Menu
Publications
2025
2024
2023
2022
2021
2020
2019
2018
2017
2016
2015
2014
2013
2012
2011
2010
2009
2008
2007
2006
2005
2004
2003
2002
2001
Editor-in-Chief
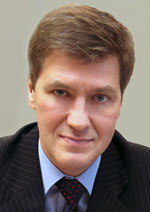
Nikiforov
Vladimir O.
D.Sc., Prof.
Partners
doi: 10.17586/2226-1494-2022-22-3-459-471
Detection of potholes on road surfaces using photogrammetry and remote sensing methods
Read the full article

Article in English
For citation:
Abstract
For citation:
Abd Mukti S.N., Tahar K.N. Detection of potholes on road surfaces using photogrammetry and remote sensing methods (review). Scientific and Technical Journal of Information Technologies, Mechanics and Optics, 2022, vol. 22, no. 3, pp. 459–471. doi: 10.17586/2226-1494-2022-22-3-459-471
Abstract
An overview of methods for obtaining 2D and 3D models of defects on the pavement is given. The integrity of the pavement can be affected by factors such as temperature, humidity, weathering and loads. Potholes are one of the most common types of pavement failure. These defects are the signs of structural failures in an asphalt road. The process of collecting and analyzing data is critical to pavement maintenance. Finding and quantifying pothole geometry information is essential to understand road maintenance forecasts and to determine the right asphalt maintenance strategies. Visual detection of road defects is costly and time consuming. Today, there are quite a lot of studies in the scientific literature showing methods for automatic detection and recognition of potholes. In our work, we consider methods for automatic detection and classification of potholes using tools — sensors integrated with a positioning system. The technique of processing two-dimensional (2D) images using various methods of machine classification allows you to determine the precise geometry of the pothole. Algorithmic methods such as artificial neural networks, decision trees, support vector machines, and fuzzy classification are used to improve the accuracy of image processing and highlight the edges of potholes. A three-dimensional model of the pothole (3D) can be obtained based on laser scanning data and photogrammetry methods. The paper summarizes various methods and proposed techniques for extracting a 3D pothole model. The results of the work can be used to improve the infrastructure for maintaining road surfaces.
Keywords: classification, image, processing, model, pavement defect, pothole
Acknowledgements. The authors thank the Faculty of Architecture, Planning and Surveying of the Universiti Teknologi MARA (UiTM), the Research Management Center (RMC) and the Ministry of Higher Education (MOHE) for providing the GPK 600-RMC/GPK 5/3 (223/2020) Foundation grant, as well as FRGS for grant FRGS/1/2021/WAB07/UITM/02/2.
References
Acknowledgements. The authors thank the Faculty of Architecture, Planning and Surveying of the Universiti Teknologi MARA (UiTM), the Research Management Center (RMC) and the Ministry of Higher Education (MOHE) for providing the GPK 600-RMC/GPK 5/3 (223/2020) Foundation grant, as well as FRGS for grant FRGS/1/2021/WAB07/UITM/02/2.
References
- Buza E., Omanovic S., Huseinovic A. Pothole detection with image processing and spectral clustering. Proc. of the 2nd International Conference on Information Technology and Computer Networks (ITCN '13), 2013.
- Cao W., Liu Q., He Z. Review of pavement defect detection methods. IEEE Access, 2020, vol. 8, pp. 14531–14544. https://doi.org/10.1109/aCCESS.2020.2966881
- KimT., RyuS.-K. Reviewandanalysisofpotholedetectionmethods. Journal of Emerging Trendsin Computing and Information Sciences,2014, vol. 5, no. 8, pp. 603–608.
