Menu
Publications
2025
2024
2023
2022
2021
2020
2019
2018
2017
2016
2015
2014
2013
2012
2011
2010
2009
2008
2007
2006
2005
2004
2003
2002
2001
Editor-in-Chief
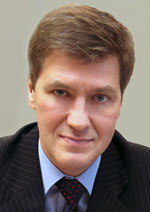
Nikiforov
Vladimir O.
D.Sc., Prof.
Partners
doi: 10.17586/2226-1494-2022-22-3-610-622
Monitoring of infiltration processes in hydraulic structures using distributed acoustic sensing technology
Read the full article

Article in English
For citation:
Abstract
For citation:
Timofeev A.V., Groznov D.I. Monitoring of infiltration processes in hydraulic structures using distributed acoustic sensing technology. Scientific and Technical Journal of Information Technologies, Mechanics and Optics, 2022, vol. 22, no. 3, pp. 610–622. doi: 10.17586/2226-1494-2022-22-3-610-622
Abstract
The article proposes a new method of monitoring the infiltration processes developing inside the body of hydraulic structures. The method is based on the use of DAS (distributed acoustic sensing) fiber-optic technology which provides high spatial continuity of hydraulic structure seismoacoustic field analysis; digital twin infiltration dynamics and efficient signal processing methods based on machine learning. As a distributed sensor of the object’s seismoacoustic field, a DAS system is used the fiber-optic sensor of which is installed inside the body of the structure according to the principle of maximum coverage. The infiltration activity inside the structure body is estimated based on the analysis of an infiltration flows ensemble which are detected and classified by machine learning (ML) methods. These infiltration flows are sources of seismoacoustic emission and are therefore confidently detected by the DAS system. A digital twin of the infiltration dynamics based on the equations of mathematical physics is used as the normal basis for estimating the current state of fluid activity in the body of the structure. The risk of a structure failure under the influence of the observed infiltration flow is estimated within the framework of the proposed formal method based on the digital twin data. Based on the analysis of Data Set, consisting of real signals of infiltration processes, the high efficiency of detection and classification of this type of signals with the special ML-classifier included in the monitoring system is proved. A digital twin model of the infiltration processes dynamics in the body of a hydraulic structure is proposed. On the basis of the digital twin model, a method for estimating the risk of damage to the body of a hydraulic structure, which may occur as a result of the observed infiltration activity, is proposed. The method of controlling infiltration processes inside hydraulic structures can be used to monitor the operational condition of almost any hydraulic structures, including those in the cryolithic zone.
Keywords: DAS, infiltration process, machine learning, classification, hydraulic structures, tailings monitoring, dam monitoring, SVM, digital twin, cryolithozone, Darcy’s equation
References
References
-
Sukhno A.M., Khlapov N.N. Automatic system for monitoring at the tailing storage of Aikhal'sky mining and concentrating works. Gornyi Zhurnal, 2008, no. 5, pp. 65–68. (in Russian).
-
Besimbaeva O.G., Khmyrova E.N., Loginov A.V., Oleinikova E.A. Research of the opportunities of 3D modeling for mine surveying support of mining works. Interexpo GEO-Siberia, 2017, vol. 1, no. 1, pp. 125–129. (in Russian).
-
Aravin V., Numerov S. The Theory of Motion of Liquids and Gases in Non-Deformable Porous Medium. Moscow: Gosudarstvennoe izdatel'stvo tehniko-teoreticheskoj literatury, 1953, 616 с. (in Russian).
-
Timofeev A.V., Groznov D.I. Classification of seismoacoustic emission sources in fiber optic systems for monitoring extended objects. Optoelectronics, Instrumentation and Data Processing, 2020, vol. 56,no. 1, pp. 50–60. https://doi.org/10.3103/S8756699020010070
-
Timofeev A.V., Denisov V.M. Multimodal heterogeneous monitoring of super-extended objects: Modern view. Studies in Systems, Decision and Control, 2016, vol. 62, pp. 97–116. https://doi.org/10.1007/978-3-319-32525-5_6
-
Becker M., Coleman T., Ciervo C., Cole M., Mondanos M. Fluid pressure sensing with fiber-optic distributed acoustic sensing. Leading Edge, 2017, vol. 36, no. 12, pp. 1018–1023. https://doi.org/10.1190/tle36121018.1
-
Liu X., Jin B., Bai Q., Wang Y., Wang D., Wang Y. Distributed fiber-optic sensors for vibration detection. Sensors, 2016, vol. 16, no. 8, pp. 1164. https://doi.org/10.3390/s16081164
-
Wang Y., Jin B., Wang Y., Wang D., Liu X., Bai Q. Real-time distributed vibration monitoring system using Φ –OTDR. IEEE Sensors Journal, 2017, vol. 17, no. 5, pp. 1333–1341. https://doi.org/10.1109/jsen.2016.2642221
-
Inaudi D., Cottone I., Figini A. Monitoring dams and levees with distributed fiber optic sensing. Proc. of the 6th International Conference on Structural Health Monitoring of Intelligent Infrastructure, 2013.
-
Johansson S., Beaupretre S., Boue A., Stork A. Distributed Acoustic Sensing for Detection of Defects in the Test Dam at Älvkarleby. Technical Report. 2021. Available at: https://energiforsk.se/en/programmes/dam-safety/reports/distributed-acoustic-sensing-for-detection-of-defects-2021-732/(accessed: 15.12.2021).
-
Ouellet S., Suszek N., Lato M., Russell B., Froese C., Carey E. Static liquefaction-type tailings dam failures: understanding options for detecting failures. Proc. of Tailings and Mine Waste, November 17–20 2019. Vancouver, Canada. University of British Columbia, 2019, pp. 415–420.
-
Forbes B., Ouellet S., Suszek N., Lato M., Russell B. Application of distributed acoustic sensing within a tailings dam warning system. Proc. of Tailings and Mine Waste Conference, 2021.
-
Rasskazov L.N., Orekhov V.G., Aniskin N.A. Hydrotechnical Constructions. Part 1. Textbook. Moscow: Association of Civil Engineering Universities Publ., 2008. 575 p. (in Russian).
-
Shapiro N., Campillo M., Stehly L., Ritzwoller M. High-resolution surface-wave tomography from ambient seismic noise. Science, 2005, vol. 307, no. 5715, pp. 1615–1618. https://doi.org/10.1126/science.110833
-
Cristianini N., Shawe-Taylor J. An Introduction to Support Vector Machines and Other Kernel-based Learning Methods. Cambridge University Press, 2000, 189 p.