Menu
Publications
2025
2024
2023
2022
2021
2020
2019
2018
2017
2016
2015
2014
2013
2012
2011
2010
2009
2008
2007
2006
2005
2004
2003
2002
2001
Editor-in-Chief
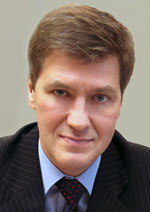
Nikiforov
Vladimir O.
D.Sc., Prof.
Partners
doi: 10.17586/2226-1494-2022-22-2-308-316
Constructing twitter corpus of Iraqi Arabic Dialect (CIAD) for sentiment analysis
Read the full article

Article in English
For citation:
Abstract
For citation:
Hassoun Al-Jawad M.M., Alharbi H., Almukhtar A.F., Alnawas A.A. Constructing twitter corpus of Iraqi Arabic Dialect (CIAD) for sentiment analysis. Scientific and Technical Journal of Information Technologies, Mechanics and Optics, vol. 22, no. 2, pp. 308–316. doi: 10.17586/2226-1494-2022-22-2-308-316
Abstract
The number of Twitter users in Iraq has increased significantly in recent years. Major events, the political situation in the country, had a significant impact on the content of Twitter and affected the tweets of Iraqi users. Creating an Iraqi Arabic Dialect corpus is crucial for sentiment analysis to study such behaviors. Since no such corpus existed, this paper introduces the Corpus of Iraqi Arabic Dialect (CIAD). The corpus has been collected, annotated and made publicly accessible to other researchers for further investigation. Furthermore, the created corpus has been validated using eight different combinations of four feature-selections approaches and two versions of Support Vector Machine (SVM) algorithm. Various performance measures were calculated. The obtained accuracy, 78 %, indicates a promising potential application.
Keywords: sentiment analysis, data mining, support vector machine, user behaviors, social media mining
References
References
-
Stone M.L. et al. BIg data for Media. Reuters Institute for the Study of Journalism, 2014, november.
-
Badaro G., Baly R., Hajj H., El-Hajj W., Shaban K.B., Habash N., Al-Sallab A., Hamdi A. A survey of opinion mining in Arabic: A comprehensive system perspective covering challenges and advances in tools, resources, models, applications, and visualizations. ACM Transactions on Asian and Low-Resource Language Information Processing, 2019, vol. 18, no. 3, pp. 27.
-
Zaidan O.F., Callison-Burch C. Arabic dialect identification. Computational Linguistics, 2014, vol. 40, no. 1, pp. 171–202. https://doi.org/10.1162/COLI_a_00169
-
Habash N.Y. Introduction to Arabic natural language processing. Synthesis Lectures on Human Language Technologies, 2010, vol. 3, no. 1. https://doi.org/10.2200/S00277ED1V01Y201008HLT010
-
Alnawas A., Arici N. The corpus based approach to sentiment analysis in modern standard Arabic and Arabic dialects: A literature review. Journal of Polytechnic - Politeknik Dergisi, 2018, vol. 21, no. 2, pp. 461–470. https://doi.org/10.2339/politeknik.403975
-
Alshutayri A., Atwell E. Classifying Arabic dialect text in the Social Media Arabic Dialect Corpus (SMADC). Proc. of the 3rd Workshop on Arabic Corpus Linguistics, 2019, pp. 51–59.
-
Abo M.E.M., Raj R.G., Qazi A. A review on Arabic sentiment analysis: State-of-The-Art, taxonomy and open research challenges. IEEE Access, 2019, vol. 7, pp. 162008–162024. https://doi.org/10.1109/ACCESS.2019.2951530
-
Kumar A., Jaiswal A. Systematic literature review of sentiment analysis on Twitter using soft computing techniques. Concurrency and Computation: Practice and Experience, 2020, vol. 32, no. 1, pp. e5107. https://doi.org/10.1002/cpe.5107
-
Cieliebak M., Deriu J., Egger D., Uzdilli F. A Twitter corpus and benchmark resources for German sentiment analysis. Proc. of the 5th International Workshop on Natural Language Processing for Social Media, SocialNLP, 2017, pp. 45–51. https://doi.org/10.18653/v1/W17-1106
-
Nabil M., Aly M., Atiya A.F. ASTD: Arabic sentiment tweets dataset. Proc. of the Conference on Empirical Methods in Natural Language Processing (EMNLP), 2015, pp. 2515–2519. https://doi.org/10.18653/v1/D15-1299
-
Alahmary R.M., Al-Dossari H.Z., Emam A.Z. Sentiment analysis of Saudi dialect using deep learning techniques. Proc. of the 18th International Conference on Electronics, Information, and Communication (ICEIC), 2019, pp. 8706408. https://doi.org/10.23919/ELINFOCOM.2019.8706408
-
Alnawas A., Arici N. Sentiment analysis of Iraqi Arabic dialect on Facebook based on distributed representations of documents. ACM Transactions on Asian and Low-Resource Language Information Processing, 2019, vol. 18, no. 3, pp. a20. https://doi.org/10.1145/3278605
-
Kwaik K.A., Saad M., Chatzikyriakidis S., Dobnik S. Shami: A corpus of levantine Arabic dialects. Proc. of the 11th International Conference on Language Resources and Evaluation. (LREC-2018), 2019, pp. 3645–3652.
-
Oussous A., Lahcen A.A., Belfkih S. Impact of text pre-processing and ensemble learning on Arabic sentiment analysis. ACM International Conference Proceeding Series, 2019, vol. part F148154, pp. 65. https://doi.org/10.1145/3320326.3320399
-
El Abdouli A., Hassouni L., Anoun H. Sentiment analysis of moroccan tweets using naive bayes algorithm. International Journal of Computer Science and Information Security, 2017, vol. 15, no. 12, pp. 191–200.
-
Bouazizi M., Ohtsuki T. Sentiment analysis: From binary to multi-class classification: A pattern-based approach for multi-class sentiment analysis in Twitter. Proc. of the IEEE International Conference on Communications (ICC), 2016, pp. 7511392. https://doi.org/10.1109/ICC.2016.7511392
-
Altamimi M., Alruwaili O., Teahan W.J. BTAC: A twitter corpus for Arabic dialect identification. Proc. of the 6th Conference on Computer-Mediated Communication (CMC) and Social Media Corpora (CMC-corpora 2018), 2018, pp. 5.
-
Al-Yasiri E.K., Al-Azawei A. Improving Arabic sentiment analysis on social media: A comparative study on applying different pre-processing techniques. Compusoft, 2019, vol. 8, no. 6, pp. 3150–3157.
-
Platt J.C. Sequential Minimal Optimization: A fast algorithm for training support vector machines. CiteSeerX, 1998, vol. 10, no. 1.43, pp. 4376.