Menu
Publications
2025
2024
2023
2022
2021
2020
2019
2018
2017
2016
2015
2014
2013
2012
2011
2010
2009
2008
2007
2006
2005
2004
2003
2002
2001
Editor-in-Chief
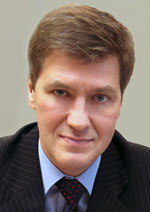
Nikiforov
Vladimir O.
D.Sc., Prof.
Partners
doi: 10.17586/2226-1494-2022-22-2-287-293
Classification of short texts using a wave model
Read the full article

Article in Russian
For citation:
Abstract
For citation:
Gruzdeva A.S., Bessmertny I.A. Classification of short texts using a wave model. Scientific and Technical Journal of Information Technologies, Mechanics and Optics, 2022, vol. 22, no. 2, pp. 287–293 (in Russian). doi: 10.17586/2226-1494-2022-22-2-287-293
Abstract
Quantum computing algorithms are actively developed and applied in the field of natural language processing. The authors of the paper proposed a new quantum-like method for classifying short texts. The basis of the method is the representation of the text as an ensemble of elementary particles. The value of the detection probability amplitude of a given ensemble at the selected points in space is chosen as a classification criterion. In this case, the space is understood as a vector space described using the distributive-semantic model of the language. The authors suggested one of the possible ways of interpreting the parameters of the wave function that describes the behavior of an elementary particle, as well as an algorithm for calculating the probability amplitude taking into account these parameters. For the experimental research of the described method, authors performed the classification of Internet communities by topics. For the analysis, the names and the “information” section of communities were used. In total, 100 groups of the social network “VKontakte” belonging to five various topics were taken. The proposed model showed rather high classification accuracy (91 % in general on the data set and from 75 % to 95 % within individual classes). The proposed model is supposed to be used to classify user comments about goods, services and events, as well as to determine some properties of the psychological portraits of users of online communities.
Keywords: classification, natural language processing, wave model, interference, quantum-like model, definition of the text subject
Acknowledgements. The work was carried out within the framework of the project No. 620164 (artificial intelligence methods for cyber-physical systems).
References
Acknowledgements. The work was carried out within the framework of the project No. 620164 (artificial intelligence methods for cyber-physical systems).
References
-
Nielsen M.A., Chuang I.L. Quantum Computation and Quantum Information. Cambridge University Press, 2010, 704 р. https://doi.org/10.1017/CBO9780511976667
-
Melucci M. Introduction to Information Retrieval and Quantum Mechanics. Berlin, Heidelberg, Springer-Verlag, 2015, 247 р. https://doi.org/10.1007/978-3-662-48313-8
-
Blacoe W., Kashefi E., Lapata M. A Quantum-theoretic approach to distributional semantics. Proc. of the 2013 Conference of the North American Chapter of the Association for Computational Linguistics: Human Language Technologies (NAACL HLT), 2013, pp. 847–857.
-
Jaiswal A.K., Holdack G., Frommholz I., Liu H. Quantum-like Generalization of complex word embedding: a lightweight approach for textual classification. CEUR Workshop Proceedings, 2018, vol. 2191, pp. 159–168.
-
Surov I.A., Semenenko E., Platonov A.V., Bessmertny I.A., Galofaro F., Toffano Z., Khrennikov A.Y., Alodjants A.P. Quantum semantics of text perception. Scientific Reports, 2021, vol. 11, no. 1, pp. 4193. https://doi.org/10.1038/s41598-021-83490-9
-
Pang B., Lee L. A sentimental education: Sentiment analysis using subjectivity summarization based on minimum cuts. Proc. of the 42nd Annual Meeting Association for Computational Linguistics (ACL),2004, pp. 271–278.https://doi.org/10.3115/1218955.1218990
-
KotelnikovE., KlekovkinaM. The automatics entimenttext classification method based on emotional vocabulary. Digital Libraries: Advanced Methods and Technologies. Proc. of the RCDL-2012, 2012, pp. 118–123.(in Russian)
-
Menshikov I.L. Sentiment analysis of a text in russian using graph models. Proc. of the Conference AIST'2013 “Analysis of Images, Social Networks, and Texts”. Ekaterinburg, 2013, pp. 151–155.(in Russian)
-
TatarnikovaT.M., BogdanovP.Yu. Human psychecreationby application o fnaturall anguageprocessing technologies. Scientific and Technical Journal of Information Technologies, Mechanics and Optics, 2021, vol. 21, no. 1, pp. 85–91. (in Russian). https://doi.org/10.17586/2226-1494-2021-21-1-85-91
-
LitvinovaT.A., ZagorovskaiaO.V., SeredinP.V., LantiukhovaN.N., ShevchenkoI.C. Author profiling of a written text: approaches, methods, and their optimization.Philology, Art Criticism, and Cultural Studies: Topical Issues and Development Trends. Рroceedings of the International Research-to-Practice Сonference. May, 13, 2013.Novosibirsk, SibAK, 2013, pp. 69–79. (inRussian)
-
Frenkel J. Wave Mechanics: Elementary Theory. The Quantum Physics. Moscow, URSS, 2019, 392 p. (in Russian)
-
KutuzovA., KuzmenkoE. WebVectors: Atoolkit for building web interfaces for vector semantic models. Communications in Computer and Information Science,2017,vol. 661, pp. 155–161. https://doi.org/10.1007/978-3-319-52920-2_15
-
Panchenko A., Ustalov D., Arefyev N., Paperno D., Konstantinova N., Loukachevitch N.,Biemann C. Human and machine judgements about russian semantic relatedness. Communications in Computer and Information Science, 2017, vol. 661, pp. 221–235. https://doi.org/10.1007/978-3-319-52920-2_21
-
Kolb P. Experiments on the difference between semantic similarity and relatedness. Proc. of the 17th Nordic Conference of Computational Linguistics(NODALIDA '09),2009,pp. 81–88.
-
Kolb P. DISCO: A multilingual database of distributionally similar words. Proc. of the KONVENS-2008, Berlin, 2008, pp. 6–12.