Menu
Publications
2025
2024
2023
2022
2021
2020
2019
2018
2017
2016
2015
2014
2013
2012
2011
2010
2009
2008
2007
2006
2005
2004
2003
2002
2001
Editor-in-Chief
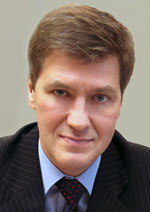
Nikiforov
Vladimir O.
D.Sc., Prof.
Partners
doi: 10.17586/2226-1494-2022-22-2-317-323
A novel framework for the prevention of black-hole in wireless sensors using hybrid convolution network
Read the full article

Article in English
For citation:
Abstract
For citation:
Kolangiappan J., Senthil Kumar A. A novel framework for the prevention of black-hole in wireless sensors using hybrid convolution network. Scientific and Technical Journal of Information Technologies, Mechanics and Optics, 2022, vol. 22, no. 2, pp. 317–323. doi: 10.17586/2226-1494-2022-22-2-317-323
Abstract
Problems of Wireless Sensor Networks (WSN) are associated with a significant increase in the number of devices on these networks. In this regard, the requirements for the protection and the security of WSN from external influences are increasing significantly. WSN security problems are solved by solving the problem of optimal path routing, energy conservation, and so on. This paper proposes a hybrid model of an efficient packet routing and delivery system to prevent Black-hole attacks. This type of attack is considered the most common on the network due to its unique characteristics. To detect such attacks, a deep learning model using a Convolutional Neural Network (CNN) is proposed. The learning algorithm must be reliable and trustworthy so that attack analysis can be considered at different levels to study the intelligent behavior of network attacks. The paper considers the problem of finding the optimal shortest path using Deep Q-Learning and convolutional neural networks to perform efficient routing and delivery of packets in a safer way. As a result of simulation, the achieved accuracy reached 98.57 %.
Keywords: convolutional neural network, CNN, Deep Q-Learning, wireless sensor network, WSN, routing, trustworthy
References
References
-
Ding Z., Zhu C., De Graef M. Determining crystallographic orientation via hybrid convolutional neural network. Materials Characterization, 2021, vol. 178, pp. 111213. https://doi.org/10.1016/j.matchar.2021.111213
-
Subasini C.A., Karuppiah S.P., Sheeba Adlin, Padmakala S. Developing an attack detection framework for wireless sensor network-based healthcare applications using hybrid convolutional neural network. Transactions on Emerging Telecommunications Technologies, 2021, vol. 32, no. 11, pp. e4336. https://doi.org/10.1002/ett.4336
-
da Silva Cotrim W., Felix L.B., Minim V.P., Campos R.C., Minim L.A. Development of a hybrid system based on convolutional neural networks and support vector machines for recognition and tracking color changes in food during thermal processing. Chemical Engineering Science, 2021, vol. 240, pp. 116679. https://doi.org/10.1016/j.ces.2021.116679
-
Bangotra D.K., Singh Y., Selwal A., Kumar N., Singh P.K., Hong W-C. An intelligent opportunistic routing algorithm for wireless sensor networks and its application towards e-healthcare. Sensors, 2020, vol. 20, no. 14, pp. 3887. https://doi.org/10.3390/s20143887
-
Pankaj R. Chandre, Dr. Parikshit N. Mahalle, Dr. Gitanjali R. Shinde. Intrusion prevention framework for WSN using Deep CNN. Turkish Journal of Computer and Mathematics Education, 2021, vol. 12, no. 6, pp. 3567–3572.
-
Pasyar P., Mahmoudi T., Kouzehkanan S.Z., Ahmadian A., Arabalibeik H., Soltanian N., Radmard A.R. Hybrid classification of diffuse liver diseases in ultrasound images using deep convolutional neural networks. Informatics in Medicine Unlocked, 2021, vol. 22, pp. 100496. https://doi.org/10.1016/j.imu.2020.100496
-
Gulla K.K., Viswanath P., Veluru S.B., Kumar R.R. Machine learning based intrusion detection techniques. Handbook of Computer Networks and Cyber Security: Principles and Paradigms, 2019, pp. 873–888. https://doi.org/10.1007/978-3-030-22277-2_35
-
Jo W., Kim S., Lee C., Shon T. Packet preprocessing in CNN-based network intrusion detection system. Electronics, 2020, vol. 9, no. 7, pp. 1151. https://doi.org/10.3390/electronics9071151
-
Yi P., Zhu T., Zhang Q., Wu Y., Li J. A denial of service attack in advanced metering infrastructure network. Proc. 1st IEEE International Conference on Communications (ICC), 2014, pp. 1029–1034. https://doi.org/10.1109/ICC.2014.6883456
-
Wang G., Ren Y., Dou K., Li J. IDTCP: an effective approach to mitigating the TCP incast problem in data center networks. Information Systems Frontiers, 2014, vol. 16, no. 1, pp. 35–44. https://doi.org/10.1007/s10796-013-9463-4
-
Wang G., Ren Y., Li J. An effective approach to alleviating the challenges of transmission control protocol. IET Communications, 2014, vol. 8, no. 6, pp. 860–869. https://doi.org/10.1049/iet-com.2012.0154
-
Al-Mandhari W., Gyoda K., Nakajima N. Performance evaluation of active route time-out parameter in ad-hoc on demand distance vector (AODV). Proc. of the 6th WSEAS International Conference on Applied Electromagnetics, Wireless and Optical, 2008, pp. 47–51.
-
McHugh J. Testing intrusion detection systems: a critique of the 1998 and 1999 darpa intrusion detection system evaluations as performed by lincoln laboratory. ACM Transactions on Information and System Security, 2000, vol. 3, no. 4, pp. 262–294. https://doi.org/10.1145/382912.382923
-
Draper-Gil G., Lashkari A.H., Mamun M.S.I., Ghorbani A.A. Characterization of encrypted and VPN traffic using time-related features. Proc. of the 2nd International Conference on Information Systems Security and Privacy (ICISSP), 2016, pp. 407–414. https://doi.org/10.5220/0005740704070414
-
Hinton G.E., Srivastava N., Krizhevsky A., Sutskever I., Salakhutdinov R.R. Improving neural networks by preventing co-adaptation of feature detectors. arXiv, 2012, arXiv:1207.0580.
-
Wang S., Minku L.L., Yao X. A systematic study of online class imbalance learning with concept drift. IEEE Transactions on Neural Networks and Learning Systems, 2018, vol. 29, no. 10, pp. 4802–4821. https://doi.org/10.1109/TNNLS.2017.2771290