Menu
Publications
2025
2024
2023
2022
2021
2020
2019
2018
2017
2016
2015
2014
2013
2012
2011
2010
2009
2008
2007
2006
2005
2004
2003
2002
2001
Editor-in-Chief
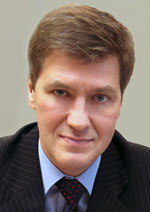
Nikiforov
Vladimir O.
D.Sc., Prof.
Partners
doi: 10.17586/2226-1494-2022-22-3-585-593
A method of multimodal machine sign language translation for natural human-computer interaction
Read the full article

Article in Russian
For citation:
Abstract
For citation:
Axyonov A.A., Kagirov I.A., Ryumin D.A. A method of multimodal machine sign language translation for natural human-computer interaction. Scientific and Technical Journal of Information Technologies, Mechanics and Optics, 2022, vol. 22, no. 3, pp. 585–593 (in Russian). doi: 10.17586/2226-1494-2022-22-3-585-593
Abstract
This paper aims to investigate the possibility of robustness enhancement as applied to an automatic system for isolated signs and sign languages recognition, through the use of the most informative spatiotemporal visual features. The authors present a method for the automatic recognition of gestural information, based on an integrated neural network model, which analyses spatiotemporal visual features: 2D and 3D distances between the palm and the face; the area of the hand and the face intersection; hand configuration; the gender and the age of signers. A 3DResNet-18-based neural network model for hand configuration data extraction was elaborated. Deepface software platform neural network models were embedded in the method in order to extract gender and age-related data. The proposed method was tested on the data from the multimodal corpus of sign language elements TheRuSLan, with the accuracy of 91.14 %. The results of this investigation not only improve the accuracy and robustness of machine sign language translation, but also enhance the naturalness of human-machine interaction in general. Besides that, the results have application in various fields of social services, medicine, education and robotics, as well as different public service centers.
Keywords: body language, gesticulation, machine sign language translation, naturalness of a communication medium
body language, gesticulation, machine sign language translation, naturalness of a communication medium
Acknowledgements. This research is financially supported by the Russian Science Foundation (No. 21-71-00141, https://rscf.ru/en/project/21-71-00141/)
References
Acknowledgements. This research is financially supported by the Russian Science Foundation (No. 21-71-00141, https://rscf.ru/en/project/21-71-00141/)
References
1. Ryumin D., Kagirov I., Ivanko D., Axyonov A., Karpov A. Automatic detection and recognition of 3D manual gestures for human-machine interaction. International Archives of the Photogrammetry, Remote Sensing and Spatial Information Sciences, 2019, vol. 42, no. 2/W12, pp. 179–183. https://doi.org/10.5194/isprs-archives-XLII-2-W12-179-2019
2. Karpov A.A., Yusupov R.M. Multimodal interfaces of human–computer interaction. Herald of the Russian Academy of Sciences, 2018, vol. 88, no. 1, pp. 67–74. https://doi.org/10.1134/S1019331618010094
3. Ryumin D., Karpov A.A. Towards automatic recognition of sign language gestures using kinect 2.0. Lecture Notes in Computer Science (Including Subseries Lecture Notes in Artificial Intelligence and Lecture Notes in Bioinformatics), 2017, vol. 10278, pp. 89–101. https://doi.org/10.1007/978-3-319-58703-5_7
4. Ryumin D. Automated hand detection method for tasks of gesture recognition in human-machine interfaces. Scientific and Technical Journal of Information Technologies, Mechanics and Optics, 2020, vol. 20, no. 4, pp. 525–531. (in Russian). https://doi.org/10.17586/2226-1494-2020-20-4-525-531
5. Tomskaia M.V., Maslova L.N. Gender research in national linguistics. Russian language in the modern society: functional and status characteristics. Moscow, 2005, pp. 102–130. (in Russian)
6. Carli L., LaFleur S., Loeber C. Nonverbal behavior, gender, and influence. Journal of Personality and Social Psychology, 1995, vol. 68, no. 6, pp. 1030–1041. https://doi.org/10.1037/0022-3514.68.6.1030
7. Iriskhanova O., Cienki A. The semiotics of gestures in cognitive linguistics: Contribution and challenges. Voprosy Kognitivnoy Lingvistiki, 2018, vol. 4, pp. 25–36. https://doi.org/10.20916/1812-3228-2018-4-25-36
8. Masson-Carro I., Goudbeek M., Krahmer E. Coming of age in gesture: A comparative study of gesturing and pantomiming in older children and adults. Proc. of the 4th Gesture and Speech in Interaction Conference (GESPIN), 2015, pp. 1–7.