- Sarie F., Bisri M., Wicaksono A., Effendi R. Types of road pavement damage for road on peatland, a study case in Palangka Raya, Central Kalimantan, Indonesia. Journal of Environmental Science, Toxicology and Food Technology, 2015, vol. 9, no. 12, pp. 53–59. https://doi.org/10.9790/2402-091235359
- WangH.-W., ChenC.-H.,ChengD.-Y.,LinC.-H.,LoC.-C. A real-time pothole detection approach for intelligent transportation system.Mathematical Problems in Engineering,2015,vol. 2015,pp. 869627. https://doi.org/10.1155/2015/869627
- Mednis A., Strazdins G., Zviedris R., Kanonirs G.,Selavo L.Real time pothole detection using Android smartphones with accelerometers.Proc. of the 7thInternational Conference on Distributed Computing in Sensor Systems and Workshops (DCOSS),2011,pp. 86376.https://doi.org/10.1109/DCOSS.2011.5982206
- Gunawan F.E. Detecting road damages by using gyroscope sensor. ICIC Express Letters, 2018, vol. 12, no. 11, pp. 1089–1098. https://doi.org/10.24507/icicel.12.11.1089
- Jamakhandi H.A., Srinivasa K.G. Internet of Things based real time mapping of road irregularities. Proc. of International Conference on Circuits, Communication, Control and Computing(I4C),2014, pp. 448–451 https://doi.org/10.1109/CIMCA.2014.7057842
- Lei T., Mohamed A.A., Claudel C. An IMU-based traffic and road condition monitoring system. HardwareX, 2018, vol. 4, pp. e00045. https://doi.org/10.1016/j.ohx.2018.e00045
- Strazdins G., Mednis A., Kanonirs G., Zviedris R., Selavo L. Towards vehicular sensor networks with android smartphones for road surface monitoring. The Second International Workshop on Networks of Cooperating Objects (CONET’11), Electronic Proceedings of CPSWeek'11, 2011, pp. 1-4.
- Rau J.Y., Hsiao K.W., Jhan J.P., Wang S.H., Fang W.C., Wang J.L. Bridge crack detection using multi-rotary UAV and object-base image analysis. International Archives of the Photogrammetry, Remote Sensing and Spatial Information Sciences - ISPRS Archives, 2017, vol. 42, no. 2W6, pp. 311–318. https://doi.org/10.5194/isprs-archives-XLII-2-W6-311-2017
- Eriksson J., Girod L., Hull B., Newton R., Madden S., Balakrishnan H. The Pothole Patrol: Using a mobile sensor network for road surface monitoring. MobiSys’08 – Proc. of the 6th International Conference on Mobile Systems, Applications, and Services, 2008, pp. 29–39. https://doi.org/10.1145/1378600.1378605
- Chambon S., Moliard J.M. Automatic road pavement assessment with image processing: Review and comparison. International Journal of Geophysics,2011, vol. 2011, pp. 989354. https://doi.org/10.1155/2011/989354
- Pan Y., Zhang X., Cervone G., Yang L. Detection of asphalt pavement potholes and cracks based on the unmanned aerial vehicle multispectral imagery. IEEE Journal of Selected Topics in Applied Earth Observations and Remote Sensing, 2018, vol. 11, no. 10, pp. 3701–3712. https://doi.org/10.1109/JSTARS.2018.2865528
- Li M., Zang S., Zhang B., Li S., Wu C. A review of remote sensing image classification techniques: The role of Spatio-contextual information. European Journal of Remote Sensing, 2014, vol. 47, no. 1, pp. 389–411. https://doi.org/10.5721/EuJRS20144723
- Qingju T., Jingmin D.A., Chunsheng L.I.U., Yuanlin L.I.U., Chunping R.Study on defects edge detection in infrared thermal image based on ant colony algorithm. International Journal of Signal Processing, Image Processing and Pattern Recognition, 2016, vol. 9, no. 4,pp. 121–130.http://dx.doi.org/10.14257/ijsip.2016.9.4.11
- Luo Z.M.,Wen J. The image segmentation algorithm of region growing and Wavelet Transform Modulus Maximum. Proc. of the 5th International Conference on Instrumentation and Measurement, Computer, Communication, and Control (IMCCC),2015, pp. 1171–1174. https://doi.org/10.1109/IMCCC.2015.251
- Sultani W., Mokhtari S., Yun H. Automatic pavement object detection using superpixel segmentation combined with conditional random field. IEEE Transactions on Intelligent Transportation Systems, 2018, vol. 19, no. 7,pp. 2076–2085.https://doi.org/10.1109/TITS.2017.2728680
- Koch C., Jog G.M., Brilakis I. Automated pothole distress assessment using asphalt pavement video data. Journal of Computing in Civil Engineering, 2013, vol. 27, no. 4, pp. 370–378. https://doi.org/10.1061/(ASCE)CP.1943-5487.0000232
- Koch C., Brilakis I. Pothole detection in asphalt pavement images. Advanced Engineering Informatics, 2011, vol. 25, no. 3, pp. 507–515. https://doi.org/10.1016/j.aei.2011.01.002
- Huang W., Zhang N. A novel road crack detection and identification method using digital image processing techniques. Proc. of the 7th International Conference on Computing and Convergence Technology (ICCCT), 2012, pp. 397–400.
