Menu
Publications
2025
2024
2023
2022
2021
2020
2019
2018
2017
2016
2015
2014
2013
2012
2011
2010
2009
2008
2007
2006
2005
2004
2003
2002
2001
Editor-in-Chief
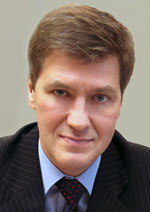
Nikiforov
Vladimir O.
D.Sc., Prof.
Partners
doi: 10.17586/2226-1494-2022-22-3-594-599
Web app for quick evaluation of subjective answers using natural language processing
Read the full article

Article in English
For citation:
Abstract
For citation:
Meenakshi Anurag T., Pradeep B.M., Vishaka M. Web app for quick evaluation of subjective answers using natural language processing. Scientific and Technical Journal of Information Technologies, Mechanics and Optics, 2022, vol. 22, no. 3, pp. 594–599. doi: 10.17586/2226-1494-2022-22-3-594-599
Abstract
In current digital climate, education sector is evolving as the computer technology advances. Education is being digitized: online classes, online examination methods are conducted, etc. During examination, students are assessed by their answers having given for the question set by a teacher. Today many tools are available to assess the performance of a student using multi choice questions tools which provide instant evaluation, but there are available very limited and operational tools where subjective type answer of students are evaluated. This paper presents a web-based application to address this challenge. It automates the process of subjective answers checking and generates results through using natural language processing methods, like keyword matching semantic, lexical analysis and cosine similarity. Experiments show that appreciated by the teacher result and the system estimation does not have much difference which signifies that the system evaluates answers with a 97 % accuracy. The presented system not only reduces manpower but also eliminates the traditional method of conducting exclusively subjective exams using paper documents. It also eliminates the delays in the paper checking, result generation process. The cases of information leak are being reduced and the objectivity of the assessment is being increased.
Keywords: cosine similarity, information extraction, keyword matching, natural language processing
References
References
-
Lakshmi V., Ramesh V. Evaluating students’ descriptive answers using natural language processing and artificial neural networks. International Journal of Creative Research Thoughts – IJCRT, 2017, vol. 5, no. 4, pp. 3168–3173.
-
Patil S.M., Patil S. Evaluating student descriptive answers using natural language processing. International Journal of Engineering Research & Technology (IJERT), 2014, vol. 3, no. 3, pp. 1716–1718.
-
Yin W., Kann K., Yu M., Schütze H. Comparative study of CNN and RNN for natural language processing. ArXiv, 2017, arXiv:1702.01923. https://doi.org/10.48550/arXiv.1702.01923
-
Kate A., Kamble S., Bodkhe A., Joshi M. Conversion of Natural Language Query to SQL Query. Proc. of the 2nd International conference on Electronics, Communication and Aerospace Technology (ICECA), 2018, pp. 488–491. https://doi.org/10.1109/ICECA.2018.8474639
-
Thangarasu S., Sasikala D. Extracting knowledge from XML document using tree-based association rules. Proc. of the International Conference on Intelligent Computing Applications (ICICA), 2014, pp. 134–137. https://doi.org/10.1109/ICICA.2014.37
-
Mati D.N., Ajdari J., Raufi B., Hamiti M., Selimi B. A Systematic mapping study of language features identification from large text collection. Proc. of the 8th Mediterranean Conference on Embedded Computing (MECO), 2019, pp. 8760042. https://doi.org/10.1109/MECO.2019.8760042
-
Cai J., Li J., Li W., Wang J. Deeplearning model used in text classification. Proc of the 15th International Computer Conference on Wavelet Active Media Technology and Information Processing (ICCWAMTIP), 2018, pp. 123–126. https://doi.org/10.1109/ICCWAMTIP.2018.8632592
-
Tan M., Santos C., Xiang B., Zhou B. Improved representation learning for question answer matching. Proc. of the 54th Annual Meeting of the Association for Computational Linguistics (ACL), 2016, pp. 464–473. https://doi.org/10.18653/v1/P16-1044
-
Lan Y., Wang S., Jiang J. Knowledge base question answering with a matching-aggregation model and question-specific contextual relations. IEEE/ACM Transactions on Audio, Speech, and Language Processing, 2019, vol. 27, no. 10, pp. 1629–1638. https://doi.org/10.1109/TASLP.2019.2926125
-
Grappy A., Grau B., Falco M-H., Ligozat A-L., Robba I., Vilnat A. Selecting answers to questions from Web documents by a robust validation process. Proc. of the IEEE/WIC/ACM International Conferences on Web Intelligence and Intelligent Agent Technology, 2011, pp. 55–62. https://doi.org/10.1109/WI-IAT.2011.210
-
Lan Y., Wang S., Jiang J. Knowledge base question answering with a matching-aggregation model and question specific contextual relations. IEEE/ACM Transactions on Audio, Speech, and Language Processing, 2019, vol. 27, no. 10, pp. 1629–1638. https://doi.org/10.1109/TASLP.2019.2926125
-
Zhang W., Ming Z., Zhang Y., Liu T., Chua T. Capturing the semantics of key phrases using multiple languages for question retrieval. IEEE Transactions on Knowledge and Data Engineering, 2016, vol. 28, no. 4, pp. 888–900. https://doi.org/10.1109/TKDE.2015.2502944
-
Pakray P., Pal S., Bandyopadhyay S., Gelbukh A. Automatic answer validation system on English language. Proc. of the 3rd International Conference on Advanced Computer Theory and Engineering, 2010, pp. V6329–V6333. https://doi.org/10.1109/ICACTE.2010.5579166
-
Thalor M.A. A descriptive answer evaluation system using cosine similarity technique. Proc. of the 4th International Conference on Communication information and Computing Technology (ICCICT), 2021. https://doi.org/10.1109/ICCICT50803.2021.9510170
-
Mandge V.A., Thalor M.A. Revolutionize cosine answer matching technique for question answering system. Proc. of the International Conference on Emerging Smart Computing and Informatics (ESCI), 2021, pp. 335–339. https://doi.org/10.1109/ESCI50559.2021.9396864