular Events in Addition to Standard Therapy in Subjects With Acute Coronary Syndrome ACS 2–Thrombolysis In Myocardial Infarction 51). Journal of the American College of Cardiology, 2013, vol. 62, no. 9, pp. 777–781. https://doi.org/10.1016/j.jacc.2013.05.024
-
Little R.J., D’Agostino R., Cohen M.L., Dickersin K., Emerson S.S., Farrar J.T., Frangakis C., Hogan J.W., Molenberghs G., Murphy S.A., Neaton J.D., Rotnitzky A., Scharfstein D., Shih W.J., Siegel J.P., Stern H. The prevention and treatment of missing data in clinical trials. New England Journal of Medicine, 2012, vol. 367, pp. 1355–1360. https://doi.org/10.1056/NEJMsr1203730
-
National Research Council. The prevention and treatment of missing data in clinical trials. Washington, DC, National Academies Press, 2010, 162 p.
-
Fabrykant M.S. Model-oriented approach to missing values: Multiple imputation in multilevel regression using R (on the example of analyzing survey data). Sociology: methodology, methods, mathematical modeling (Sociology:4M), 2015, no. 41, pp. 7–29. (in Russian)
-
Van Buuren S. Flexible Imputation of Missing Data. CRC Press, 2012, 342 p. https://doi.org/10.1201/b11826
-
Kulikova K.Yu. Statistical analysis of medical data with missing values. Management processes and sustainability, 2014, vol. 1, no. 1, pp. 253–258. (in Russian)
-
Witkiewitz K., Falk D.E., Kranzler H.R., Litten R.Z., Hallgren K.A., O’Malley S.S., Anton R.F. Methods to analyze treatment effects in the presence of missing data for a continuous heavy drinking outcome measure when participants drop out from treatment in alcohol clinical trials. Alcoholism: Clinical and Experimental Research, 2014, vol. 38, no. 11, pp. 2826–2834. https://doi.org/10.1111/acer.12543
-
Parvez B., Shah A., Muhammad R., Shoemaker M.B., Graves A.J., Heckbert S.R., Xu H., Ellinor P.T., Benjamin E.J., Alonso A., Shintani A.K., Roden D., Darbar D. Replication of a risk prediction model for ambulatory incident atrial fibrillation using electronic medical record. Circulation, 2012, vol. 126, no. 21, meeting abstract 18578.
-
Zhang Z. Multiple imputation with multivariate imputation by chained equation (MICE) package. Annals of Translational Medicine, 2016, vol. 4, no. 2, pp. 30. https://doi.org/10.3978/j.issn.2305-5839.2015.12.63
-
Aladyshkina A.S., Lakshina V.V., Leonova L.A., Maksimov A.G. Working with data on population health: imputation. Social aspects of population health, 2020, vol. 66, no. 1, pp. 12. (in Russian). https://doi.org/10.21045/2071-5021-2020-66-1-12
-
Little R.J.A., Rubin D.B. Statistical Analysis with Missing Data. John Wiley & Sons, 2014, 408 p.
-
Seitz C., Lanius V., Lippert S., Gerlinger C., Haberland C., Oehmke F., Tinneberg H.-R. Patterns of missing data in the use of the endometriosis symptom diary. BMC Women's Health, 2018, vol. 18, no. 1, pp. 88. https://doi.org/10.1186/s12905-018-0578-0