- Gao M., Wang X., Zhu S., Guan P. Detection and segmentation of cement concrete pavement pothole based on image processing technology. Mathematical Problems in Engineering, 2020, pp. 1360832. https://doi.org/10.1155/2020/1360832
- Paul A., Yang C., Breitkopf U., Liu Y., Wang Z., Rottensteiner F., Wallner M., Verworn A., Heipke C. Automatic classification of aerial imagery for urban hydrological applications. International Archives of the Photogrammetry, Remote Sensing and Spatial Information Sciences – ISPRS Archives, 2018, vol. 42, no. 3, pp. 1355–1362. https://doi.org/10.5194/isprs-archives-XLII-3-1355-2018
- Maas A., Alrajhi M., Alobeid A., Heipke C. Automatic classification of high resolution satellite imagery – A case study for urban areas in the Kingdom of Saudi Arabia. International Archives of the Photogrammetry, Remote Sensing and Spatial Information Sciences – ISPRS Archives, 2017, vol. 42, no. 1W1, pp. 11–16. https://doi.org/10.5194/isprs-archives-XLII-1-W1-11-2017
- Jabari S., Fathollahi F., Zhang Y. Application of sensor fusion to improve UAV image classification. International Archives of the Photogrammetry, Remote Sensing and Spatial Information Sciences – ISPRS Archives, 2017, vol. 42, no. 2W6, pp. 153–156. https://doi.org/10.5194/isprs-archives-XLII-2-W6-153-2017
- Jawak S.D., Wankhede S.F., Luis A.J. Comparison of pixel and object-based classification techniques for glacier facies extraction. International Archives of the Photogrammetry, Remote Sensing and Spatial Information Sciences – ISPRS Archives, 2018, vol. 42, no. 5, pp. 543–548. https://doi.org/10.5194/isprs-archives-xlii-5-543-2018
- Verma A.K., Garg P.K., Prasad K.S.H., Dadhwal V.K. Classification of LISS IV imagery using decision tree methods. International Archives of the Photogrammetry, Remote Sensing and Spatial Information Sciences – ISPRS Archives, 2016, vol. 41, pp. 1061–1066. https://doi.org/10.5194/isprsarchives-XLI-B8-1061-2016
- Saini R., Ghosh S.K. Crop classification on single date sentinel-2 imagery using random forest and suppor vector machine. International Archives of the Photogrammetry, Remote Sensing and Spatial Information Sciences – ISPRS Archives, 2018, vol. 42, no. 5, pp. 683–688. https://doi.org/10.5194/isprs-archives-xlii-5-683-2018
- Shaharum N.S.N., Shafri H.Z.M., Ghani W.A.W.A., Samsatli S., Yusuf B., Al-Habshi M.M.A., Prince H.M. Image classification for mapping oil palm distribution via support vector machine using scikit-learn module. International Archives of the Photogrammetry, Remote Sensing and Spatial Information Sciences – ISPRS Archives, 2018, vol. 42, no. 4/W9, pp. 139–145. https://doi.org/10.5194/isprs-archives-XLII-4-W9-133-2018
- Kamavisdar P., Saluja S., Agrawal S. A survey on image classification approaches and techniques. International Journal of Advanced Research in Computer and Communication Engineering, 2013, vol. 2, no. 1, pp. 1005–1009.
- Abdellatif M., Peel H., Cohn A.G., Fuentes R. Hyperspectral imaging for autonomous inspection of road pavement defects. Proc. of the 36th International Symposium on Automation and Robotics in Construction (ISARC), 2019, pp. 384–392. https://doi.org/10.22260/isarc2019/0052
- Jengo C.M., Hughes D., LaVeigne J.D., Curtis I. Pothole detection and road condition assessment using hyperspectral imager. Proc. of the Annual Conference “Geospatial Goes Global: From Your Neighborhood to the Whole Planet”, 2005, pp. 461–473.