9. Reviewed Work: Sign language structure: An outline of the visual communication systems of the American deaf by William C. Stokoe, Jr. Language, 1961, vol. 37, no. 2, pp. 269–271. https://doi.org/10.2307/410856
10. Dimskis L.S. Learning Sign Language. Moscow, Akademija Publ., 2002, 128 p. (in Russian)
11. Sonkusare J., Chopade N., Sor R., Tade S. A review on hand gesture recognition system. Proc. of the 1st International Conference on Computing, Communication, Control and Automation, 2015, pp. 790–794. https://doi.org/10.1109/ICCUBEA.2015.158
12. De Smedt Q., Wannous H., Vandeborre J. Heterogeneous hand gesture recognition using 3D dynamic skeletal data. Computer Vision and Image Understanding, 2019, vol. 181, pp. 60–72. https://doi.org/10.1016/j.cviu.2019.01.008
13. Grif M., Prikhodko A., Bakaev M. Recognition of signs and movement epentheses in Russian Sign Language. Communications in Computer and Information Science, 2022, vol. 1503, pp. 67–82. https://doi.org/10.1007/978-3-030-93715-7_5
14. Grishina E.A. Ring and grappolo: Fingertip connections in Russian gesticulation and their meanings. Komp'juternaja Lingvistika i Intellektual'nye Tehnologii, 2014, no. 13, pp. 182–202. (in Russian)
15. Zhang C., Yang X., Tian Y. Histogram of 3D Facets: A characteristic descriptor for hand gesture recognition. Proc. of the 10th International Conference Automatic Face and Gesture Recognition (FG), 2013, pp. 6553754. https://doi.org/10.1109/FG.2013.6553754
16. Ryumin D.A., Kagirov I.A. Approaches to automatic gesture recognition: hardware and methods overview. Manned Spaceflight, 2021, no. 3(40), pp. 82–99. (in Russian). https://doi.org/10.34131/MSF.21.3.82-99
17. Camgoz C.N., Hadfield S., Koller O., Bowden R. SubUNets: End-to-end hand shape and continuous sign language recognition. Proc. of the 16th International Conference on Computer Vision (ICCV), 2017, pp. 3075–3084. https://doi.org/10.1109/ICCV.2017.332
18. Grif M.G., Korolkova O.O., Prikhodko A.L. Sign speech recognition taking into account combinatorial changes of gestures. Problems, Methods, and Technologies in the Computer Science. Proceedings of the XXI International Scientific and Technical Conference, 2021, pp. 1387–1393. (in Russian)
19. Ryumin D., Kagirov I., Axyonov A., Pavlyuk N., Saveliev A., Kipyatkova I., Zelezny M., Mporas I., Karpov A. A multimodal user interface for an assistive robotic shopping cart. Electronics, 2020, vol. 9, no. 12, pp. 1–25. https://doi.org/10.3390/electronics9122093
20. Axyonov А., Ryumin D., Kagirov I. Method of multi-modal video analysis of hand movements for automatic recognition of isolated signs of Russian sign language. International Archives of the Photogrammetry, Remote Sensing and Spatial Information Sciences, 2021, vol. 44, no. 2/W1, pp. 7–13. https://doi.org/10.5194/isprs-archives-XLIV-2-W1-2021-7-2021
21. Wu J., Zhang Y., Zhao X. A prototype-based generalized zero-shot learning framework for hand gesture recognition. Proc. of the 25th International Conference on Pattern Recognition (ICPR), 2021, pp. 3435–3442. https://doi.org/10.1109/ICPR48806.2021.9412548
22. Voskou A., Panousis K.P., Kosmopoulos D., Metaxas D.N., Chatzis S. Stochastic transformer networks with linear competing units: Application to end-to-end SL translation. Proc. of the 18th International Conference on Computer Vision (ICPR), 2021, pp. 11926–11935. https://doi.org/10.1109/ICCV48922.2021.01173
23. Jiang S., Sun B., Wang L., Bai Y., Li K., Fu Y. Skeleton aware multi-modal sign language recognition. Proc. of the Conference on Computer Vision and Pattern Recognition (CVPR), 2021, pp. 3408–3418. https://doi.org/10.1109/CVPRW53098.2021.00380
24. Ryumin D. Models and Methods for Automatic Recognition of Russian Sign Language Elements for Human-Machine Interaction. Academic dissertation сandidate of engineering. ITMO University, 2020, 352 p. Available at: http://fppo.ifmo.ru/dissertation/?number=246869(accessed: 26.03.2022). (in Russian)
25. Winata G.I., Kampman O.P., Fung P. Attention-based LSTM for psychological stress detection from spoken language using distant supervision. Proc. of the International Conference on Acoustics, Speech and Signal Processing (ICASSP), 2018, pp. 6204–6208. https://doi.org/10.1109/ICASSP.2018.8461990
26. Serengil S.I., Ozpinar A. LightFace: A Hybrid deep face recognition framework. Proc. of the Innovations in Intelligent Systems and Applications Conference (ASYU), 2020, pp. 9259802. https://doi.org/10.1109/ASYU50717.2020.9259802
27. Zhang H., Cisse M., Dauphin Y.N., Lopez-Paz D. MixUp: Beyond empirical risk minimization. Proc. of the 6th International Conference on Learning Representations (ICLR), 2018.
28. Dresvyanskiy D., Ryumina E., Kaya H., Markitantov M., Karpov A., Minker W. End-to-end modeling and transfer learning for audiovisual emotion recognition in-the-wild. Multimodal Technologies and Interaction, 2022, vol. 6, no. 2, pp. 11. https://doi.org/10.3390/mti6020011
29. Zhong Z., Lin Z.Q., Bidart R., Hu X., Daya I.B., Li Z., Zheng W., Li J., Wong A. Squeeze-and-attention networks for semantic segmentation. Proc. of the Conference on Computer Vision and Pattern Recognition (CVPR), 2020, pp. 13062–13071. https://doi.org/10.1109/cvpr42600.2020.01308
30. Kagirov I., Ivanko D., Ryumin D., Axyonov A., Karpov A. TheRuSLan: Database of Russian Sign Language. Proc. of the 12th Conference on Language Resources and Evaluation (LREC), 2020, pp. 6079–6085.
31. Kagirov I.A., Ryumin D.A., Axyonov A.A., Karpov A.A. Multimedia database of russian sign language items in 3D. Voprosy Jazykoznanija, 2020, no. 1, pp. 104–123. (in Russian). https://doi.org/10.31857/S0373658X0008302-1