- Branco L.H.C., Segantine P.C.L. MaNIAC-UAV - A methodology for automatic pavement defects detection using images obtained by Unmanned Aerial Vehicles. Journal of Physics: Conference Series, 2015, vol. 633, no. 1, pp. 012122. https://doi.org/10.1088/1742-6596/633/1/012122
- Herold M., Roberts D., Smadi O., Noronh V. Road condition mapping with hyperspectral remote sensing.Available at: https://www.ugpti.org/smartse/research/citations/downloads/Herold-Road_Condition_Mapping_with_HSI-2004.pdf(accessed: 30.12.2021)
- Pascucci S., Bassani C., Palombo A., Poscolieri M., Cavalli R. Road asphalt pavements analyzed by airborne thermal remote sensing: Preliminary results of the venice highway. Sensors, 2008, vol. 8, no. 2, pp. 1278–1296. https://doi.org/10.3390/s8021278
- Shahi K., Shafri H.Z.M., Taherzadeh E., Mansor S., Muniandy R. A novel spectral index to automatically extract road networks from WorldView-2 satellite imagery. Egyptian Journal of Remote Sensing and Space Science, 2015, vol. 18, no. 1, pp. 27–33. https://doi.org/10.1016/j.ejrs.2014.12.003
- Pan Y., Zhang X., Sun M., Zhao Q. Object-based and supervised detection of potholes and cracks from the pavement images acquired by UAV. International Archives of the Photogrammetry, Remote Sensing and Spatial Information Sciences – ISPRS Archives, 2017, vol. 42, no. 4W4, pp. 209–217. https://doi.org/10.5194/isprs-archives-XLII-4-W4-209-2017
- Resende M.R., Bernucci L.L.B., Quintanilha J.A. Monitoring the condition of roads pavement surfaces: proposal of methodology using hyperspectral images. Journal of Transport Literature, 2014, vol. 8, no. 2, pp. 201–220. https://doi.org/10.1590/s2238-10312014000200009
- Raziq A., Xu A., Li Y., Zhao X. Extraction of urban road network from multispectral images using multivariate kernel statistic and segmentation method. Geoinformatics & Geostatistics: An Overview, 2017, vol. 5, no. 1. https://doi.org/10.4172/2327-4581.1000158
- Caltagirone L., Bellone M., Svensson L., Wahde M. LiDAR–camera fusion for road detection using fully convolutional neural networks. Robotics and Autonomous Systems, 2019, vol. 111, pp. 125–131. https://doi.org/10.1016/j.robot.2018.11.002
- Kumar P., Angelats E. An automated road roughness detection from mobile laser scanning data. International Archives of the Photogrammetry, Remote Sensing and Spatial Information Sciences – ISPRS Archives, 2017, vol. 42, no. 1W1, pp. 91–96. https://doi.org/10.5194/isprs-archives-XLII-1-W1-91-2017
- Walters R.C., Jaselskis E. Using scanning lasers for real-time pavement thickness measurement. Proc. of the 2005 ASCE International Conference on Computing in Civil Engineering, 2005, pp. 375–385. https://doi.org/10.1061/40794(179)36
- Yu S.J., Sukumar S.R., Koschan A.F., Page D.L., Abidi M.A. 3D reconstruction of road surfaces using an integrated multi-sensory approach. Optics and Lasers in Engineering, 2007, vol. 45, no. 7, pp. 808–818. https://doi.org/10.1016/j.optlaseng.2006.12.007
- Li Q., Yao M., Yao X., Yu W., Xu B. A real-time 3D scanning system for pavement rutting and pothole detections. Proceedings of SPIE, 2009, vol. 7447, pp. 74470B. https://doi.org/10.1117/12.824559
-
Mathavan S., Kamal K., Rahman M. A review of three-dimensional imaging technologies for pavement distress detection and measurements. IEEE Transactions on Intelligent Transportation Systems, 2015, vol. 16, no. 5, pp. 2353–2362. https://doi.org/10.1109/TITS.2015.2428655
-
Zhang C., Elaksher A. 3D Reconstruction from UAV-acquired Imagery for Road Surface Distress Assessment. Proc. of the 31st Asian Conference on Remote Sensing (ACRS), 2010, pp. 386–391.
-
Zhang A., Wang K.C.P., Li B., Yang E., Dai X., Peng Y., Fei Y., Liu Y., Li J.Q., Chen C. Automated pixel-level pavement crack detection on 3D asphalt surfaces using a deep-learning network. Computer-Aided Civil and Infrastructure Engineering, 2017, vol. 32, no. 10, pp. 805–819. https://doi.org/10.1111/mice.12297
-
Kertesz I., Lovas T., Barsi A. Measurement of road roughness by low-cost photogrammetric system.International Archives of the Photogrammetry, Remote Sensing and Spatial Information Sciences –ISPRS Archives,2007, vol. 36, no. 5/C55, pp. 4.
-
Hou Z., Wang K.C.P., Gong W. Experimentation of 3D pavement imaging through stereovision. Proc. of the International Conference on Transportation Engineering (ICTE), 2007, pp. 376–381. https://doi.org/10.1061/40932(246)62
-
El Gendy A., Shalaby A., Saleh M., Flintsch G.W. Stereo-vision applications to reconstruct the 3D texture of pavement surface. International Journal of Pavement Engineering, 2011, vol. 12, no. 3, pp. 263–273. https://doi.org/10.1080/10298436.2010.546858
-
Yu X., Salari E. Pavement pothole detection and severity measurement using laser imaging.Proc. of the IEEE International Conference on Electro Information Technology, 2011,pp. 5978573. https://doi.org/10.1109/EIT.2011.5978573
-
Jokela M., Kutila M., Le L. Road condition monitoring system based on a stereo camera. Proc. of the IEEE 5th International Conference on Intelligent Computer Communication and Processing (ICCP), 2009, pp. 423–428. https://doi.org/10.1109/ICCP.2009.5284724
-
Tan Y., Li Y. UAV photogrammetry-based 3D road distress detection. ISPRS International Journal of Geo-Information, 2019, vol. 8, no. 9, pp. 409. https://doi.org/10.3390/ijgi8090409
-
Ragnoli A., de Blasiis M.R., Di Benedetto A. Pavement distress detection methods: A review. Infrastructures, 2018, vol. 3, no. 4, pp. 58. https://doi.org/10.3390/infrastructures3040058
-
Wang X., Al-Shabbani Z., Sturgill R., Kirk A., Dadi G.B. Estimating earthwork volumes through use of unmanned aerial systems. Transportation Research Record, 2017, vol. 2630, pp. 1–8. https://doi.org/10.3141/2630-01
-
Tahar K.N., Ahmad A., Akib W.A.A.W.M., Mohd W.M.N.W. A generic approach for photogrammetric survey using a six-rotor unmanned aerial vehicle.IOP Conference Series: Earth and Environmental Science, 2014,vol. 18, no. 1, pp. 012003 https://doi.org/10.1088/1755-1315/18/1/012003
-
Abd Mukti S.N., Tahar K.N. Low altitude photogrammetry for urban road mapping. Built Environment Journal, 2021, vol. 18, no. 1, pp. 31. https://doi.org/10.24191/bej.v18i1.10205
-
Slimane A.B., Khoudeir M., Brochard J., Do M.T. Characterization of road microtexture by means of image analysis. Wear, 2008, vol. 264, no. 5–6, pp. 464–468. https://doi.org/10.1016/j.wear.2006.08.045
-
Jog G.M., Koch C., Golparvar-Fard M., Brilakis I. Pothole properties measurement through visual 2D recognition and 3D reconstruction. Proc. of the ASCE International Conference on Computing in Civil Engineering, 2012, pp. 553–560. https://doi.org/10.1061/9780784412343.0070
-
Saad A.M., Tahar K.N. Identification of rut and pothole by using multirotor unmanned aerial vehicle (UAV). Measurement: Journal of the International Measurement Confederation, 2019, vol. 137, pp. 647–654. https://doi.org/10.1016/j.measurement.2019.01.093
-
Biçici S., Zeybek M. An approach for the automated extraction of road surface distress from a UAV-derived point cloud. Automation in Construction, 2021, vol. 122, pp. 103475. https://doi.org/10.1016/j.autcon.2020.103475